Multi-Layer Attention-based State Representation for the Reinforcement Learning of Visual Servoing.
ICCE(2023)
摘要
In this paper, we propose multi-layer attention-based state representation for robotic visual servoing using reinforcement learning. It is challenging for a robot to manipulate an object that it has never been trained on, which can occur in real use cases. Multiple attention layers allow the model to extract the spatial features of the object in the scene from high to low resolution in latent space. Using the extracted features allows the robotic controller model to learn more robust trajectory planning. We evaluated and compared the robustness of our method with that of other methods on a fetch-reach task in a simulation by randomly scaling the size of the target object. The results demonstrated that our method yielded the highest success rate, with the most efficient trajectory planning, which indicates that our state representation model provided more useful information that enabled visual servoing to perform more robustly for unseen objects.
更多查看译文
关键词
representation learning,autoencoder,visual servoing,reinforcement learning
AI 理解论文
溯源树
样例
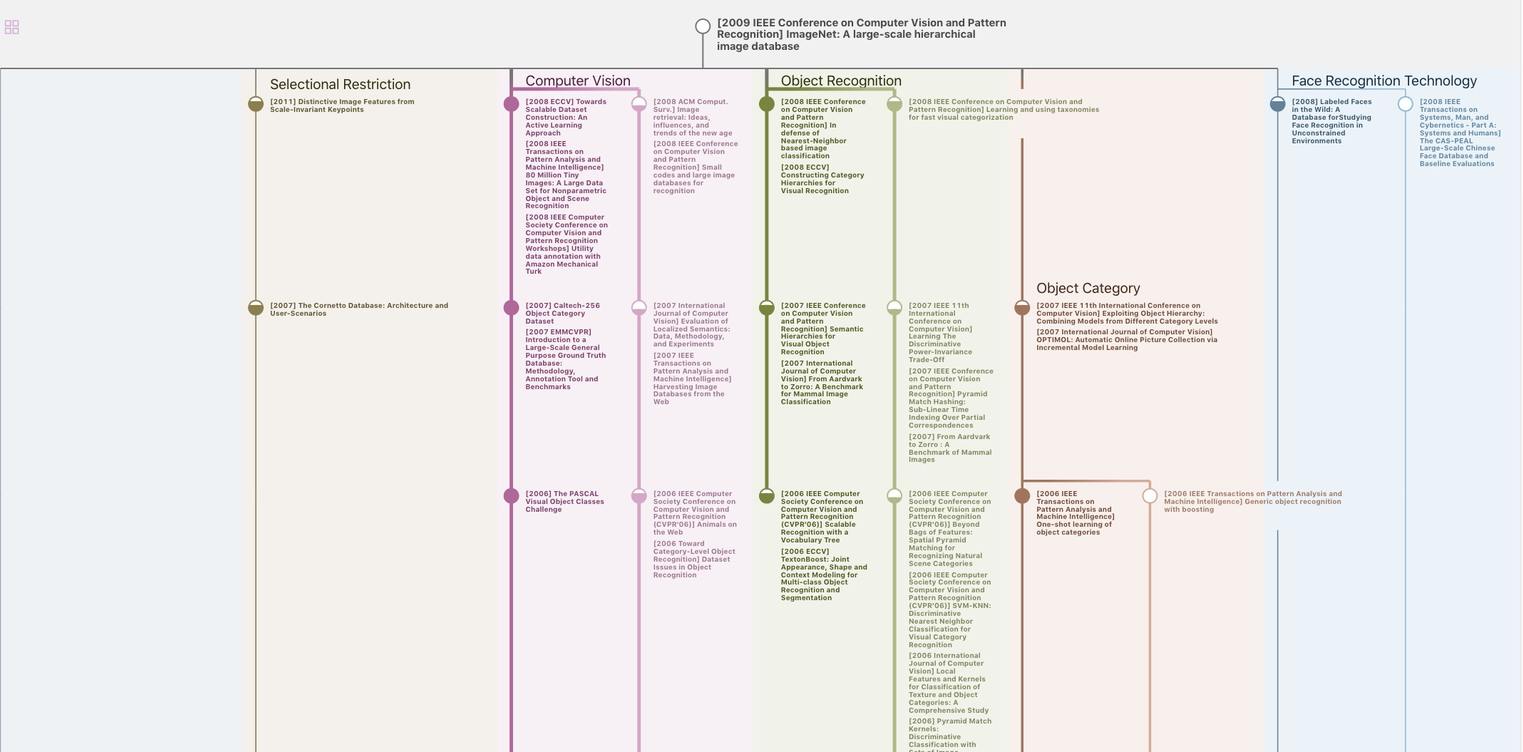
生成溯源树,研究论文发展脉络
Chat Paper
正在生成论文摘要