SODA: Protecting Proprietary Information in On-Device Machine Learning Models
HotMobile(2023)
摘要
The growth of low-end hardware has led to a proliferation of machine
learning-based services in edge applications. These applications gather
contextual information about users and provide some services, such as
personalized offers, through a machine learning (ML) model. A growing practice
has been to deploy such ML models on the user's device to reduce latency,
maintain user privacy, and minimize continuous reliance on a centralized
source. However, deploying ML models on the user's edge device can leak
proprietary information about the service provider. In this work, we
investigate on-device ML models that are used to provide mobile services and
demonstrate how simple attacks can leak proprietary information of the service
provider. We show that different adversaries can easily exploit such models to
maximize their profit and accomplish content theft. Motivated by the need to
thwart such attacks, we present an end-to-end framework, SODA, for deploying
and serving on edge devices while defending against adversarial usage. Our
results demonstrate that SODA can detect adversarial usage with 89
less than 50 queries with minimal impact on service performance, latency, and
storage.
更多查看译文
关键词
on-device,machine learning,proprietary information,privacy
AI 理解论文
溯源树
样例
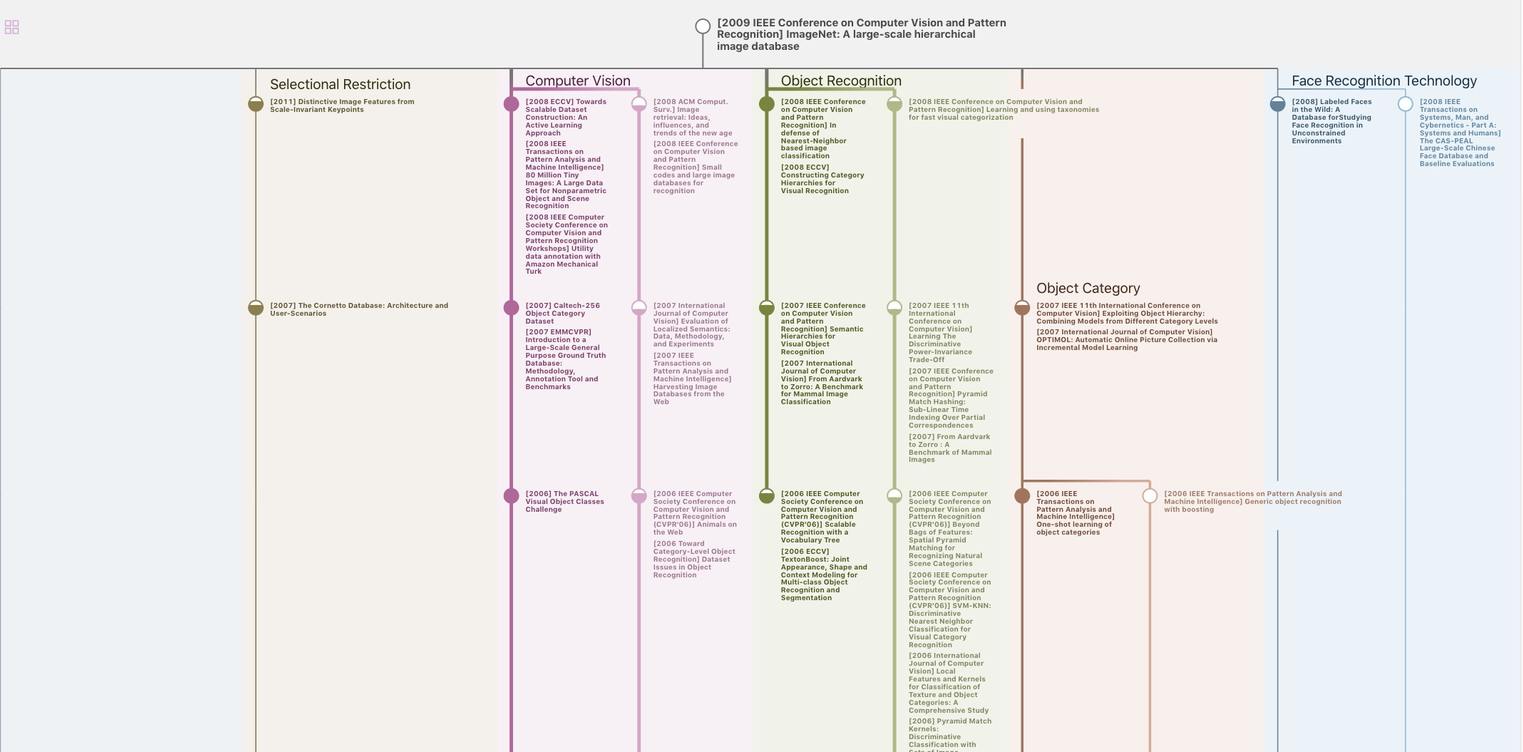
生成溯源树,研究论文发展脉络
Chat Paper
正在生成论文摘要