Adversarial Label-Poisoning Attacks and Defense for General Multi-Class Models Based on Synthetic Reduced Nearest Neighbor.
ICIP(2022)
摘要
Machine learning models are vulnerable to data poisoning attacks whose purpose is to undermine the model's integrity. However, the current literature on data poisoning attacks mainly focuses on ad hoc techniques that are generally limited to either binary classifiers or to gradient-based algorithms. To address these limitations, we propose a novel model-free label-flipping attack based on the multi-modality of the data, in which the adversary targets the clusters of classes while constrained by a label-flipping budget. The complexity of our proposed attack algorithm is linear in time over the size of the dataset. Also, the proposed attack can increase the error up to two times for the same attack budget. Second, a novel defense technique is proposed based on the Synthetic Reduced Nearest Neighbor model. The defense technique can detect and exclude flipped samples on the fly during the training procedure. Our empirical analysis demonstrates that (i) the proposed attack technique can deteriorate the accuracy of several models drastically, and (ii) under the proposed attack, the proposed defense technique significantly outperforms other conventional machine learning models in recovering the accuracy of the targeted model.
更多查看译文
关键词
Synthetic Reduced Nearest Neighbor,Adversarial Attacks,Modality,Machine Learning
AI 理解论文
溯源树
样例
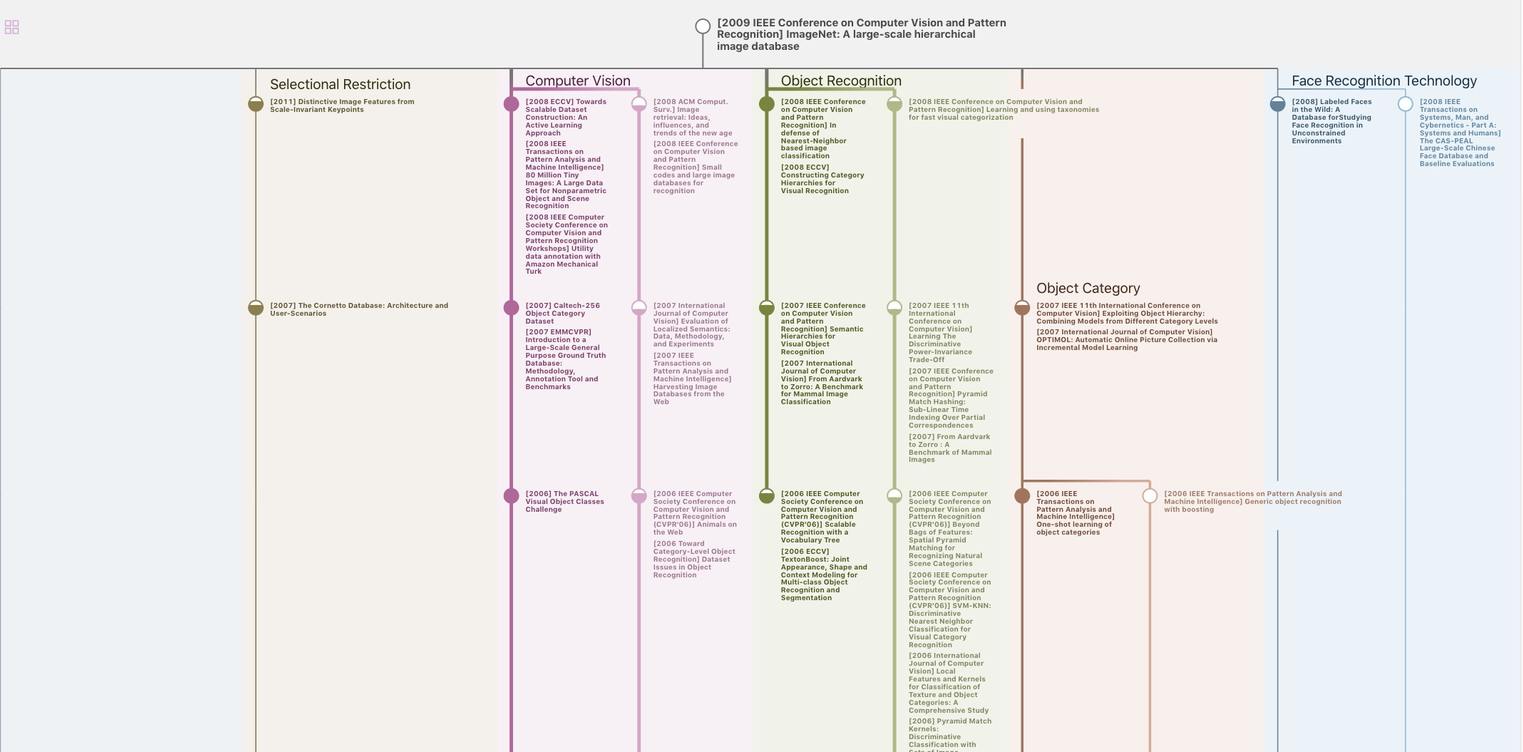
生成溯源树,研究论文发展脉络
Chat Paper
正在生成论文摘要