AirDrop: Towards Collaborative, Multi-Resolution Air-Ground Teaming for Terrain-Aware Navigation.
HotMobile(2023)
摘要
Driven by advances in deep neural network models that fuse multi-modal input such as RGB and depth representations to accurately understand the semantics of the environments (e.g., objects of different classes, obstacles, etc.), ground robots have gone through dramatic improvements in navigating unknown environments. Relying on their singular, limited perspective, however, can lead to suboptimal paths that are wasteful and quickly drain out their batteries, especially in the case of long-horizon navigation. We consider a special class of ground robots, that are air-deployed, and pose the central question: can we leverage aerial perspectives of differing resolutions and fields of view from air-to-ground robots to achieve superior terrain-aware navigation? We posit that a key enabler of this direction of research is collaboration between such robots, to collectively update their route plans, leveraging advances in long-range communication and on-board computing. Whilst each robot can capture a sequence of high resolution images during their descent, intelligent, lightweight pre-processing on-board can dramatically reduce the size of the data that needs to be shared among its peers over severely bandwidth-limited long range communication channels (e.g., over sub gigahertz frequencies). In this paper, we discuss use cases and key technical challenges that must be resolved to realize our vision for collaborative, multi-resolution terrain-awareness for air-to-ground robots.
更多查看译文
AI 理解论文
溯源树
样例
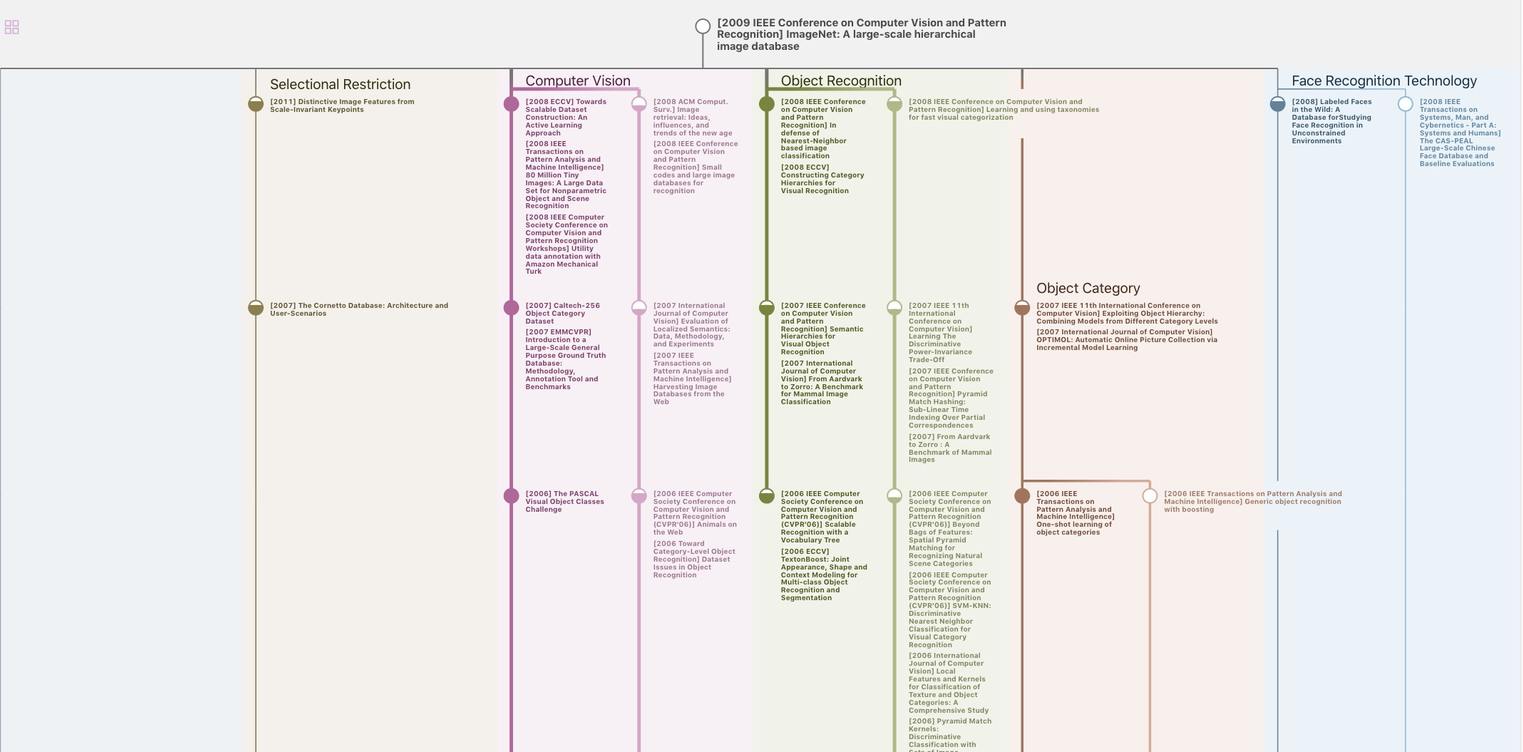
生成溯源树,研究论文发展脉络
Chat Paper
正在生成论文摘要