RangeGrad: Explaining Neural Networks by Measuring Uncertainty Through Bound Propagation.
PKDD/ECML Workshops (1)(2022)
摘要
When generating local neural network explanations, many methods remove or obfuscate information at the input and observe the effect on the neural network output. If the lack of certain information causes meaningful changes to the output, we assume it was important and forms part of the explanation for the prediction result. It is not trivial, however, to decide on a clear definition for the absence of information. Previous methods have blurred, darkened or added normally distributed noise to certain portions of the input. In this paper, we propose using interval bounds as a proxy for uncertainty about, or absence of, information. Using this insight, we developed RangeGrad, a novel method for generating saliency maps for neural networks. This method exploits the relationship between uncertainty on the input with prediction uncertainty. We show that the uncertainty framework produces valid explanations in line with existing methods.
更多查看译文
关键词
neural networks,bound propagation,uncertainty
AI 理解论文
溯源树
样例
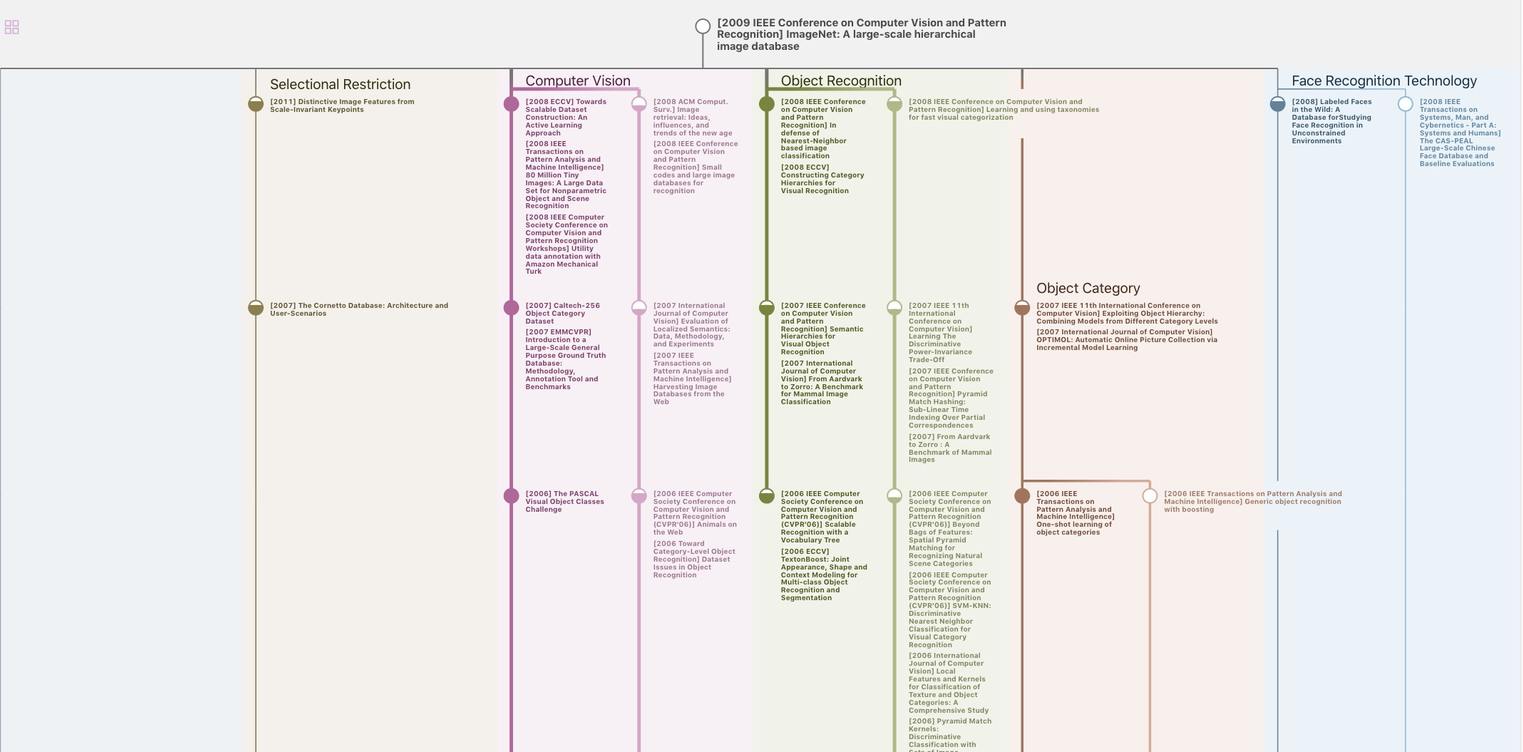
生成溯源树,研究论文发展脉络
Chat Paper
正在生成论文摘要