Modeling dynamics of cryptocurrency XRP using tweets, news, and GMDH-based algorithms.
CSIT(2022)
摘要
This paper shows the possibilities of an averaged quantitative forecast of popular cryptocurrency XRP based on dynamics of tweets and news. The contribution of the paper are threefold: 1) mid-term forecasts (weekly and monthly ones) with typical GMDH-based algorithms unlike forecasts with other algorithms; 2) consideration of news (experts) along with tweets (population) unlike traditional attention to tweets; 3) using only contents of texts reflected in tweets and news instead of their tonality and other related characteristics. The paper describes procedures of text preprocessing and modeling. Experiments are implemented with regression and hybrid models. The data cover the period of a full year (2020). Baselines are presented by time series analysis with GMDH-based algorithms and the popular Holt-Winter (HW) method. To reveal the temporal relations between tweets and news we propose to use two-way mutual correlation. The completed research shows the following results: 1) application of tweets provides better accuracy than that of news both for month and for week forecasts; 2) GMDH-based algorithms with autoregression have a small advantages over HW-method for month and for week forecasts; 3) using tweets and news for month forecast gives the results close to those of Baseline for the best models and algorithms; 4) using tweets and news for week forecast has significant advantage comparing to Baseline, when Neuro algorithm is used on hybrid model; 5) news get ahead of tweets in their prediction of XRP dynamics but the proposed way needs an additional study.
更多查看译文
关键词
XRP, ripple, cryptocurrency, forecast, text mining, mutual correlation, GMDH, tweets, news
AI 理解论文
溯源树
样例
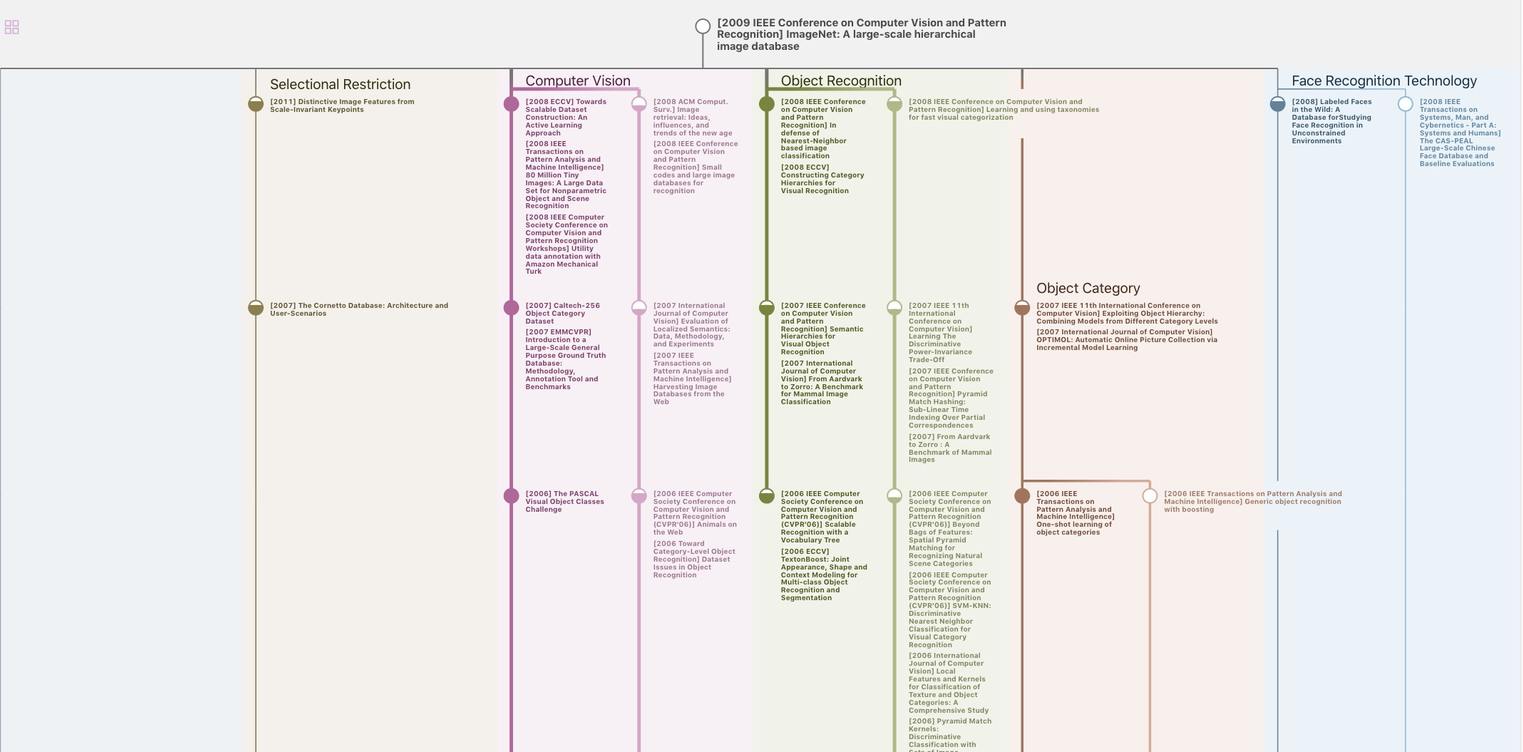
生成溯源树,研究论文发展脉络
Chat Paper
正在生成论文摘要