Evaluating state-of-the-art # SAT solvers on industrial configuration spaces
Empirical Software Engineering(2023)
摘要
Product lines are widely used to manage families of products that share a common base of features. Typically, not every combination (configuration) of features is valid. Feature models are a de facto standard to specify valid configurations and allow standardized analyses on the variability of the underlying system. A large variety of such analyses depends on computing the number of valid configurations. To analyze feature models, they are typically translated to propositional logic. This allows to employ # SAT solvers that compute the number of satisfying assignments of the propositional formula translated from a feature model. However, the # SAT problem is generally assumed to be even harder than SAT and its scalability when applied to feature models has only been explored sparsely. Our main contribution is an investigation of the performance of off-the-shelf # SAT solvers on computing the number of valid configurations for industrial feature models. We empirically evaluate 21 publicly available # SAT solvers on 130 feature models from 15 subject systems. Our results indicate that current solvers master a majority of the evaluated systems (13/15) with the fastest solvers requiring less than one second for each successfully evaluated feature model. However, there are two complex systems for which none of the evaluated solvers scales. For the given experiment design, the solvers that consumed the least runtime are sharpSAT (2.5 seconds in sum for the 13 systems) and Ganak (3.5 seconds).
更多查看译文
AI 理解论文
溯源树
样例
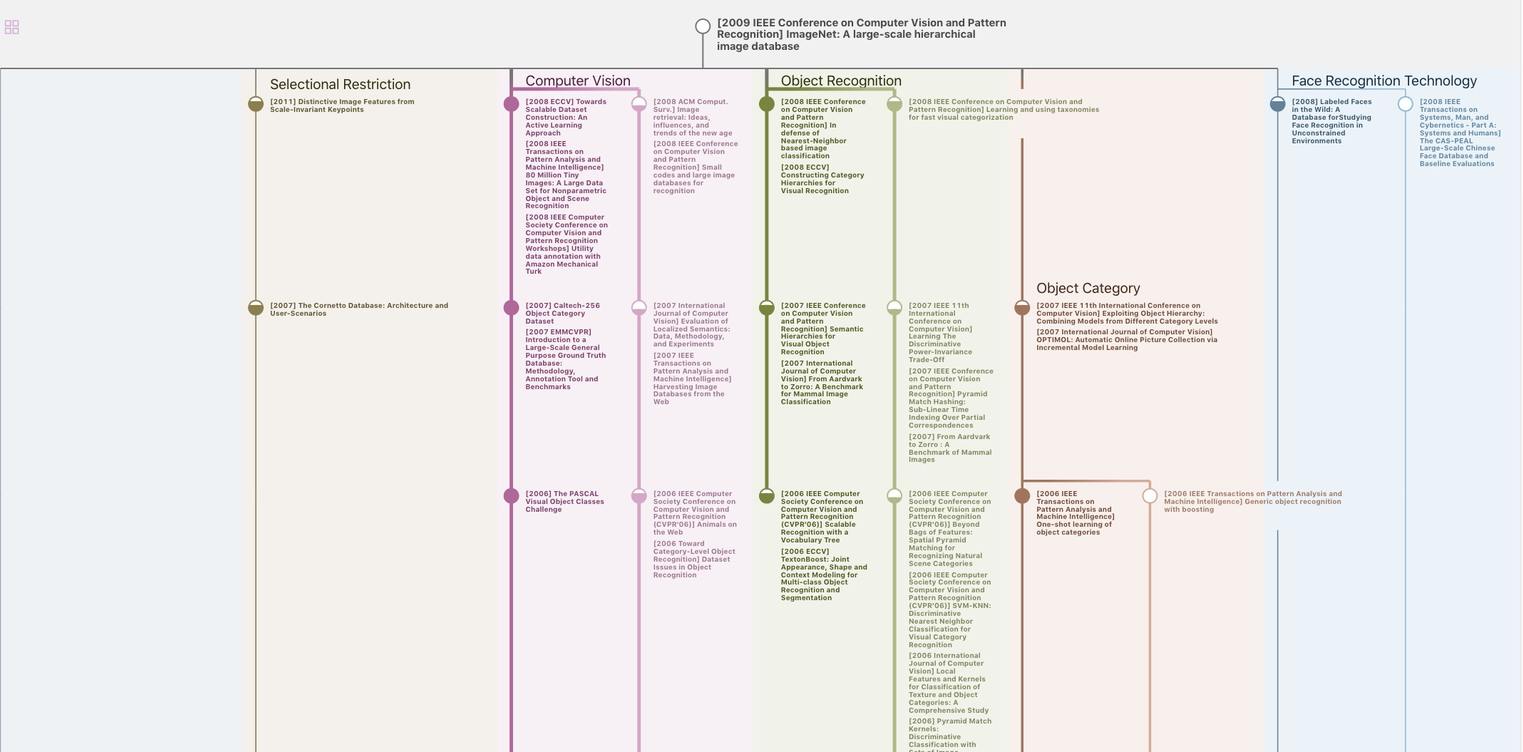
生成溯源树,研究论文发展脉络
Chat Paper
正在生成论文摘要