Predicting Radiologist Attention During Mammogram Reading with Deep and Shallow High-Resolution Encoding.
ICIP(2022)
摘要
Radiologists' eye-movement during diagnostic image reading reflects their personal training and experience, which means that their diagnostic decisions are related to their perceptual processes. For training, monitoring, and performance evaluation of radiologists, it would be beneficial to be able to automatically predict the spatial distribution of the radiologist's visual attention on the diagnostic images. The measurement of visual saliency is a well-studied area that allows for prediction of a person's gaze attention. However, compared with the extensively studied natural image visual saliency (in free viewing tasks), the saliency for diagnostic images is less studied; there could be fundamental differences in eye-movement behaviours between these two domains. Most current saliency prediction models have been optimally developed for natural images, which could lead them to be less adept at predicting the visual attention of radiologists during the diagnosis. In this paper, we propose a method specifically for automatically capturing the visual attention of radiologists during mammogram reading. By adopting high-resolution image representations from both deep and shallow encoders, the proposed method avoids potential detail losses and achieves superior results on multiple evaluation metrics in a large mammogram eye-movement dataset.
更多查看译文
关键词
Eye movement, saliency, radiologist, mammogram, deep learning
AI 理解论文
溯源树
样例
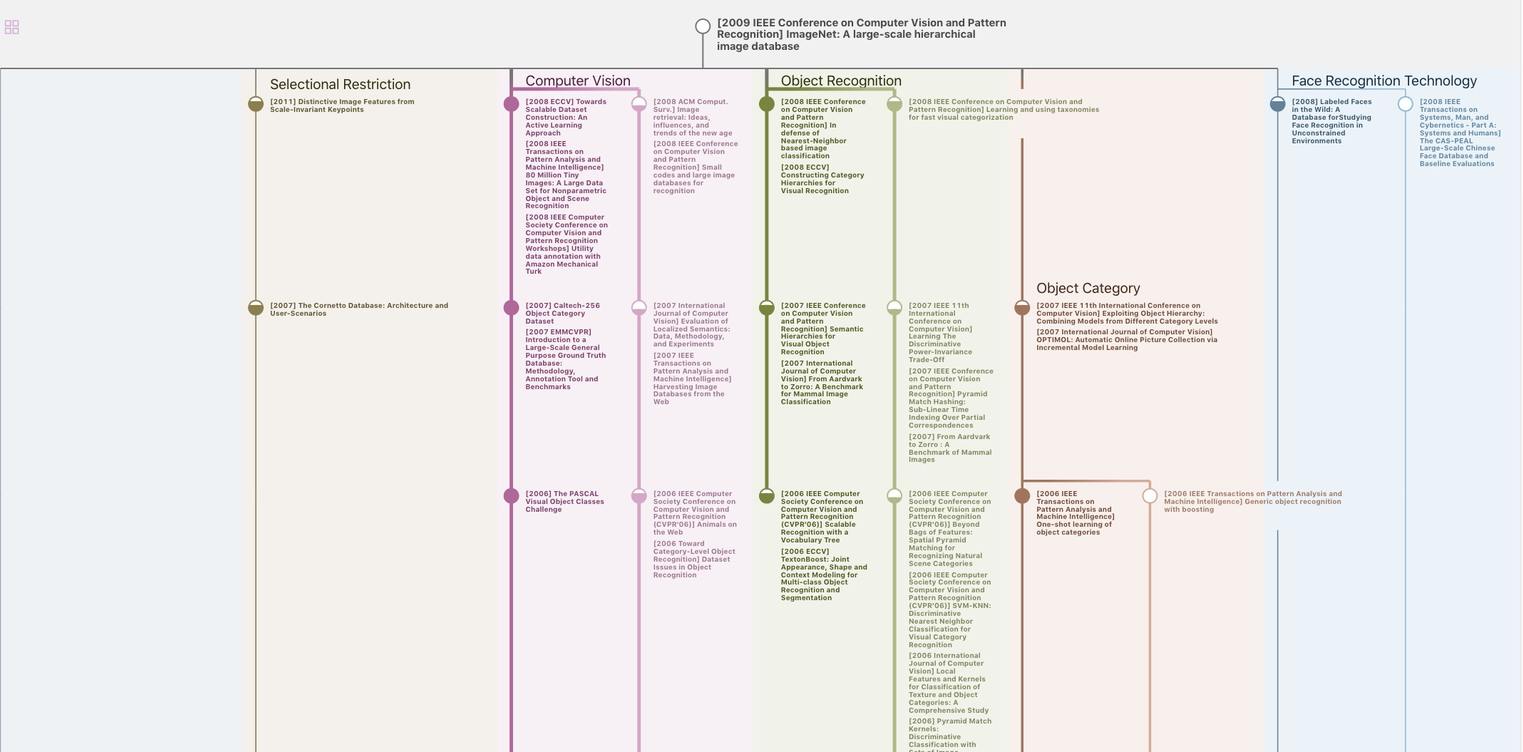
生成溯源树,研究论文发展脉络
Chat Paper
正在生成论文摘要