Absolute 3D Human Pose Estimation Using Noise-Aware Radial Distance Predictions.
Symmetry(2023)
摘要
We present a simple yet effective pipeline for absolute three-dimensional (3D) human pose estimation from two-dimensional (2D) joint keypoints, namely, the 2D-to-3D human pose lifting problem. Our method comprises two simple baseline networks, a 3D conversion function, and a correction network. The former two networks predict the root distance and the root-relative joint distance simultaneously. Given the input and predicted distances, the 3D conversion function recovers the absolute 3D pose, and the correction network reduces 3D pose noise caused by input uncertainties. Furthermore, to cope with input noise implicitly, we adopt a Siamese architecture that enforces the consistency of features between two training inputs, i.e., ground truth 2D joint keypoints and detected 2D joint keypoints. Finally, we experimentally validate the advantages of the proposed method and demonstrate its competitive performance over state-of-the-art absolute 2D-to-3D pose-lifting methods.
更多查看译文
关键词
absolute 3D human pose estimation,2D-to-3D human pose lifting,distance prediction
AI 理解论文
溯源树
样例
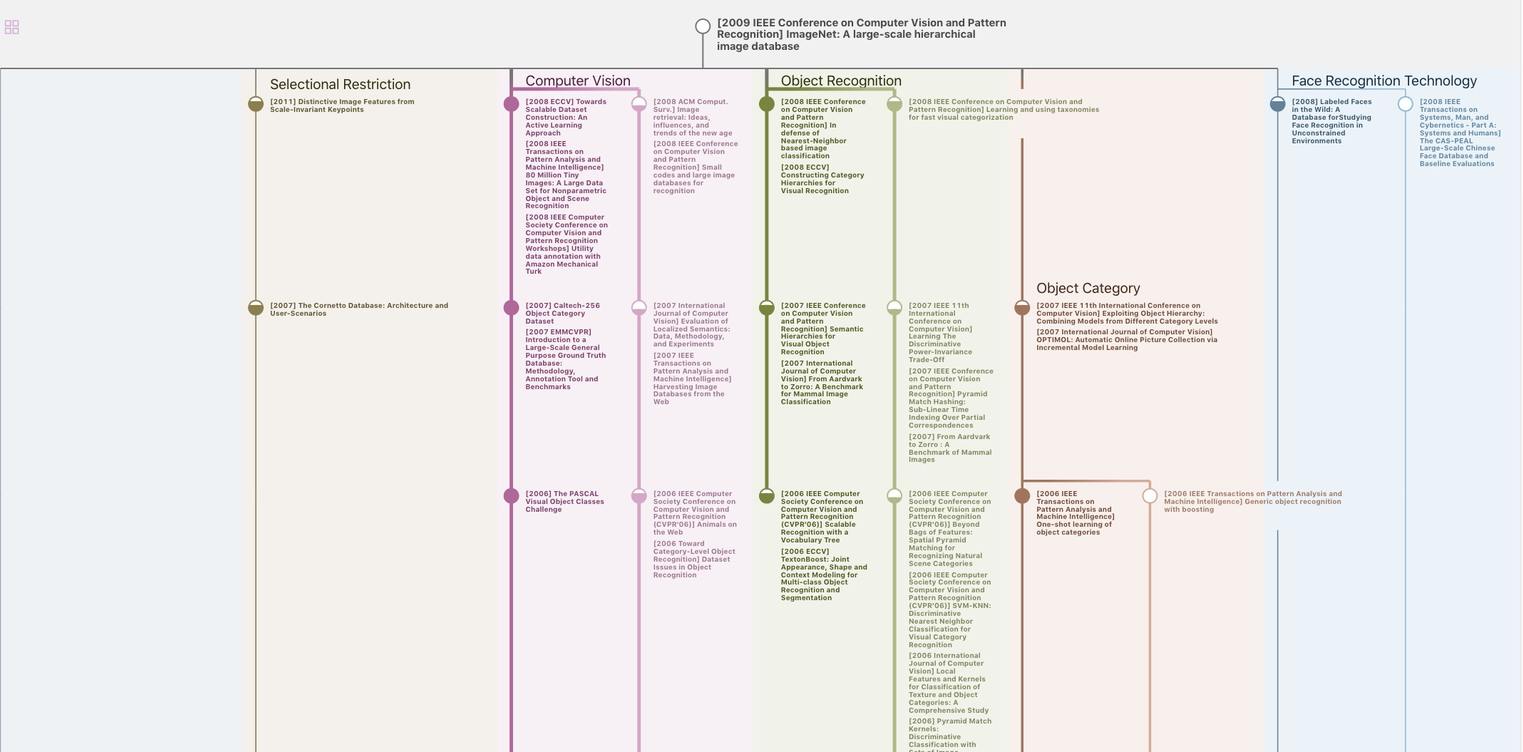
生成溯源树,研究论文发展脉络
Chat Paper
正在生成论文摘要