Supervised Machine-Learning Methodology for Industrial Robot Positional Health Using Artificial Neural Networks, Discrete Wavelet Transform, and Nonlinear Indicators.
Sensors (Basel, Switzerland)(2023)
摘要
Robotic systems are a fundamental part of modern industrial development. In this regard, they are required for long periods, in repetitive processes that must comply with strict tolerance ranges. Hence, the positional accuracy of the robots is critical, since degradation of this can represent a considerable loss of resources. In recent years, prognosis and health management (PHM) methodologies, based on machine and deep learning, have been applied to robots, in order to diagnose and detect faults and identify the degradation of robot positional accuracy, using external measurement systems, such as lasers and cameras; however, their implementation is complex in industrial environments. In this respect, this paper proposes a method based on discrete wavelet transform, nonlinear indices, principal component analysis, and artificial neural networks, in order to detect a positional deviation in robot joints, by analyzing the currents of the actuators. The results show that the proposed methodology allows classification of the robot positional degradation with an accuracy of 100%, using its current signals. The early detection of robot positional degradation, allows the implementation of PHM strategies on time, and prevents losses in manufacturing processes.
更多查看译文
关键词
Katz fractal dimension,accuracy degradation,discrete wavelet transform,fault prognosis,principal component analysis,robot health
AI 理解论文
溯源树
样例
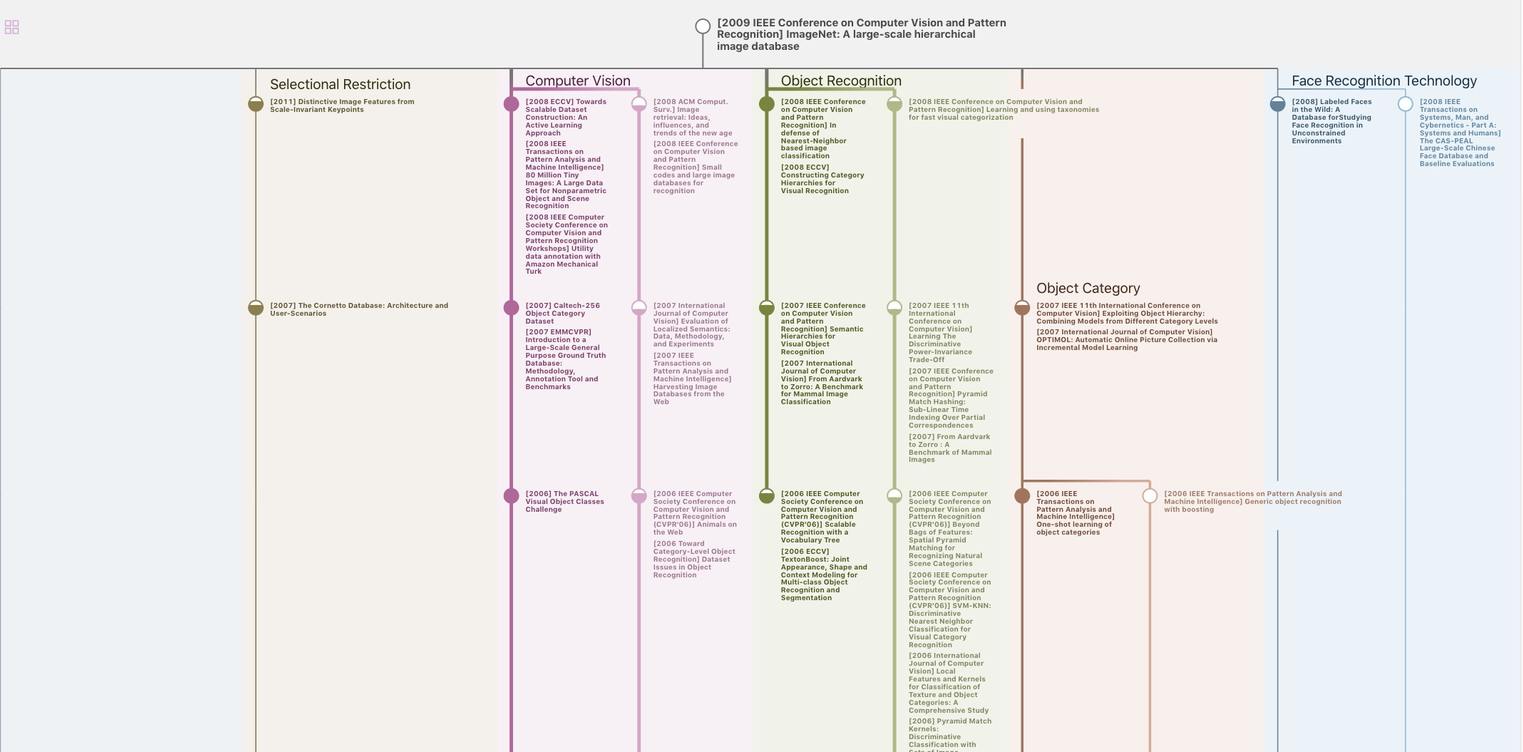
生成溯源树,研究论文发展脉络
Chat Paper
正在生成论文摘要