Application of Machine-Learning Algorithms for Better Understanding the Properties of Liquisolid Systems Prepared with Three Mesoporous Silica Based Carriers
Pharmaceutics(2023)
摘要
The processing of liquisolid systems (LSS), which are considered a promising approach to improving the oral bioavailability of poorly soluble drugs, has proven challenging due to the relatively high amount of liquid phase incorporated within them. The objective of this study was to apply machine-learning tools to better understand the effects of formulation factors and/or tableting process parameters on the flowability and compaction properties of LSS with silica-based mesoporous excipients as carriers. In addition, the results of the flowability testing and dynamic compaction analysis of liquisolid admixtures were used to build data sets and develop predictive multivariate models. In the regression analysis, six different algorithms were used to model the relationship between tensile strength (TS), the target variable, and eight other input variables. The AdaBoost algorithm provided the best-fit model for predicting TS (coefficient of determination = 0.94), with ejection stress (ES), compaction pressure, and carrier type being the parameters that influenced its performance the most. The same algorithm was best for classification (precision = 0.90), depending on the type of carrier used, with detachment stress, ES, and TS as variables affecting the performance of the model. Furthermore, the formulations with Neusilin((R)) US2 were able to maintain good flowability and satisfactory values of TS despite having a higher liquid load compared to the other two carriers.
更多查看译文
关键词
liquisolid systems,mesoporous silica carrier,flowability,direct compression,compaction behavior,machine-learning
AI 理解论文
溯源树
样例
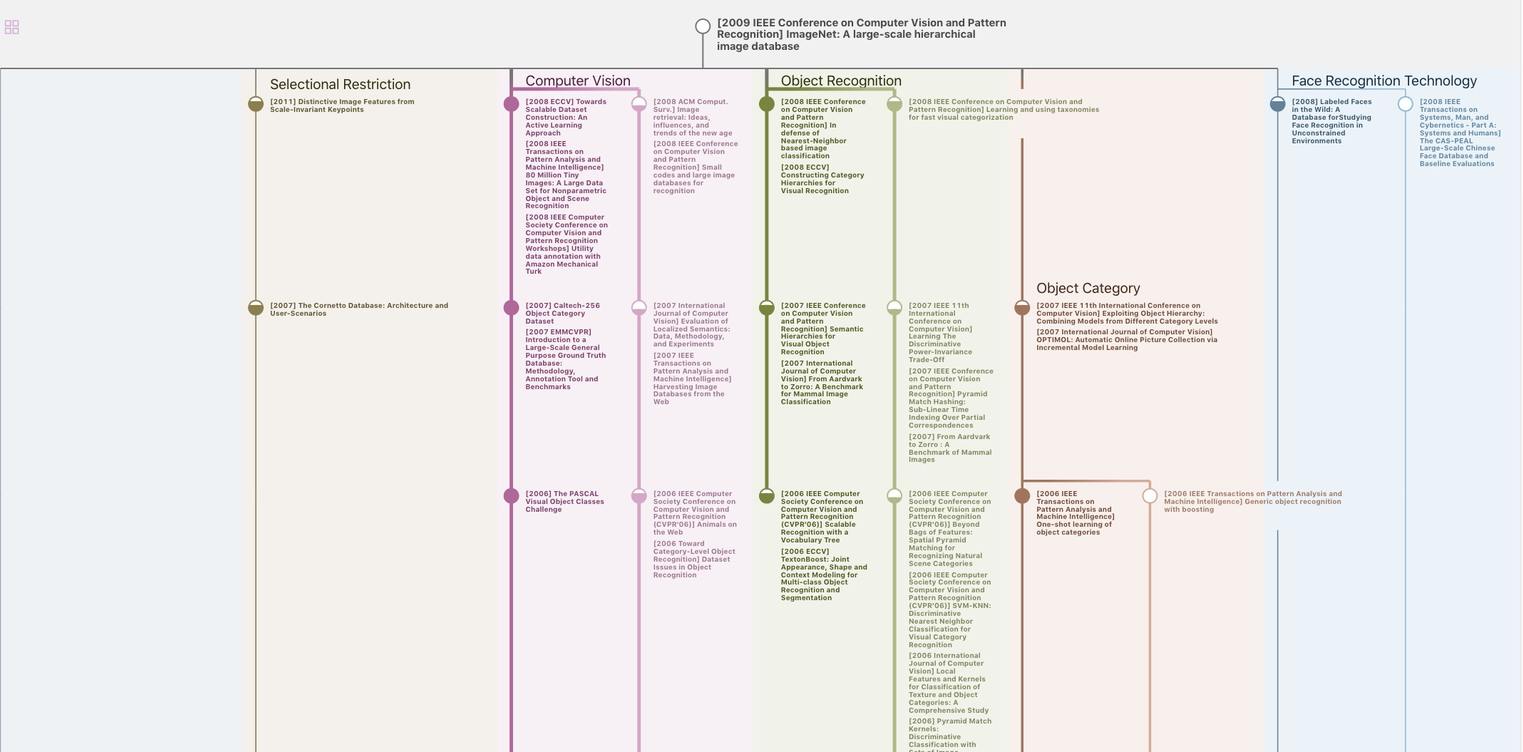
生成溯源树,研究论文发展脉络
Chat Paper
正在生成论文摘要