Structure-Attribute Social Network Graph Data Publishing Satisfying Differential Privacy.
Symmetry(2022)
摘要
With the development of big data, data collection and publishing are symmetrical. The purpose of data collection is to better publish data. To better collect user data and promote data analysis, publishing massive amounts of data can better provide services for people's lives. However, in the process of publishing data, the problem of low data availability caused by over protection is widespread. In addition, the attacker indirectly obtains the data of the target user by accessing the data of the user's friends or neighbors, which leads to the disclosure of the user's privacy. In order to solve these problems, a structure-attribute social network data publishing model is proposed. This model protects the privacy of user attribute data and prevents homogeneity attacks through attribute data perturbation. In addition, the model disrupts the structure of social networks by introducing uncertainty graphs into network partitions to generate published social network data. Our scheme has been tested on three public datasets, and the results show that our scheme can retain the social network structure as much as possible.
更多查看译文
关键词
attribute information,community division,uncertainty graph
AI 理解论文
溯源树
样例
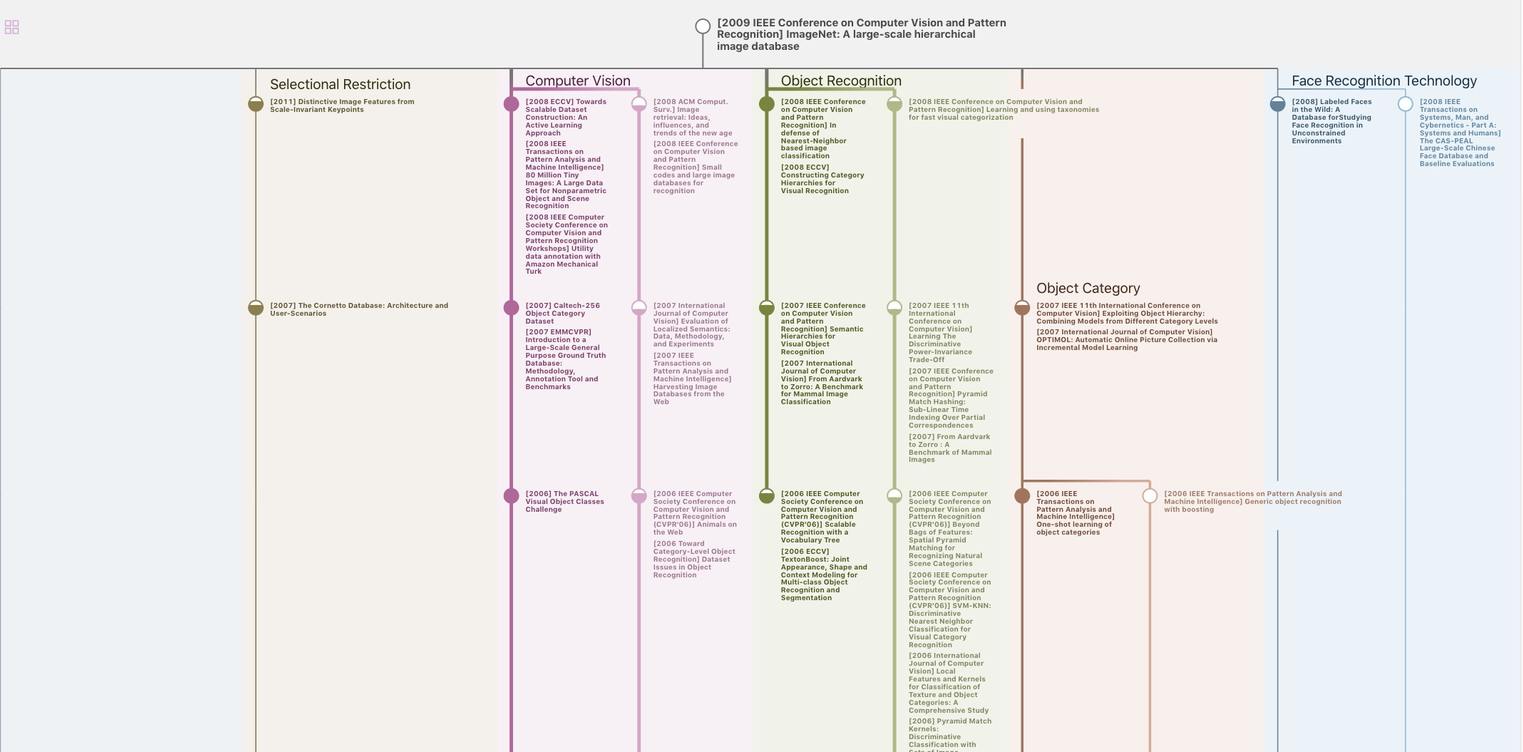
生成溯源树,研究论文发展脉络
Chat Paper
正在生成论文摘要