Estimation of User Personality Traits on the Web Using Multi-Task Learning.
HotMobile(2023)
摘要
In recent web services, it is possible to perform more efficient marketing strategies and recommendations than ever before by considering users' psychological states, such as emotions and personality traits. In particular, personality traits are stable over the long term and thus have a greater impact on human behavior and decision-making than short-term fluctuating traits such as emotions and stress. Diverse applications can be expected by making it possible to estimate the users' personality traits on mobile devices: recommendation, service personalization, job screenings, and social network analysis. However, it is impractical to obtain responses from all users of the service, as questionnaires are commonly used to assess personality traits. In this study, we propose a novel method to estimate users' personality traits automatically from web search behavior and browsing behavior of web news articles. The proposed method is shown in Figure 1. We constructed deep multi-task learning model from 8728 user data through Yahoo! JAPAN service, using the features calculated from news browsing logs and search queries. As ground truth, we used Big Five (Extraversion, Agreeableness, Conscientiousness, Neuroticism, and Openness) collected via the Ten Item Personality Inventory [2] using crowdsourcing. From the article ID of news browsing logs, we calculated embedding features using Doc2Vec (natural language processing technique) that considers a user as a document and viewed article IDs as words. In addition, we extracted features that took the user context states into account. For example, the time and rate of article reading were aggregated for each user, and multiple statistical processes were performed. From search queries, we extracted psycho-linguistic features using J-LIWC lexicon [1]. A multi-task neural network consists of an input layer, three hidden layers, and five output layers in Figure 2. The number of nodes in the input layer is 202, with 200 and 100 nodes in the shared hidden layer, and 50 nodes in the task-specific hidden layer, through which 2-dimensional values are finally output for binary classification. We use Swish activation in the first three layers, Identity activation in the task-specific layer, and Sigmoid activation in the final layer. All data were randomly split at a ratio of 8:2, with 80% of samples as training data and 20% of samples as test data. For training our model, we trained for 50 epochs, the batch size set to 32, used AdamW as the optimizer, learning rate was 1e-5. We calculated the accuracy for each Big Five item in the test data and obtained the following results: Extraversion: 0.58, Agreeableness: 0.57, Conscientiousness: 0.48, Neuroticism: 0.50, Openness: 0.54. As a result, we confirmed that personality traits can be estimated with a certain degree of accuracy for several items.
更多查看译文
AI 理解论文
溯源树
样例
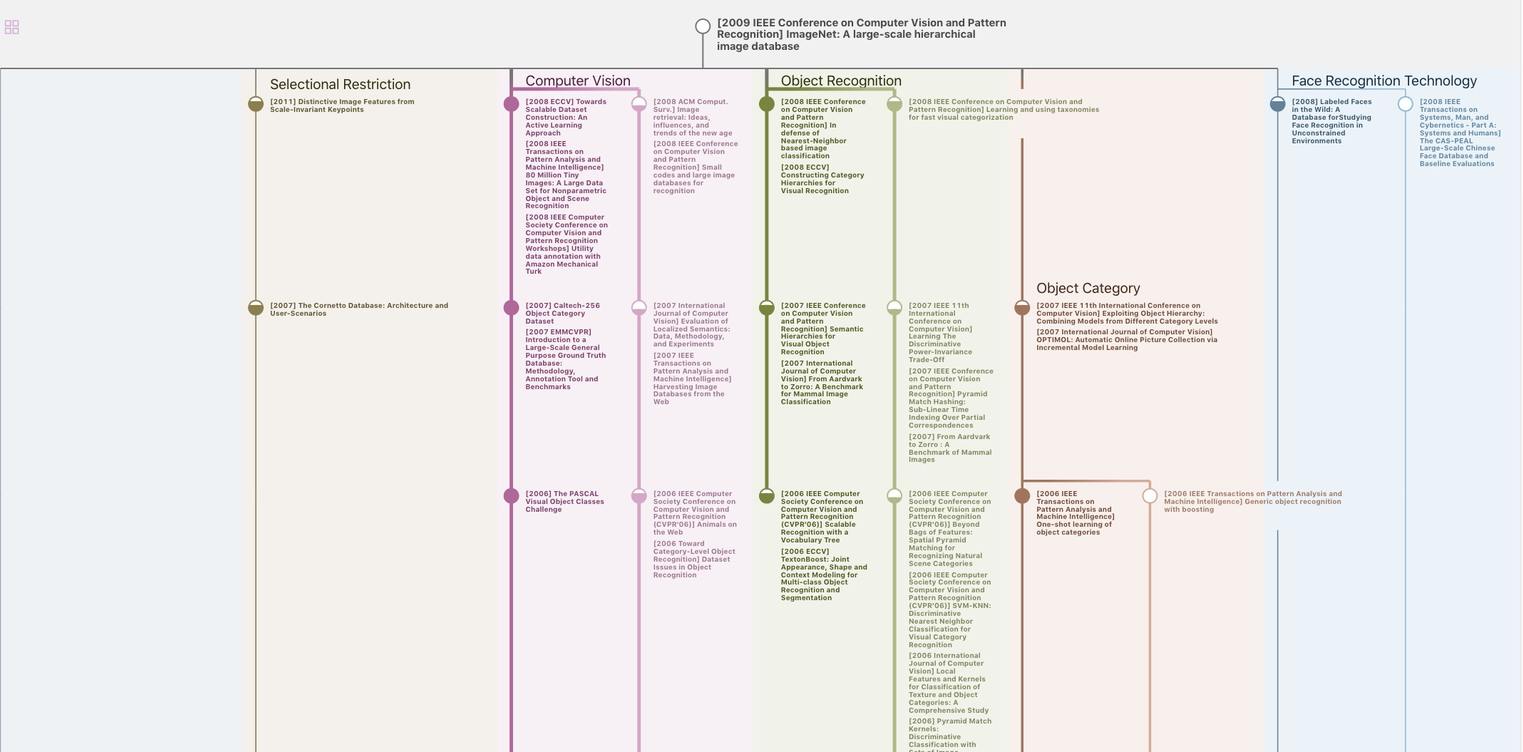
生成溯源树,研究论文发展脉络
Chat Paper
正在生成论文摘要