Implicit Map Augmentation for Relocalization.
ECCV Workshops (3)(2022)
摘要
Learning neural radiance fields (NeRF) has recently revolutionized novel view synthesis and related topics. The fact that the implicit scene models learned via NeRF greatly extend the representational capability compared with sparse maps, however, is largely overlooked. In this paper, we propose implicit map augmentation (IMA) that utilizes implicit scene representations to augment the sparse maps and help with visual relocalization. Given a sparse map reconstructed by structure-from-motion (SfM) or SLAM, the method first trains a NeRF model conditioned on the sparse reconstruction. Then an augmented sparse map representation can be sampled from the NeRF model to render better relocalization performance. Unlike the existing implicit mapping and pose estimation methods based on NeRF, IMA takes a hybrid approach by bridging the sparse map representation with MLP-based implicit representation in a non-intrusive way. The experiments demonstrate that our approach achieves better relocalization results with the augmented maps on challenging views. We also show that the resulting augmented maps not only remove the noisy 3D points but also bring back missing details that get discarded during the sparse reconstruction, which helps visual relocalization in wide-baseline scenarios.
更多查看译文
关键词
map
AI 理解论文
溯源树
样例
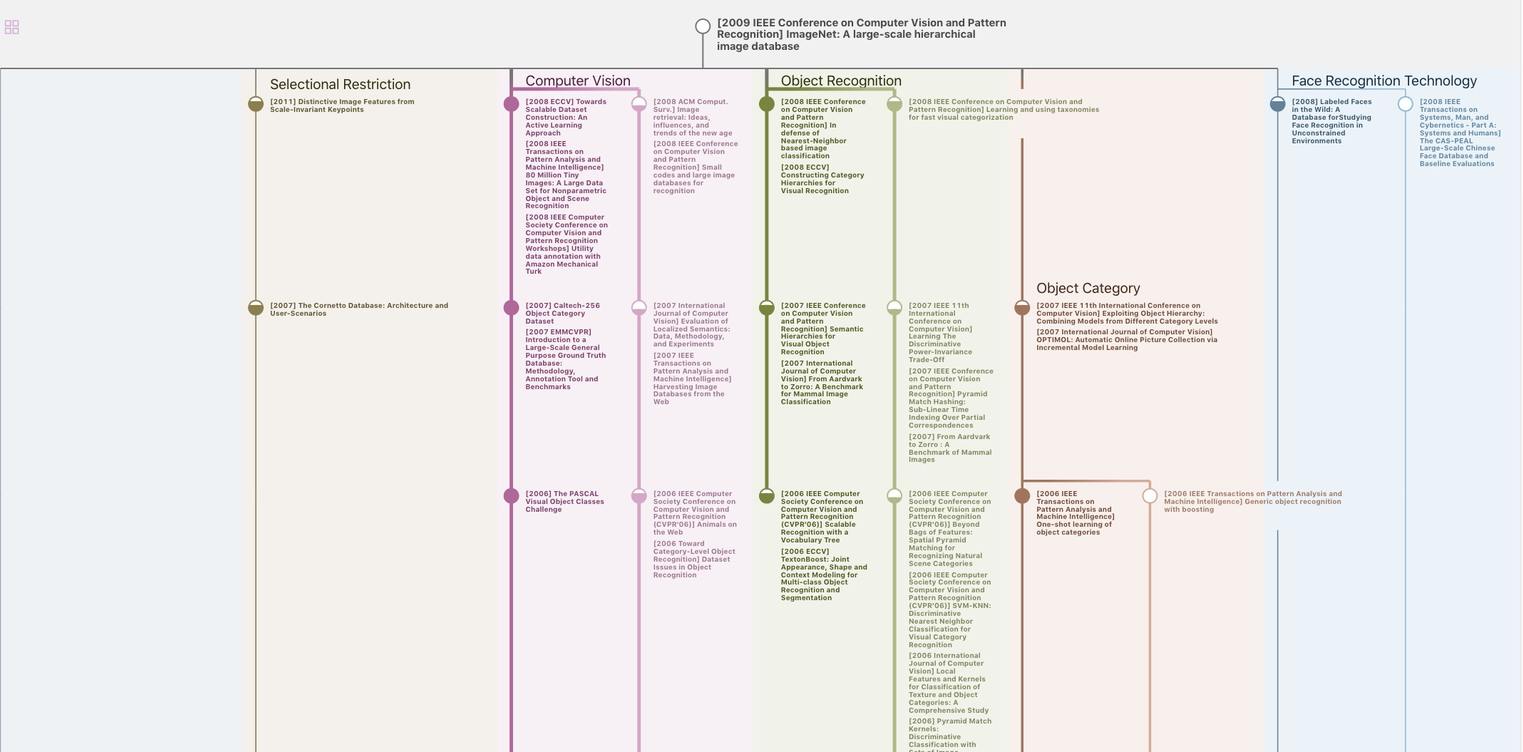
生成溯源树,研究论文发展脉络
Chat Paper
正在生成论文摘要