Molecular Property Prediction by Combining LSTM and GAT.
Biomolecules(2023)
摘要
Molecular property prediction is an important direction in computer-aided drug design. In this paper, to fully explore the information from SMILE stings and graph data of molecules, we combined the SALSTM and GAT methods in order to mine the feature information of molecules from sequences and graphs. The embedding atoms are obtained through SALSTM, firstly using SMILES strings, and they are combined with graph node features and fed into the GAT to extract the global molecular representation. At the same time, data augmentation is added to enlarge the training dataset and improve the performance of the model. Finally, to enhance the interpretability of the model, the attention layers of both models are fused together to highlight the key atoms. Comparison with other graph-based and sequence-based methods, for multiple datasets, shows that our method can achieve high prediction accuracy with good generalizability.
更多查看译文
关键词
artificial intelligence,deep learning,graph convolutional network,molecular representation
AI 理解论文
溯源树
样例
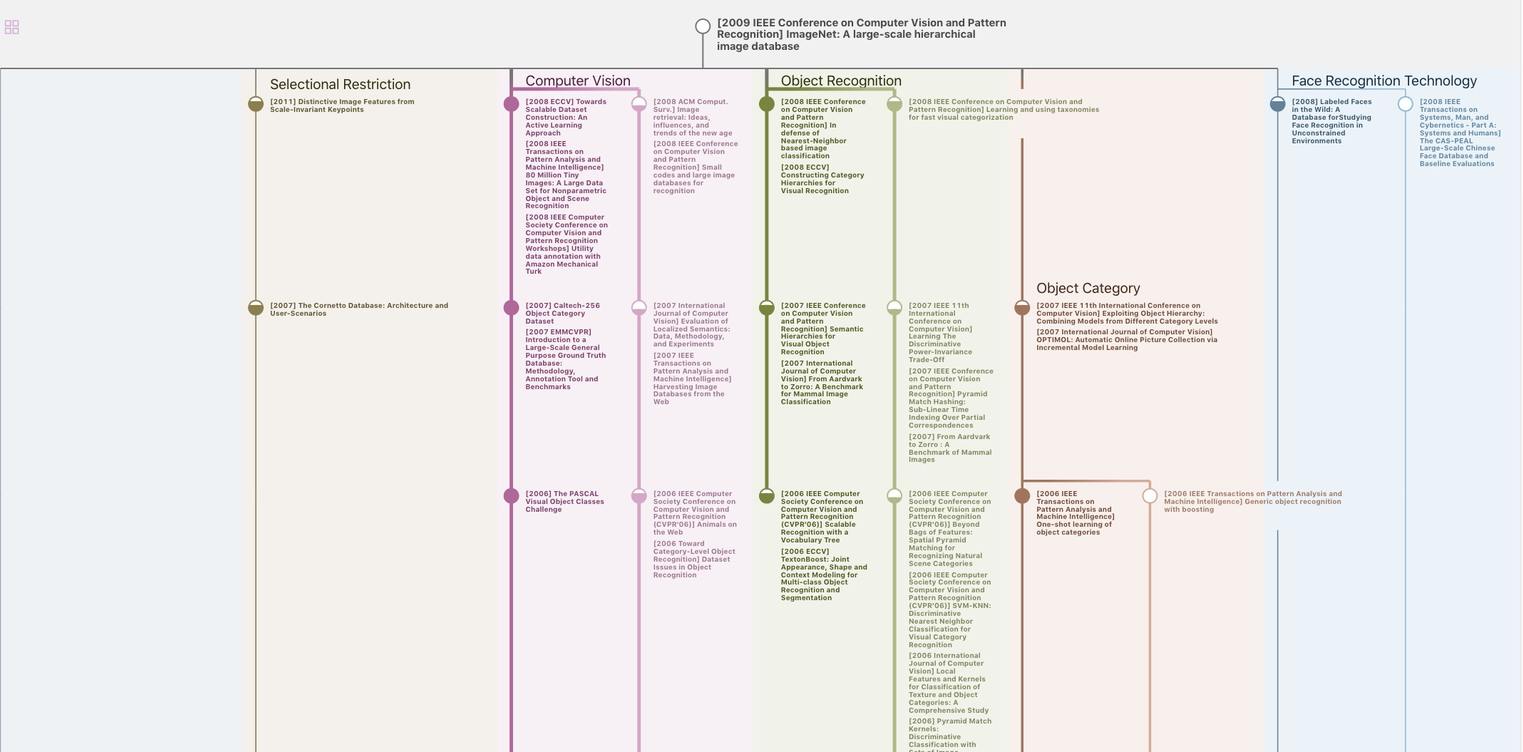
生成溯源树,研究论文发展脉络
Chat Paper
正在生成论文摘要