Traditional Chinese Medicine Health Status Identification with Graph Attention Network.
ML4CS (1)(2022)
摘要
The Traditional Chinese Medicine Health Status Identification plays an important role in TCM diagnosis and prescription recommendation. In this paper, we propose a method of Status Identification via Graph Attention Network, named SIGAT, which captures the complex medical correlation in the symptom-syndrome graph. More specifically, we construct a symptom-syndrome graph in that symptoms are taken as nodes and the edges are connected by syndromes. And we realize automatic induction of symptom to state element classification by using the attention mechanism and perceptron classifier. Finally, we conduct experiments by using hamming loss, coverage, 0/1 error, ranking loss, average precision, macro-F1 score, and micro-F1 score as evaluation metrics. The results demonstrate that the SIGAT model outperforms comparison algorithms on Traditional Chinese Medicine Prescription Dictionary dataset. The case study results suggest that the proposed method is a valuable way to identify the state element. The application of the graph attention network classification algorithm in TCM health status identification is of high precision and methodological feasibility.
更多查看译文
关键词
Graph attention network, Multi-label classification, TCM health status identification
AI 理解论文
溯源树
样例
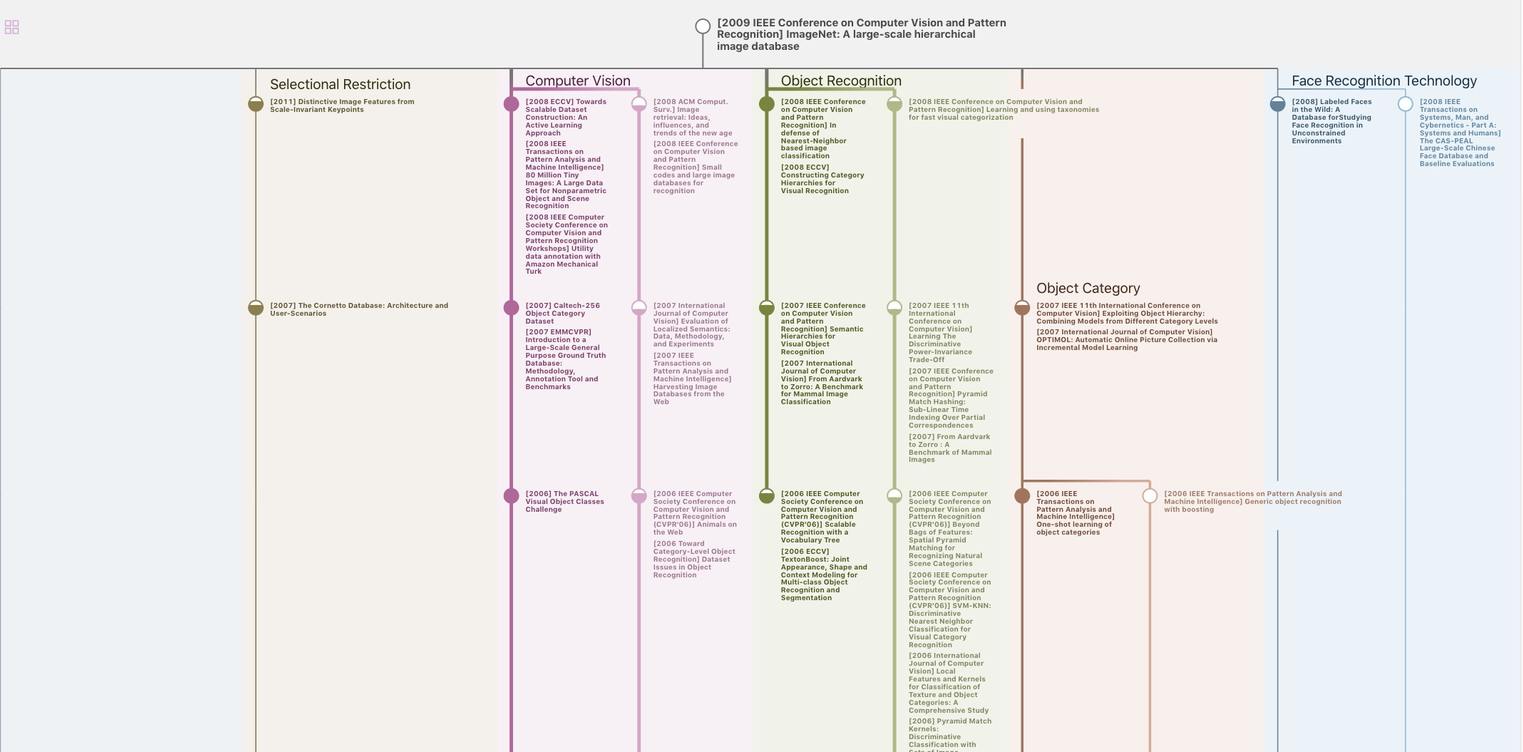
生成溯源树,研究论文发展脉络
Chat Paper
正在生成论文摘要