Automatic prediction of epileptic seizure using hybrid deep ResNet-LSTM model.
AI Commun.(2023)
摘要
Numerous advanced data processing and machine learning techniques for identifying epileptic seizures have been developed in the last two decades. Nonetheless, many of these solutions need massive data sets and intricate computations. Our approach transforms electroencephalogram (EEG) data into the time-frequency domain by utilizing a short-time fourier transform (STFT) and the spectrogram (t-f) images as the input stage of the deep learning model. Using EEG data, we have constructed a hybrid model comprising of a Deep Convolution Network (ResNet50) and a Long Short-Term Memory (LSTM) for predicting epileptic seizures. Spectrogram images are used to train the proposed hybrid model for feature extraction and classification. We analyzed the CHB-MIT scalp EEG dataset. For each preictal period of 5, 15, and 30 minutes, experiments are conducted to evaluate the performance of the proposed model. The experimental results indicate that the proposed model produced the optimum performance with a 5-minute preictal duration. We achieved an average accuracy of 94.5%, the average sensitivity of 93.7%, the f1-score of 0.9376, and the average false positive rate (FPR) of 0.055. Our proposed technique surpassed the random predictor and other current algorithms used for seizure prediction for all patients' data in the dataset. One can use the effectiveness of our proposed model to help in the early diagnosis of epilepsy and provide early treatment.
更多查看译文
关键词
STFT,ResNet-50,LSTM,deep-learning epilepsy,seizure prediction
AI 理解论文
溯源树
样例
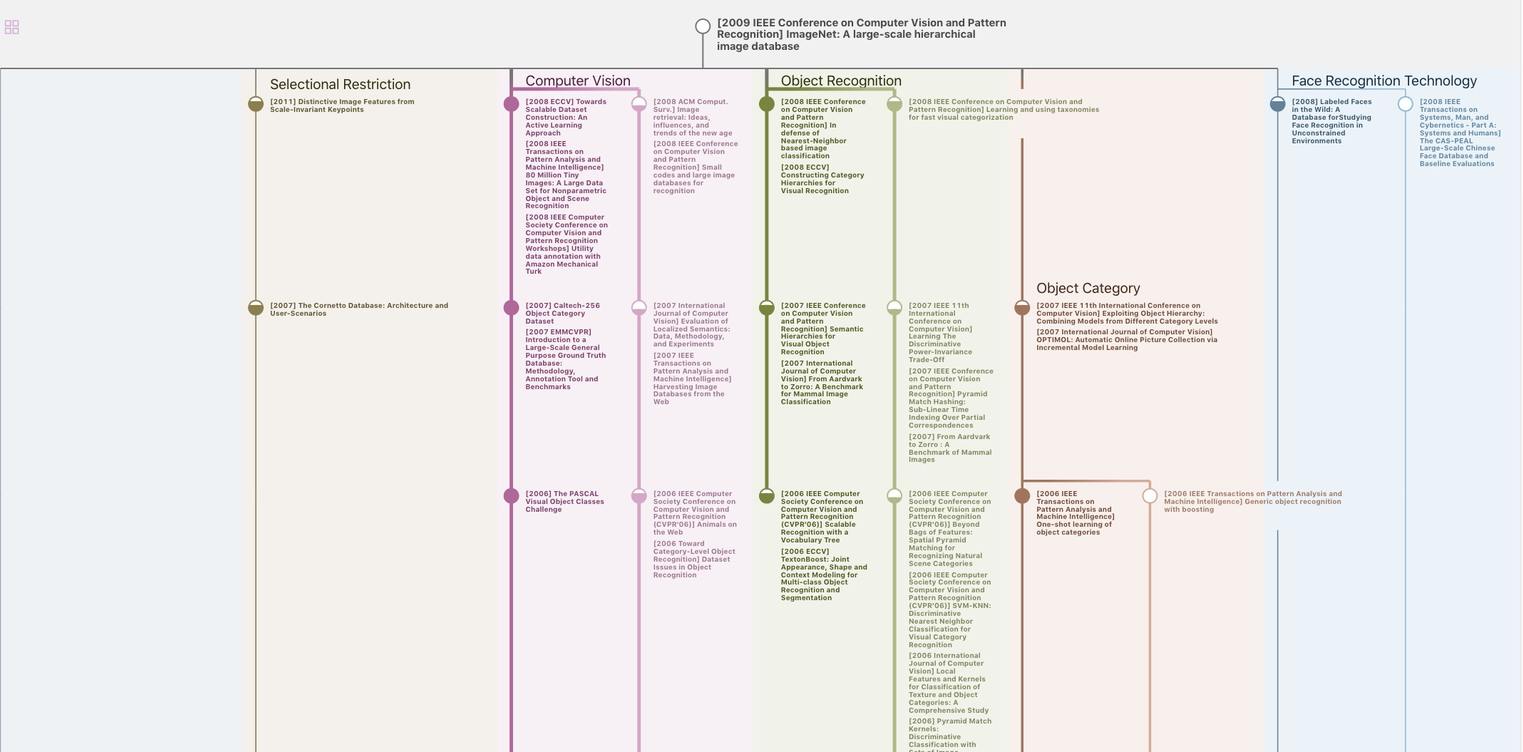
生成溯源树,研究论文发展脉络
Chat Paper
正在生成论文摘要