Monte Carlo Dropout Based BatchEnsemble For Improving Uncertainty Estimation.
COMAD/CODS(2023)
摘要
Modelling uncertainty in deep learning is important for several high-risk applications such as autonomous driving and healthcare. Existing techniques for uncertainty modelling in deep learning such as Monte Carlo (MC) Dropout [1] and BatchEnsemble [2] suffer from some drawbacks. MC dropout shares parameters across models resulting in highly correlated predictions while BatchEnsemble requires storing additional parameters for each model in the ensemble. In our work, we aim to bring the best of both worlds by combining MC-dropout in the process of ensemble creation in BatchEnsemble. The proposed approach, Monte-Carlo BatchEnsemble, helps in generating ensembles with less correlation in prediction with the addition of a few parameters. The experimental results show the effectiveness of the proposed technique for image classification.
更多查看译文
AI 理解论文
溯源树
样例
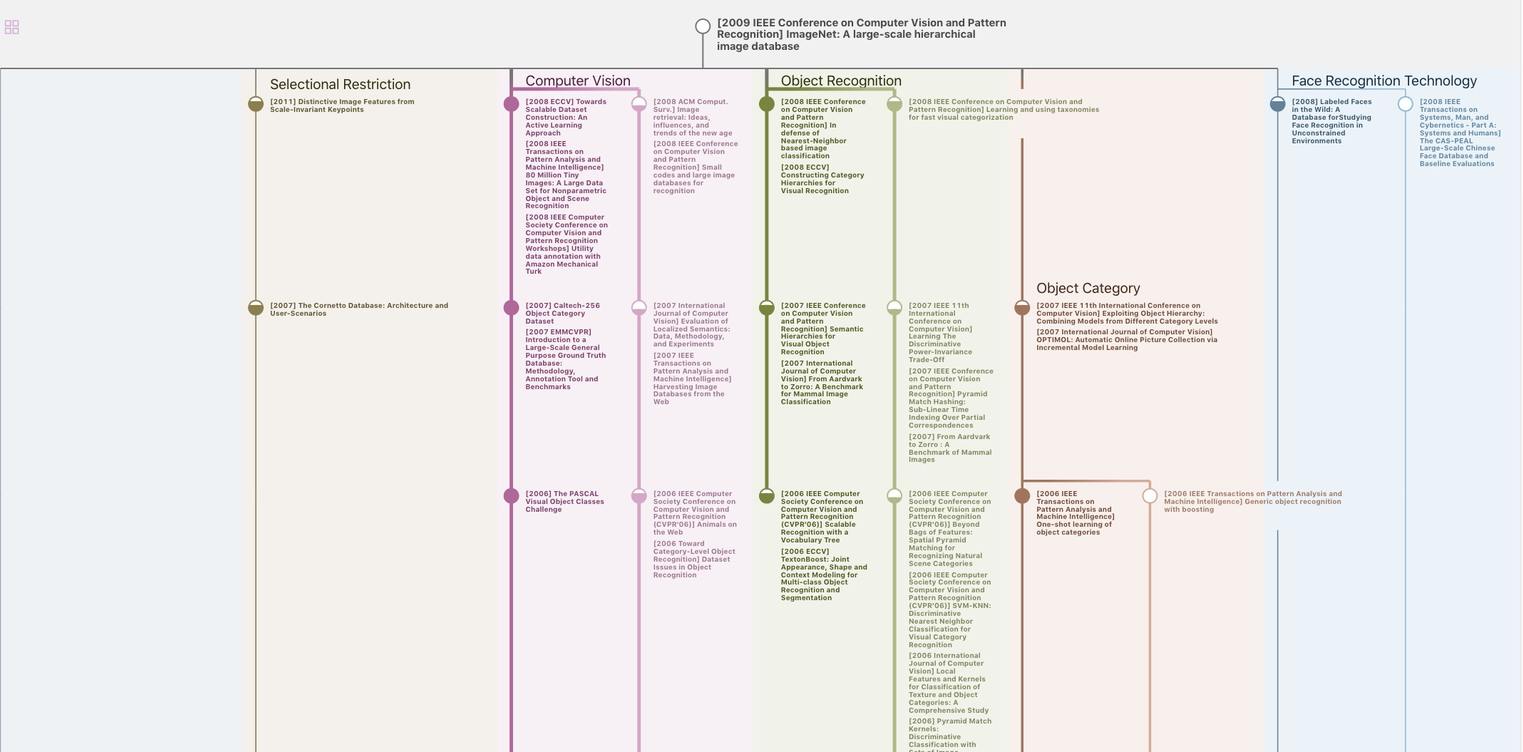
生成溯源树,研究论文发展脉络
Chat Paper
正在生成论文摘要