When is the Cleaning of Subjective Data Relevant to Train UGC Video Quality Metrics?
ICIP(2022)
摘要
Outlier analysis and spammer detection recently gained momentum in order to reduce uncertainty of subjective ratings in image & video quality assessment tasks. The large proportion of unreliable ratings from online crowdsourcing experiments and the need for qualitative and quantitative large-scale studies in the deep-learning ecosystem played a role in this event. We study the effect that data cleaning has on trainable models predicting the visual quality for videos, and present results demonstrating when cleaning is necessary to reach higher efficiency. To this end, we present and analyze a benchmark on clean and noisy User Generated Content (UGC) large-scale datasets on which we re-trained models, followed by an empirical exploration of the constraint of data removal. Our results show that a dataset presenting between 7 and 30% of outliers benefits from cleaning before training.
更多查看译文
关键词
Outlier, Cleaning, Video Quality Metrics, User Generated Content, Training metrics
AI 理解论文
溯源树
样例
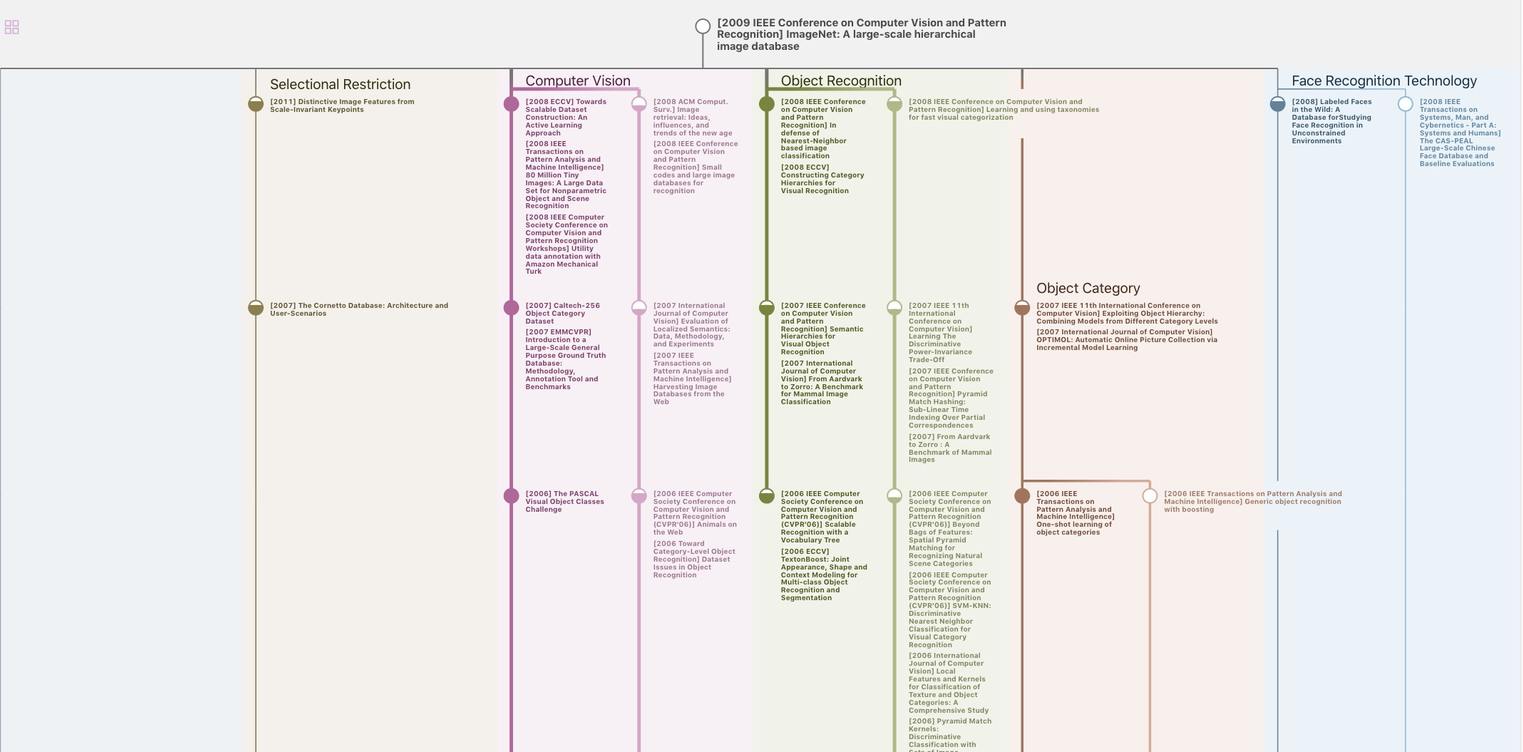
生成溯源树,研究论文发展脉络
Chat Paper
正在生成论文摘要