A Pareto-Efficient Task-Allocation Framework Based on Deep Reinforcement Learning Algorithm in MEC.
CollaborateCom (2)(2022)
摘要
Mobile-edge computing (MEC) has emerged as a promising paradigm that moves tasks running in the cloud to edge servers. In MEC systems, there are various individual requirements, such as less user-perceived time and lower energy consumption. In this case, substantial efforts have been paid to task allocation, aiming at enabling lower latency and higher resource utilization. However existing studies on multiple-objectives task allocation algorithms rarely consider the Pareto efficient problem, where no objective could be further improved without vitiating the other objectives optimization. In this paper, we propose a Pareto-efficient task-allocation framework based on a deep reinforcement learning algorithm. We give the formal formulations for objectives and construct a multi-objectives' optimization model for task allocation. Then a Pareto efficient algorithm is proposed to solve the problem of conflicting among multi-objectives. By coordinating multi-objectives parameters get from Pareto efficient algorithm, the deep reinforcement learning model takes a Pareto-efficient task allocation to improve real-time and resource utilization performance. We evaluate the proposed framework over various real-world tasks and compare it with existing allocating tasks models in edge computing networks. By using the proposed framework, we can get an accuracy that not be lower than 90% under the 0.6 s latency requirement. The simulation results also show that the proposed framework achieves lower latency and higher resource utilization compared to other task allocation methods.
更多查看译文
关键词
Mobile edge computing, Pareto-efficient, Task-allocation framework, Deep reinforcement learning algorithm
AI 理解论文
溯源树
样例
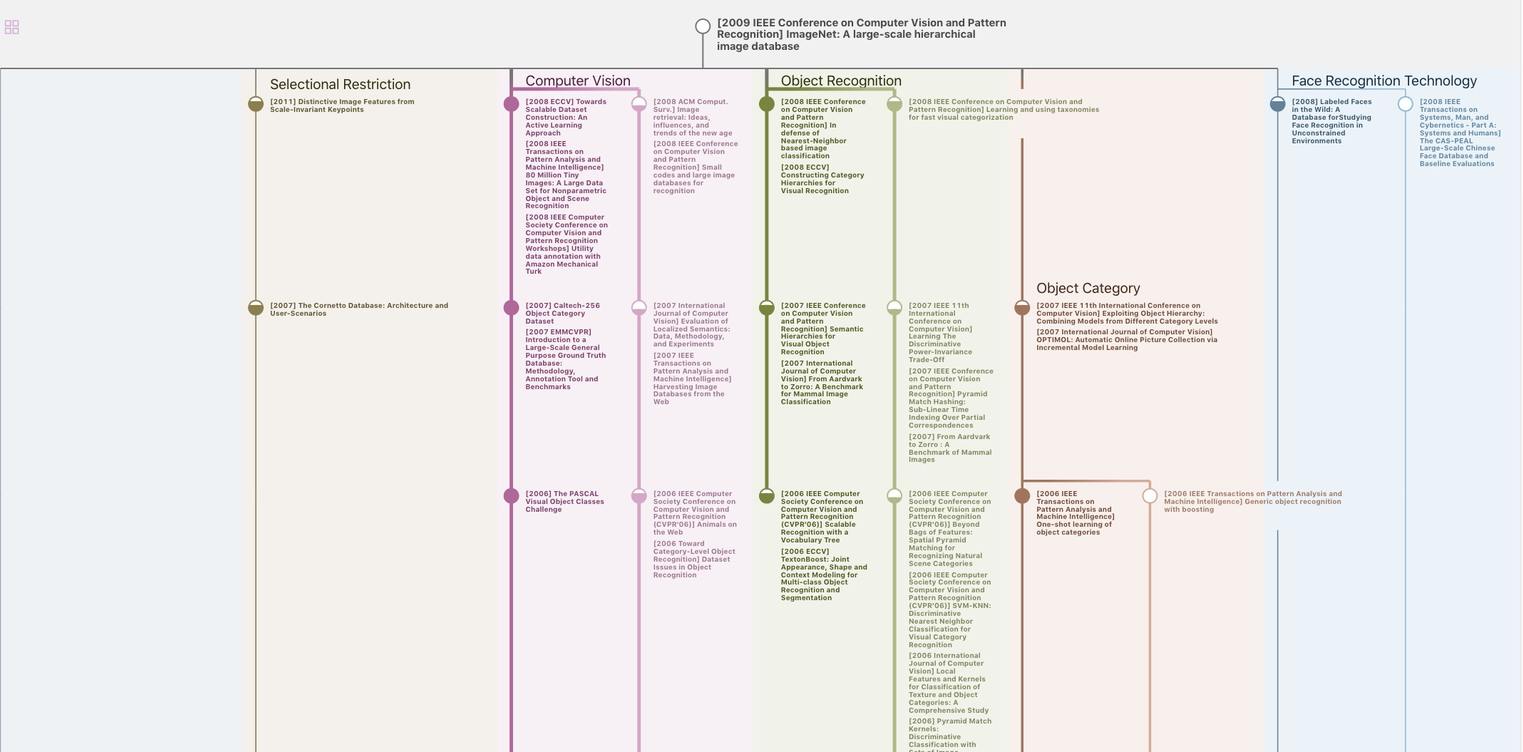
生成溯源树,研究论文发展脉络
Chat Paper
正在生成论文摘要