An Unsupervised Cluster Analysis of Post-COVID-19 Mental Health Outcomes and Associated Comorbidities.
AMIA(2022)
摘要
The COVID-19 pandemic continues to be widespread, and little is known about mental health impacts from dealing with the disease itself. This retrospective study used a deidentified health information exchange (HIE) dataset of electronic health record data from the state of Rhode Island and characterized different subgroups of the positive COVID-19 population. Three different clustering methods were explored to identify patterns of condition groupings in this population. Increased incidence of mental health conditions was seen post-COVID-19 diagnosis, and these individuals exhibited higher prevalence of comorbidities compared to the negative control group. A self-organizing map cluster analysis showed patterns of mental health conditions in half of the clusters. One mental health cluster revealed a higher comorbidity index and higher severity of COVID-19 disease. The clinical features identified in this study motivate the need for more in-depth analysis to predict and identify individuals at high risk for developing mental illness post-COVID-19 diagnosis.
更多查看译文
关键词
associated comorbidities,mental health,unsupervised cluster analysis,post-covid
AI 理解论文
溯源树
样例
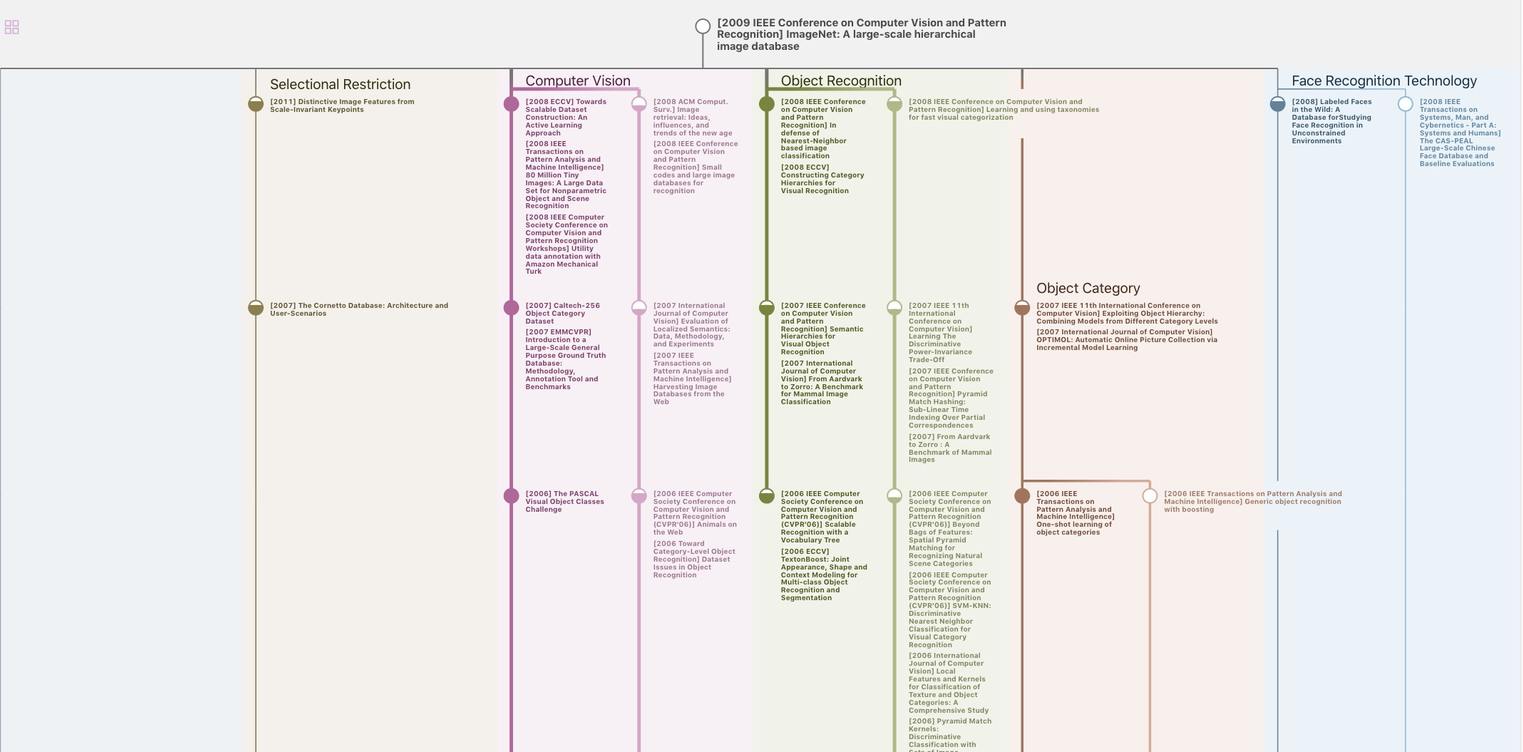
生成溯源树,研究论文发展脉络
Chat Paper
正在生成论文摘要