A contrastive learning framework enhanced by unlabeled samples for remaining useful life prediction
Reliability Engineering & System Safety(2023)
摘要
Deep learning (DL)-based methods for remaining useful life (RUL) prediction have received increasing research attention due to excellent feature extraction abilities. Most DL methods rely on abundant labeled samples for supervised training. However, because of the adoption of the over-maintenance strategy of equipment, the monitored data for the degradation of equipment usually consists of few labeled samples and a large amount of unlabeled samples, which limits the performance of DL methods. To take advantage of the value of unlabeled samples, this paper proposed a contrastive learning framework for RUL prediction. First, an unlabeled sample augmentation is developed firstly to extend the sample set. Then, an unlabeled sample learning (USL) architecture is proposed to learn the information of degradation from unlabeled samples to promote general DL models’ performance on RUL prediction. Based on the proposed framework, USL-convolutional neural network and USL-long short-term memory network are used to validate its performance based on datasets of turbofan engine and bearing. Results show that the performance of RUL prediction based on the proposed framework can be enhanced by unlabeled samples and verify the good scalability and generalization ability of the proposed framework.
更多查看译文
关键词
Deep learning,Remaining useful life,Unlabeled samples,Prognostics and health management,Sample augmentation
AI 理解论文
溯源树
样例
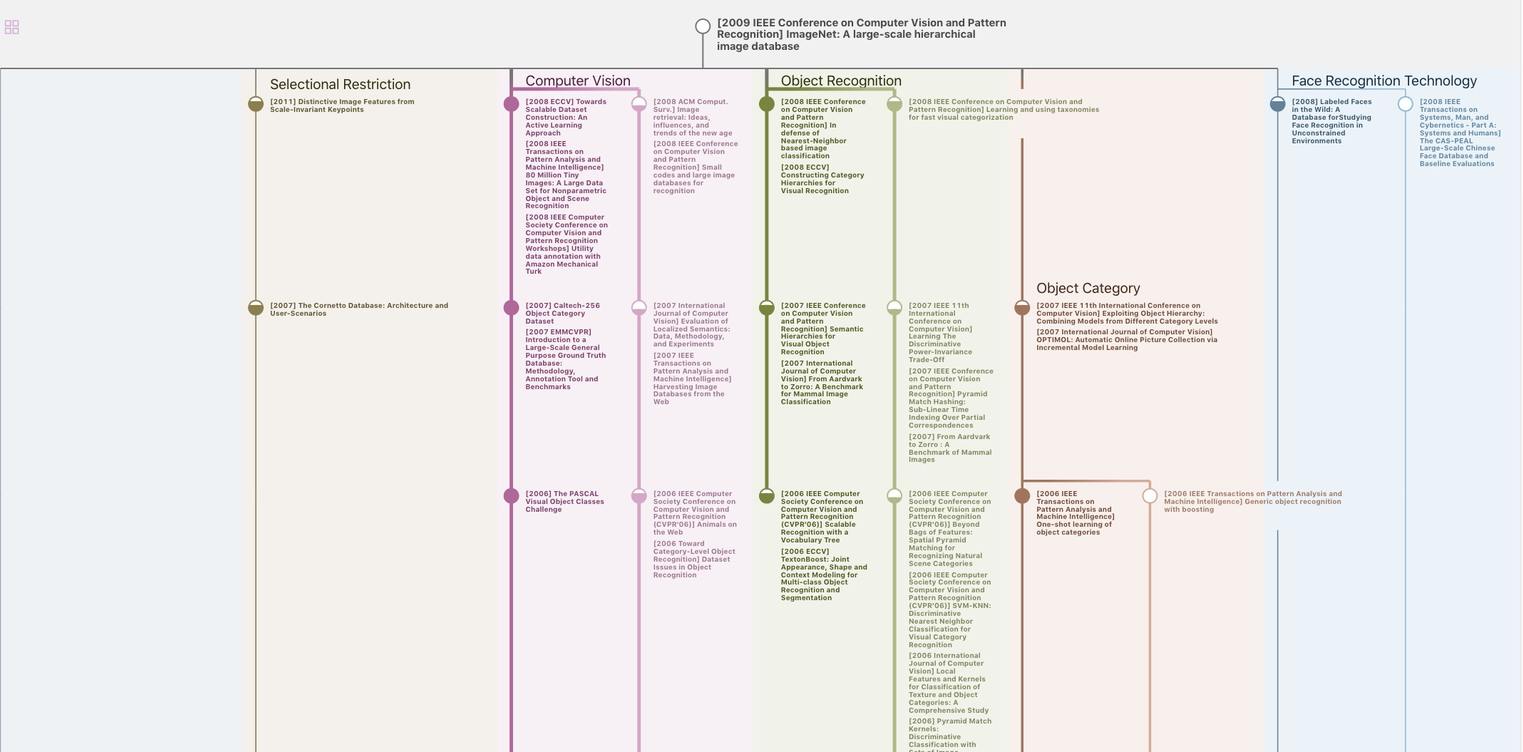
生成溯源树,研究论文发展脉络
Chat Paper
正在生成论文摘要