Data-driven multiscale modeling of subgrid parameterizations in climate models
CoRR(2023)
摘要
Subgrid parameterizations, which represent physical processes occurring below the resolution of current climate models, are an important component in producing accurate, long-term predictions for the climate. A variety of approaches have been tested to design these components, including deep learning methods. In this work, we evaluate a proof of concept illustrating a multiscale approach to this prediction problem. We train neural networks to predict subgrid forcing values on a testbed model and examine improvements in prediction accuracy that can be obtained by using additional information in both fine-to-coarse and coarse-to-fine directions.
更多查看译文
关键词
subgrid parameterizations,multiscale modeling,climate
AI 理解论文
溯源树
样例
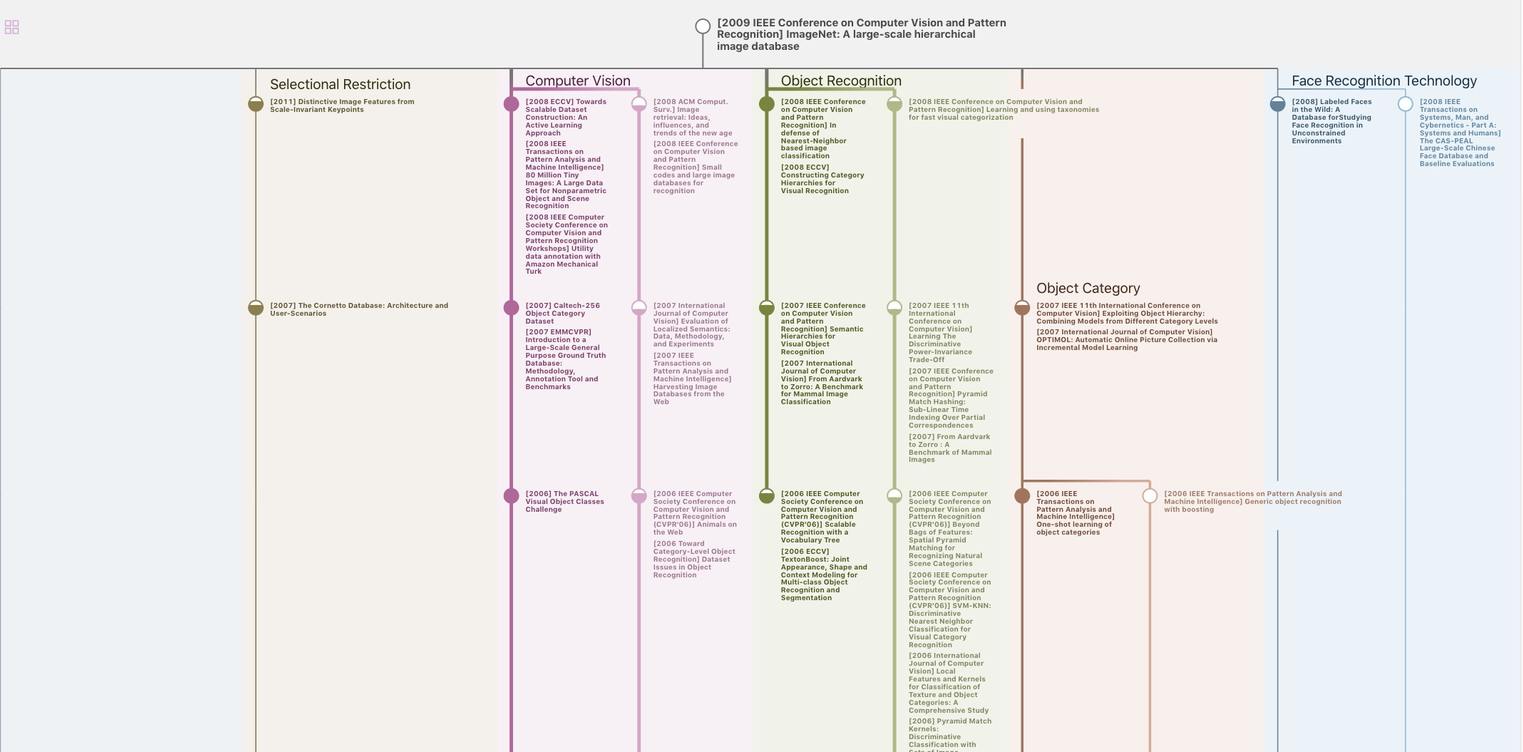
生成溯源树,研究论文发展脉络
Chat Paper
正在生成论文摘要