Transformer-Based Fire Detection in Videos.
Sensors (Basel, Switzerland)(2023)
摘要
Fire detection in videos forms a valuable feature in surveillance systems, as its utilization can prevent hazardous situations. The combination of an accurate and fast model is necessary for the effective confrontation of this significant task. In this work, a transformer-based network for the detection of fire in videos is proposed. It is an encoder-decoder architecture that consumes the current frame that is under examination, in order to compute attention scores. These scores denote which parts of the input frame are more relevant for the expected fire detection output. The model is capable of recognizing fire in video frames and specifying its exact location in the image plane in real-time, as can be seen in the experimental results, in the form of segmentation mask. The proposed methodology has been trained and evaluated for two computer vision tasks, the full-frame classification task (fire/no fire in frames) and the fire localization task. In comparison with the state-of-the-art models, the proposed method achieves outstanding results in both tasks, with 97% accuracy, 20.4 fps processing time, 0.02 false positive rate for fire localization, and 97% for f-score and recall metrics in the full-frame classification task.
更多查看译文
关键词
fire detection,fire localization,image classification,real-time,segmentation,transformers,videos
AI 理解论文
溯源树
样例
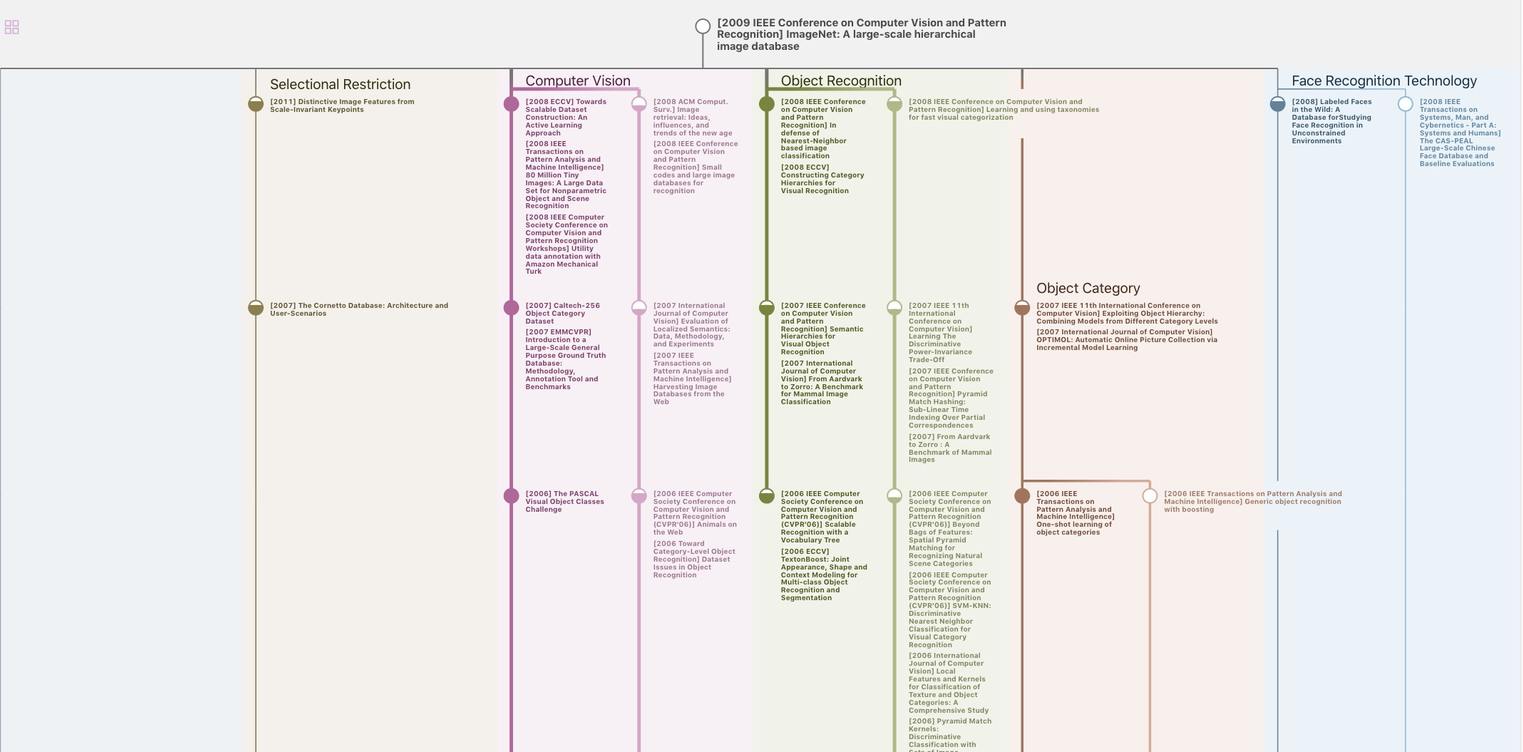
生成溯源树,研究论文发展脉络
Chat Paper
正在生成论文摘要