Deeblif: Deep Blind Light Field Image Quality Assessment by Extracting Angular and Spatial Information.
ICIP(2022)
摘要
In the era of immersive media, the high-dimensional Light Field Image (LFI) puts forward higher requirements for Light Field Image Quality Assessment (LF-IQA). However, currently most existing LF-IQA metrics still rely on sophisticated hand-crafted feature extraction, which fail to predict the quality of LFI accurately. In this paper, we propose a patch-based Deep Blind Light Field image quality assessment metric (abbreviated as DeeBLiF), by employing a two-stream Convolutional Neural Network (CNN) model specifically designed for extracting the angular and spatial information of LFI. Firstly, the spatio-angular patches are generated as input data, which effectively reflect the spatio-angular information of LFI. After that, a two-stream CNN model is exploited to extract the patch features and further predict the patch scores. Finally, all the patch scores are pooled into an overall quality score of LFI. Experimental results on the LFI dataset demonstrate that the proposed DeeBLiF outperforms the state-of-the-art LF-IQA metrics. The code will be publicly available at https://github.com/ZhengyuZhang96/DeeBLiF.
更多查看译文
关键词
Immersive media, light field, blind image quality assessment, spatio-angular patch, CNN
AI 理解论文
溯源树
样例
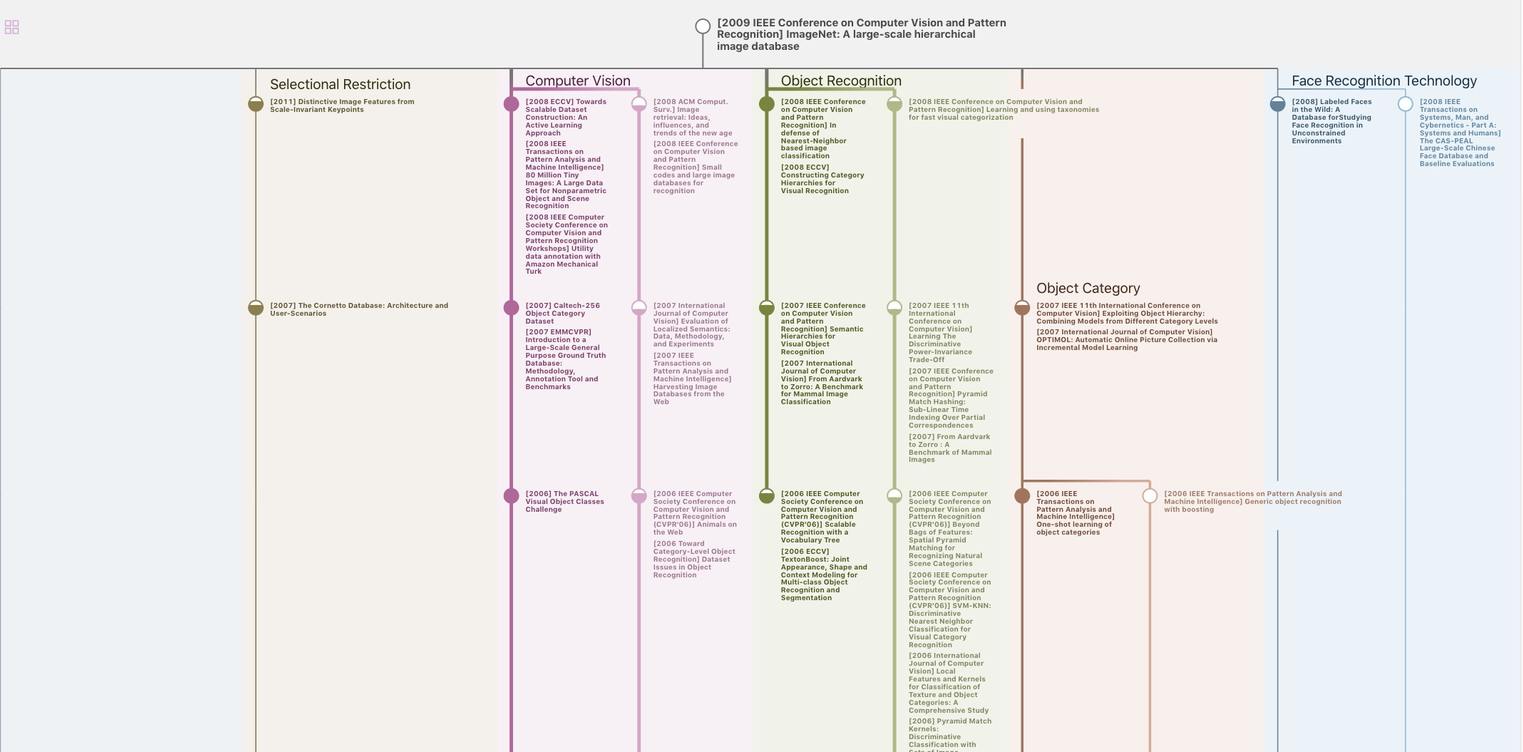
生成溯源树,研究论文发展脉络
Chat Paper
正在生成论文摘要