Decoupling Schedule, Topology Layout, and Algorithm to Easily Enlarge the Tuning Space of GPU Graph Processing.
PACT(2022)
摘要
Only with a right schedule and a right topology layout, a graph algorithm can be efficiently processed on GPUs. Existing GPU graph processing frameworks try to find an optimal schedule and topology layout for an algorithm via iterative search, but they fail to find the optimal configuration because their schedules and topology layouts are tightly coupled in their processing models. Moreover, their tightly coupled schedules and topology layouts make it difficult for developers to extend the tuning space. To easily enlarge the tuning space of GPU graph processing, this work proposes a new GPU graph processing abstraction scheme that fully decouples schedules, topology layouts, and algorithms from each other with abstraction interfaces. Moreover, this work proposes GRAssembler, a new GPU graph processing framework that efficiently integrates the decoupled schedule, topology layout, and algorithm without abstraction overhead. Thanks to the efficient decoupling and integration, GRAssembler increases the tuning space from 336 to 4,480 and achieves 30.4% higher performance on geomean average, compared to the state-of-the-art GPU graph processing framework.
更多查看译文
关键词
Graph Processing, Compiler Optimization, GPUs, Auto-tuning
AI 理解论文
溯源树
样例
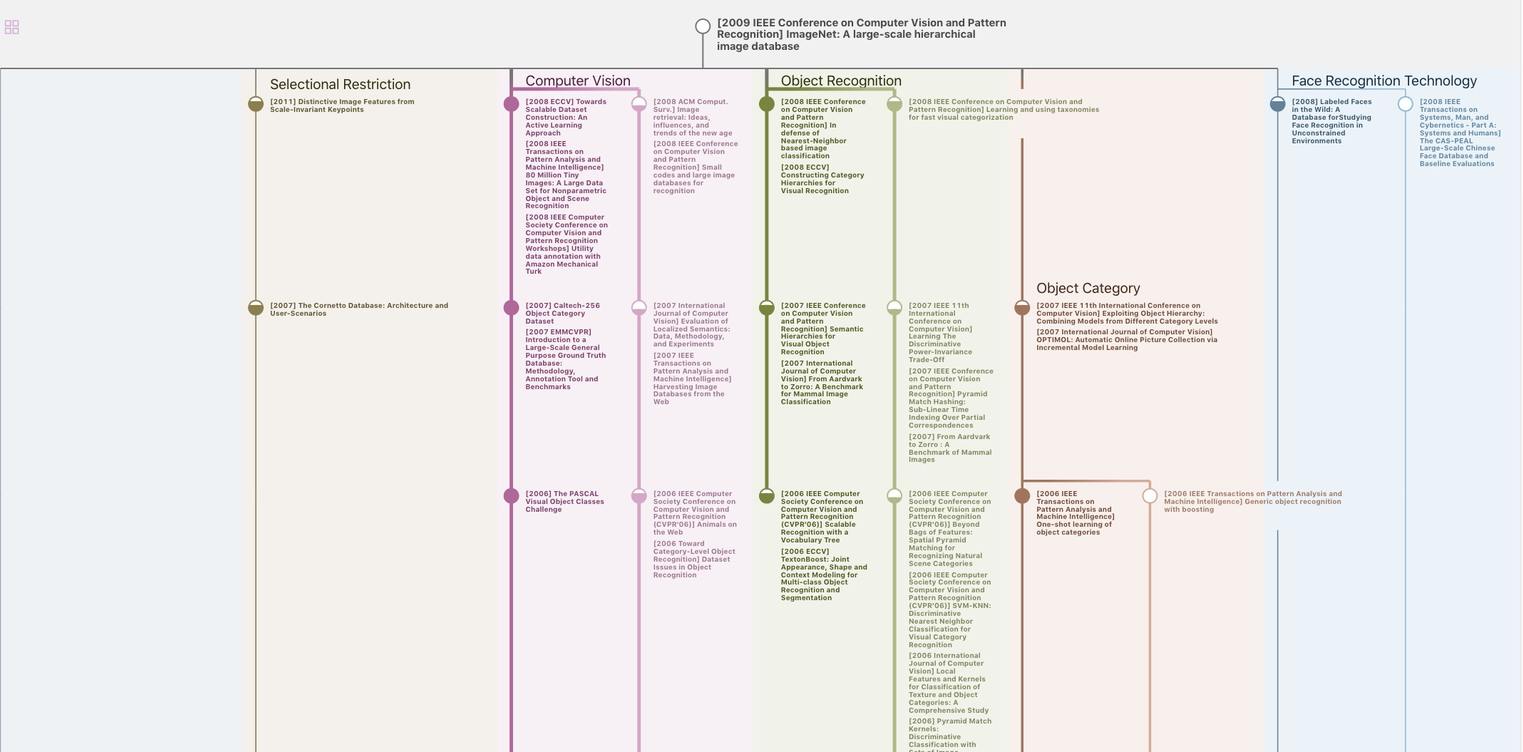
生成溯源树,研究论文发展脉络
Chat Paper
正在生成论文摘要