Mole Recruitment: Poisoning of Image Classifiers via Selective Batch Sampling
CoRR(2023)
摘要
In this work, we present a data poisoning attack that confounds machine learning models without any manipulation of the image or label. This is achieved by simply leveraging the most confounding natural samples found within the training data itself, in a new form of a targeted attack coined "Mole Recruitment." We define moles as the training samples of a class that appear most similar to samples of another class, and show that simply restructuring training batches with an optimal number of moles can lead to significant degradation in the performance of the targeted class. We show the efficacy of this novel attack in an offline setting across several standard image classification datasets, and demonstrate the real-world viability of this attack in a continual learning (CL) setting. Our analysis reveals that state-of-the-art models are susceptible to Mole Recruitment, thereby exposing a previously undetected vulnerability of image classifiers.
更多查看译文
关键词
image classifiers,selective batch
AI 理解论文
溯源树
样例
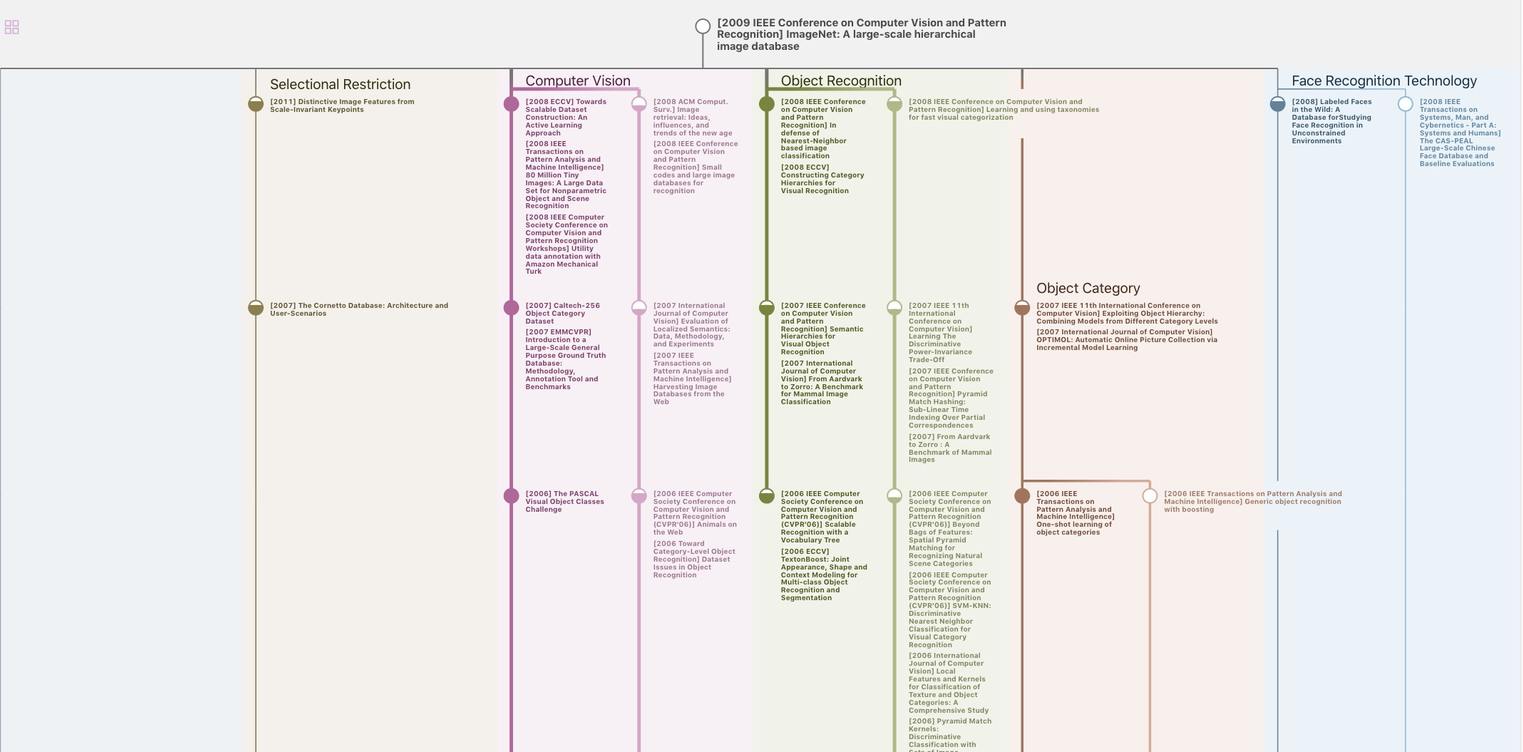
生成溯源树,研究论文发展脉络
Chat Paper
正在生成论文摘要