Enabling Machine Learning on Resource-constrained Tactical Networks.
MILCOM(2022)
摘要
In tactical networks, processing data located at the edge is difficult due to highly constrained computational and network resources. In order to more readily access this data, edge servers are deployed closer to the edge to lower latency. Due to rapidly changing network conditions, however, static offload decisions can quickly fail or become suboptimal. Therefore, there is a need to adaptively perform resource allocation at the tactical edge to ensure performance requirements. In this paper, we propose a system that allows execution of inference task applications throughout a network using a variety of adaptations to machine learning models that offer accuracy and latency trade-offs as conditions change. We present inference execution characterization results and then develop a resource allocation algorithm that optimally places tasks throughout a network. We present initial results of our system on a military relevant network and scenario.
更多查看译文
关键词
mobile edge computing, wireless network, optimization, tactical networks, edge machine learning
AI 理解论文
溯源树
样例
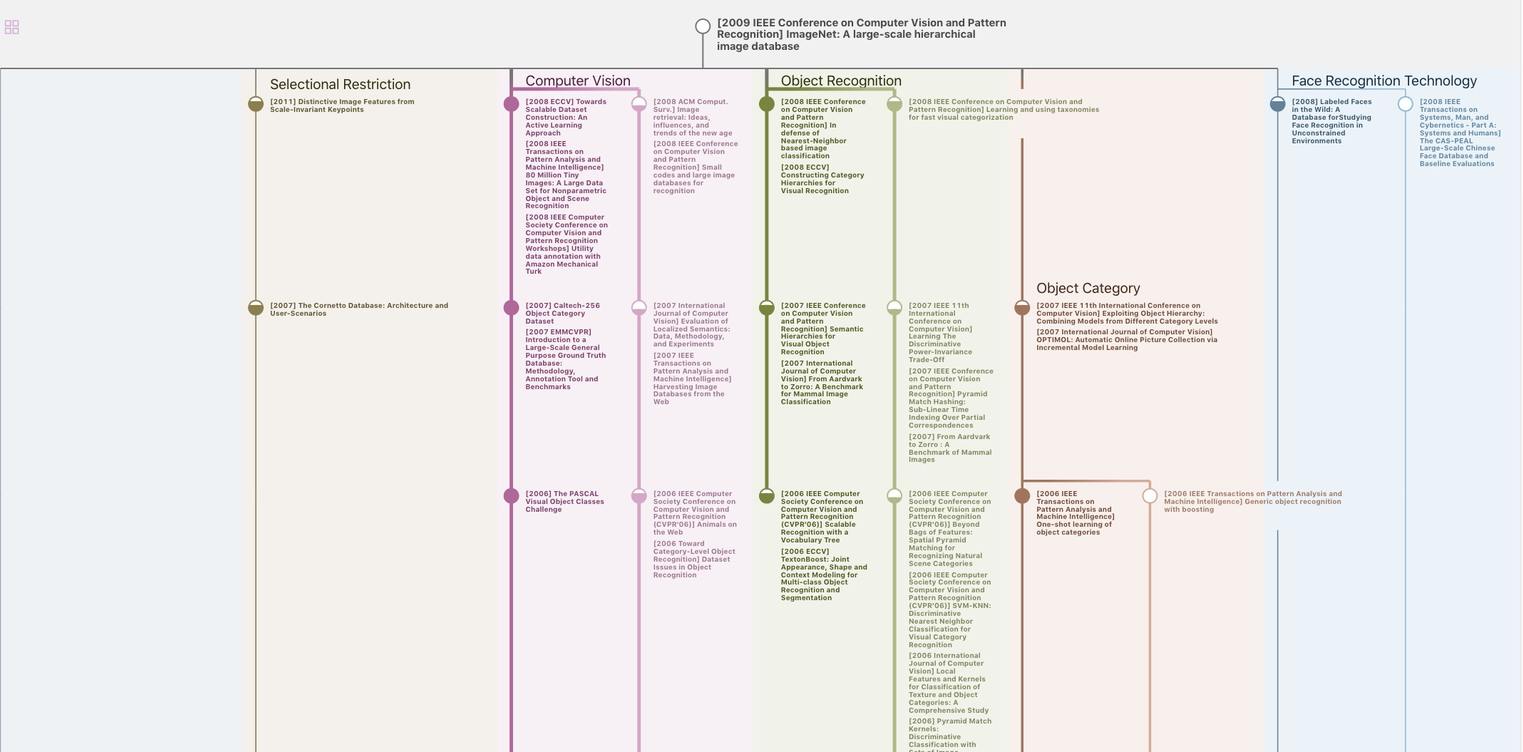
生成溯源树,研究论文发展脉络
Chat Paper
正在生成论文摘要