Evolving U-Nets Using Genetic Programming for Tree Crown Segmentation.
IVCNZ(2022)
摘要
The U-Net deep learning algorithm and its variants have been developed for biomedical image segmentation, and due to their success gained popularity in other science domains including remote sensing. So far no U-Net structure has been specifically designed to segment complex tree canopies from aerial imagery. In this paper, a handcrafted convolutional block is introduced to replace the raw convolutional block used in the standard U-Net structure. Furthermore, we proposed a Genetic Programming (GP) approach to evolving convolutional blocks used in the U-Net structure. The experimental results on a tree crown dataset show that both the handcrafted block and the GP evolved blocks have better segmentation results than the standard U-Net. Additionally, the U-Net using the proposed handcrafted blocks has fewer numbers of the learning parameters than the standard U-Net. Also, the proposed GP approach can evolve convolutional blocks used in U-Nets that perform better than the handcrafted U-Net and the standard U-Net, and can also achieve automation.
更多查看译文
关键词
genetic programming,tree,u-nets
AI 理解论文
溯源树
样例
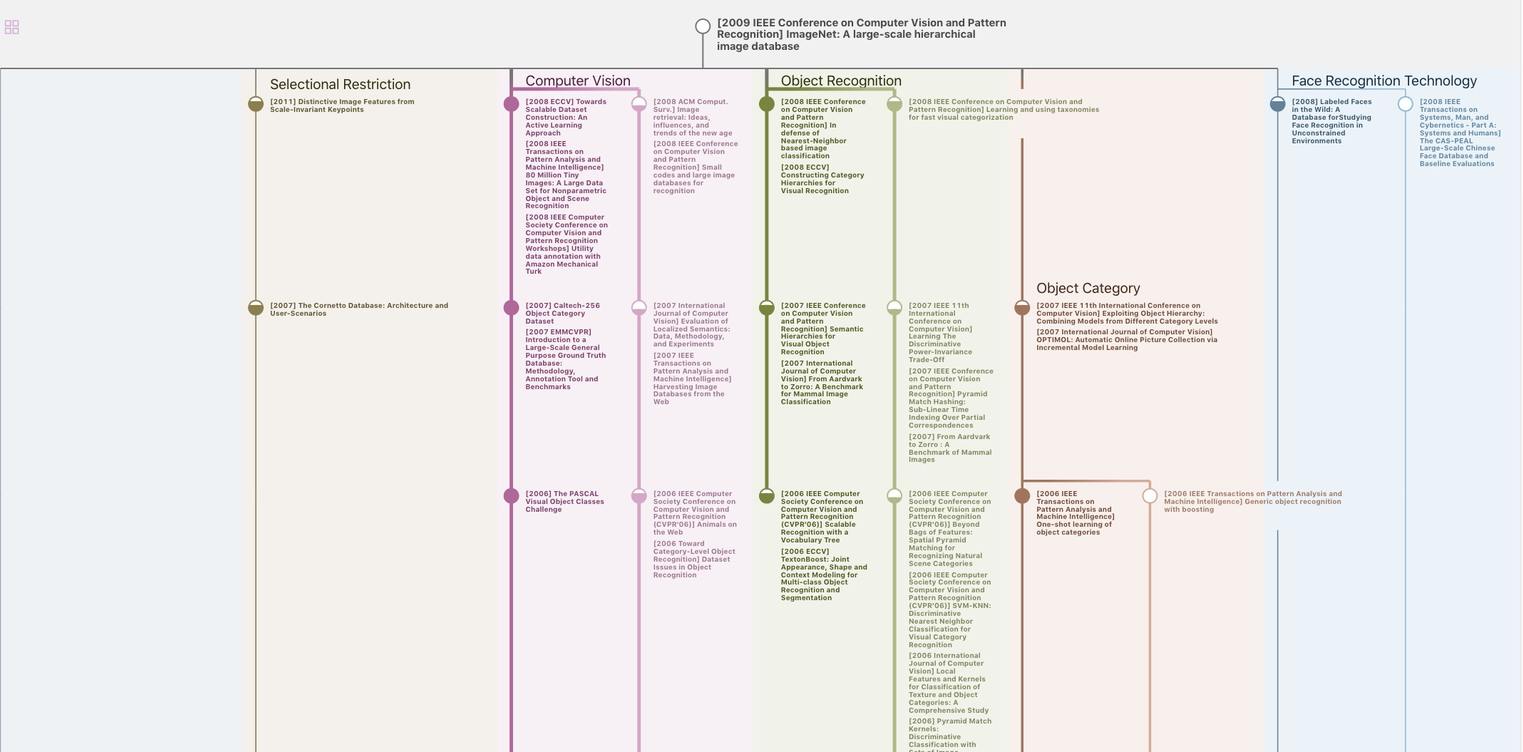
生成溯源树,研究论文发展脉络
Chat Paper
正在生成论文摘要