Online Estimation of Self-Body Deflection With Various Sensor Data Based on Directional Statistics.
SII(2023)
摘要
In this paper, we propose a method for online estimation of the robot's posture. Our method uses von Mises and Bingham distributions as probability distributions of joint angles and 3D orientation, which are used in directional statistics. We constructed a particle filter using these distributions and configured a system to estimate the robot's posture from various sensor information (e.g., joint encoders, IMU sensors, and cameras). Furthermore, unlike tangent space approximations, these distributions can handle global features and represent sensor characteristics as observation noises. As an application, we show that the yaw drift of a 6-axis IMU sensor can be represented probabilistically to prevent adverse effects on attitude estimation. For the estimation, we used an approximate model that assumes the actual robot posture can be reproduced by correcting the joint angles of a rigid body model. In the experiment part, we tested the estimator's effectiveness by examining that the joint angles generated with the approximate model can be estimated using the link pose of the same model. We then applied the estimator to the actual robot and confirmed that the gripper position could be estimated, thereby verifying the validity of the approximate model in our situation.
更多查看译文
关键词
directional statistics,various sensor data,estimation,self-body
AI 理解论文
溯源树
样例
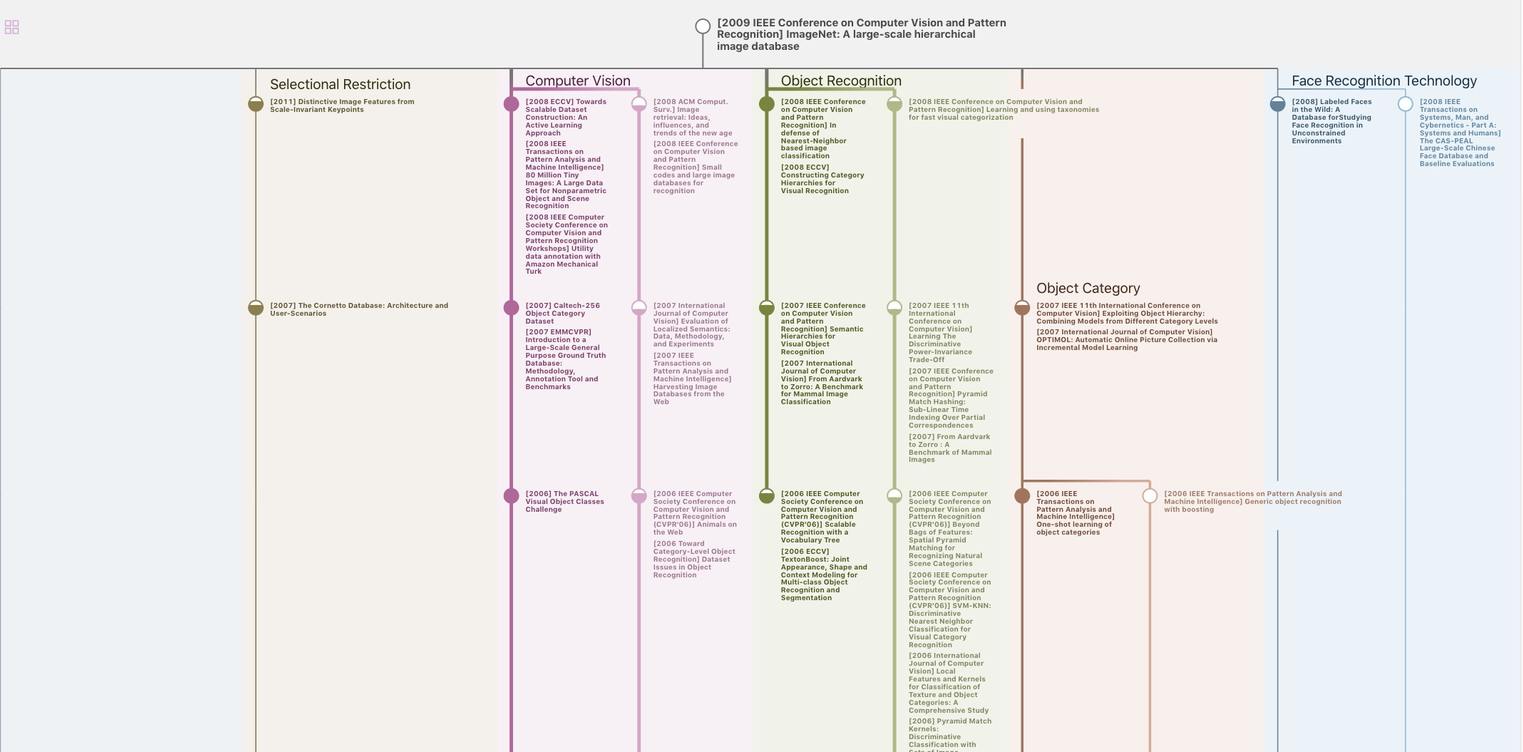
生成溯源树,研究论文发展脉络
Chat Paper
正在生成论文摘要