Unleashing the Potential of Adaptation Models via Go-getting Domain Labels.
ECCV Workshops (8)(2022)
摘要
In this paper, we propose an embarrassingly simple yet highly effective adversarial domain adaptation (ADA) method. We view ADA problem primarily from an optimization perspective and point out a fundamental dilemma, in that the real-world data often exhibits an imbalanced distribution where the large data clusters typically dominate and bias the adaptation process. Unlike prior works that either attempt loss re-weighting or data re-sampling for alleviating this defect, we introduce a new concept of go-getting domain labels (Go-labels) to replace the original immutable domain labels on the fly. The reason why call it as “Go-labels” is because “go-getting” means able to deal with new or difficult situations easily, like here Go-labels adaptively transfer the model attention from over-studied aligned data to those overlooked samples, which allows each sample to be well studied (i.e., alleviating data imbalance influence) and fully unleashes the potential of adaption model. Albeit simple, this dynamic adversarial domain adaptation framework with Go-labels effectively addresses data imbalance issue and promotes adaptation. We demonstrate through theoretical insights, empirical results on real data as well as toy games that our method leads to efficient training without bells and whistles, while being robust to different backbones.
更多查看译文
关键词
adaptation models,domain labels,go-getting
AI 理解论文
溯源树
样例
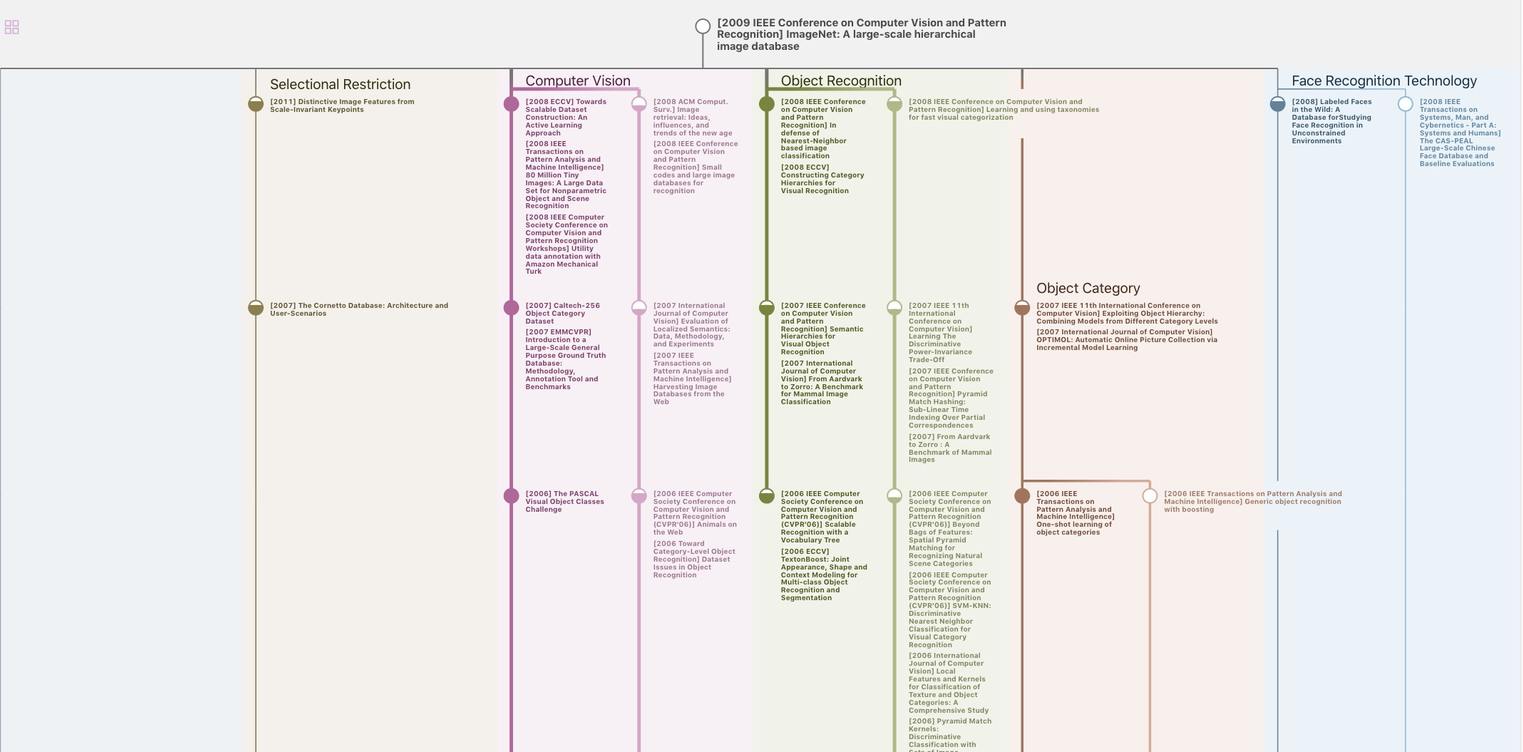
生成溯源树,研究论文发展脉络
Chat Paper
正在生成论文摘要