RGB road scene material segmentation
Image and Vision Computing(2024)
摘要
We introduce RGB road scene material segmentation, i.e., per-pixel segmentation of materials in real-world driving views with pure RGB images, as a novel computer vision task by building a benchmark dataset and by deriving a new method. Our dataset, KITTI-Materials, is based on the well-established KITTI dataset and consists of 1000 frames covering 24 different road scenes of urban/suburban landscapes, carefully annotated with one of 20 material categories for every pixel. It is the first dataset for RGB material segmentation in real driving scenes. Through careful analysis of KITTI-Materials, we identify the extraction and fusion of texture and image context as the key to accurate modeling of road scene material appearance. For this, we introduce Road scene Material Segmentation Network (RMSNet) as a baseline method for this challenging task. RMSNet encodes multi-scale hierarchical features with efficient Transformer layers. We construct the decoder of RMSNet based on a novel efficient self-attention model, which we refer to as SAMixer which adaptively fuses texture and context cues across multiple feature levels. Extensive experiments on KITTI-Materials validate the effectiveness of our RMSNet. We believe our work lays a solid foundation for further studies on RGB road scene material segmentation.
更多查看译文
关键词
RGB road scene material segmentation,Self-attention mechanism,Multi-level feature fusion
AI 理解论文
溯源树
样例
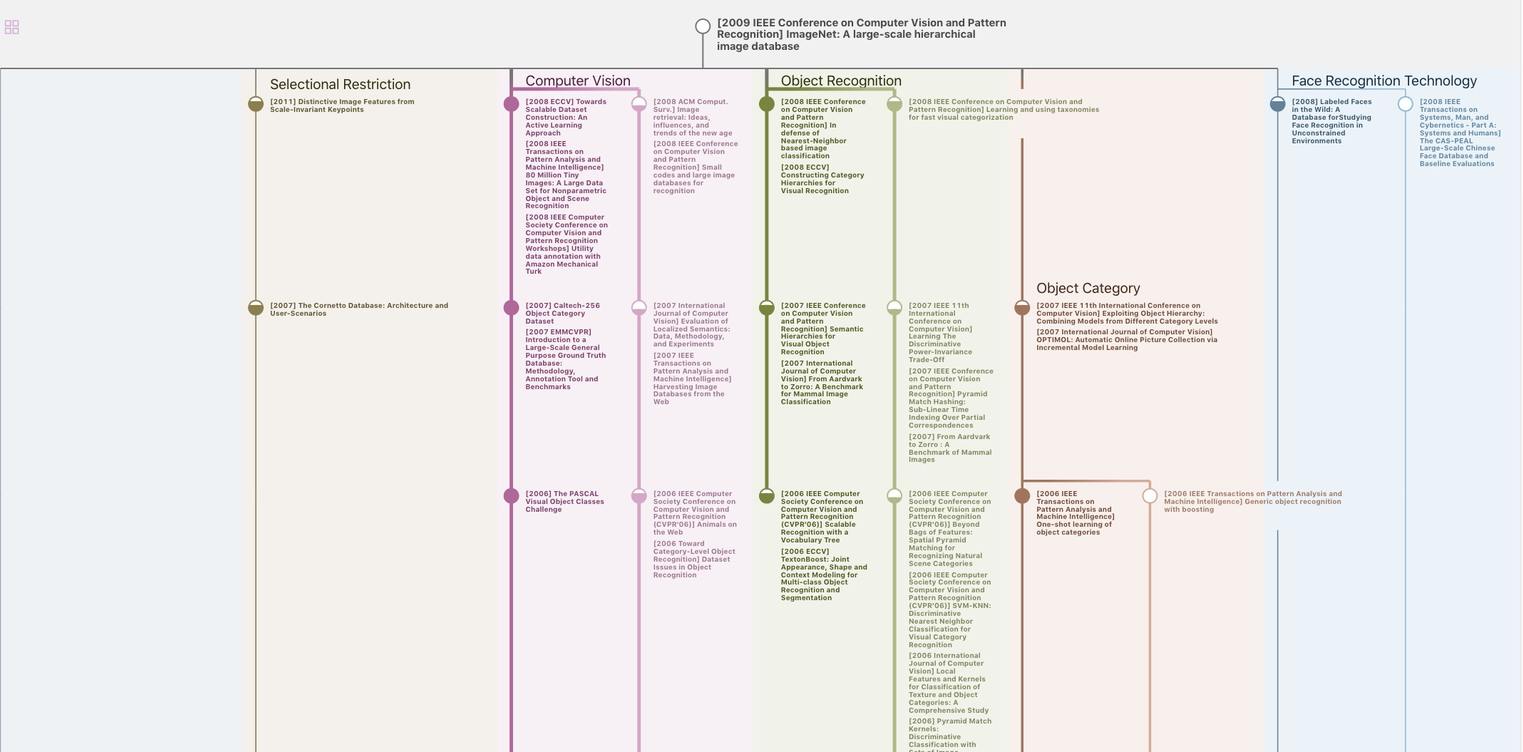
生成溯源树,研究论文发展脉络
Chat Paper
正在生成论文摘要