Boosting the Performance of Weakly-Supervised 3d Human Pose Estimators With Pose Prior Regularizers.
ICIP(2022)
摘要
This work aims to boost the performance of 3D human pose estimators trained in a weakly-supervised setting where there are much fewer annotated 3D poses than unlabeled video data. We formulate two self-supervised pose prior regularizers (PPR) - bone proportion and joint mobility constraints - that are pose translation, scale, and rotation invariant. These regularizers, combined with bone symmetry loss, reduce overfitting to the 2D reprojection loss commonly used in weakly-supervised settings by optimizing the bone lengths and joint rotations of estimated 3D poses. Consequently, improving the accuracy of 3D pose estimators. The regularizers are network independent and can be applied to any network architecture without modifications. We apply our proposed PPR to VideoPose3D network [1] and show that it decreases the MPJPE by 24% when using <= 5% of annotated H36M [2] 3D data, improving state-of-the-art accuracy by 7.9 mm.
更多查看译文
关键词
pose estimation,weak supervision,pose prior regularizers,bone proportion,joint mobility constraint
AI 理解论文
溯源树
样例
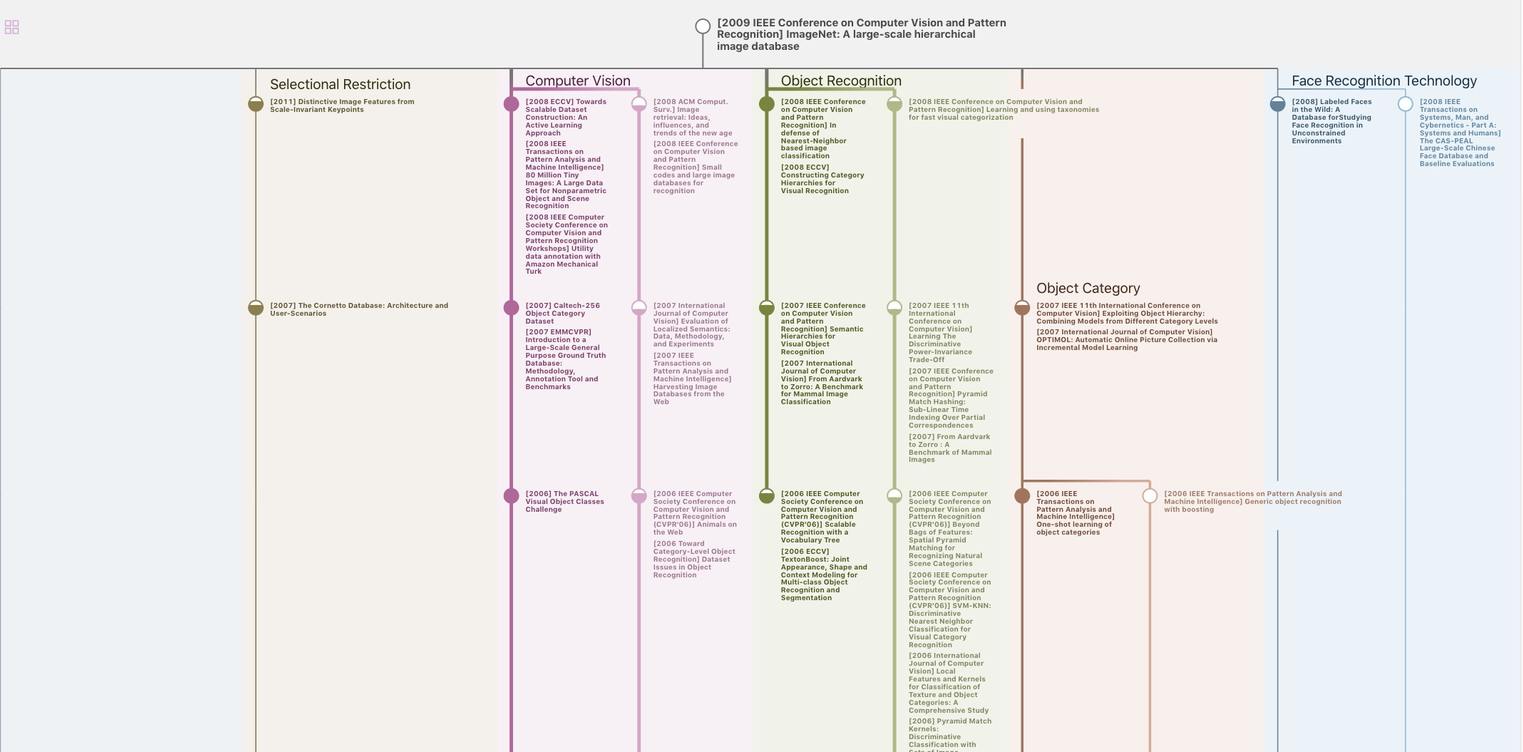
生成溯源树,研究论文发展脉络
Chat Paper
正在生成论文摘要