Effect of Weight Adjustment in Virtual Co-embodiment During Collaborative Training
AHs '23: Proceedings of the Augmented Humans International Conference 2023(2023)
摘要
Acquisition of motor skills plays an essential role in various contexts, such as sports, factory jobs, and nursing. For effective motor skill learning, "virtual co-embodiment" has been proposed as a novel virtual reality (VR) based method in which a virtual avatar is controlled based on the weighted average of the learner's and teacher's movements. Using virtual co-embodiment, a learner can learn the motor intention because they can feel a strong sense of agency in the avatar's movements modified by the teacher. However, after the assistance of the virtual co-embodiment vanishes, there is a performance drop problem; the learner cannot move as they learned, even if they understand the correct movement or motor intention, because the difference in body positions between the co-embodied avatar and the learner requires the latter to move differently after termination of the assistance. One way to match their positions is to increase the weight assigned to the learner's weight. However, simply assigning the learner a high weight does not allow the teacher to correct the avatar's movements and convey the correct movement and motor intention. By allowing the teacher a greater influence in the early stages of learning, and decreasing the influence as the learning progresses, it is expected to gradually allow the student to learn to operate independently. Therefore, we propose a method to prevent performance drop by adjusting weights according to the learning performance, thereby maintaining a high learning efficiency and helping advanced learners learn to independently demonstrate their abilities. In this study, we experimented with dual task learning to evaluate the automation of movement, which is considered an essential element of motor skills. We compared the performance drop when the virtual co-embodiment assist was terminated with static or adjusted weights based on the performance. Consequently, although the learning efficiency was slightly lower, the use of adjusted weights resulted in a significantly smaller performance drop after the termination of virtual co-embodiment assistance than that after the use of static weight.
更多查看译文
关键词
Virtual Co-embodiment, Motor Skill Learning, Collaborative Training, Adjustment
AI 理解论文
溯源树
样例
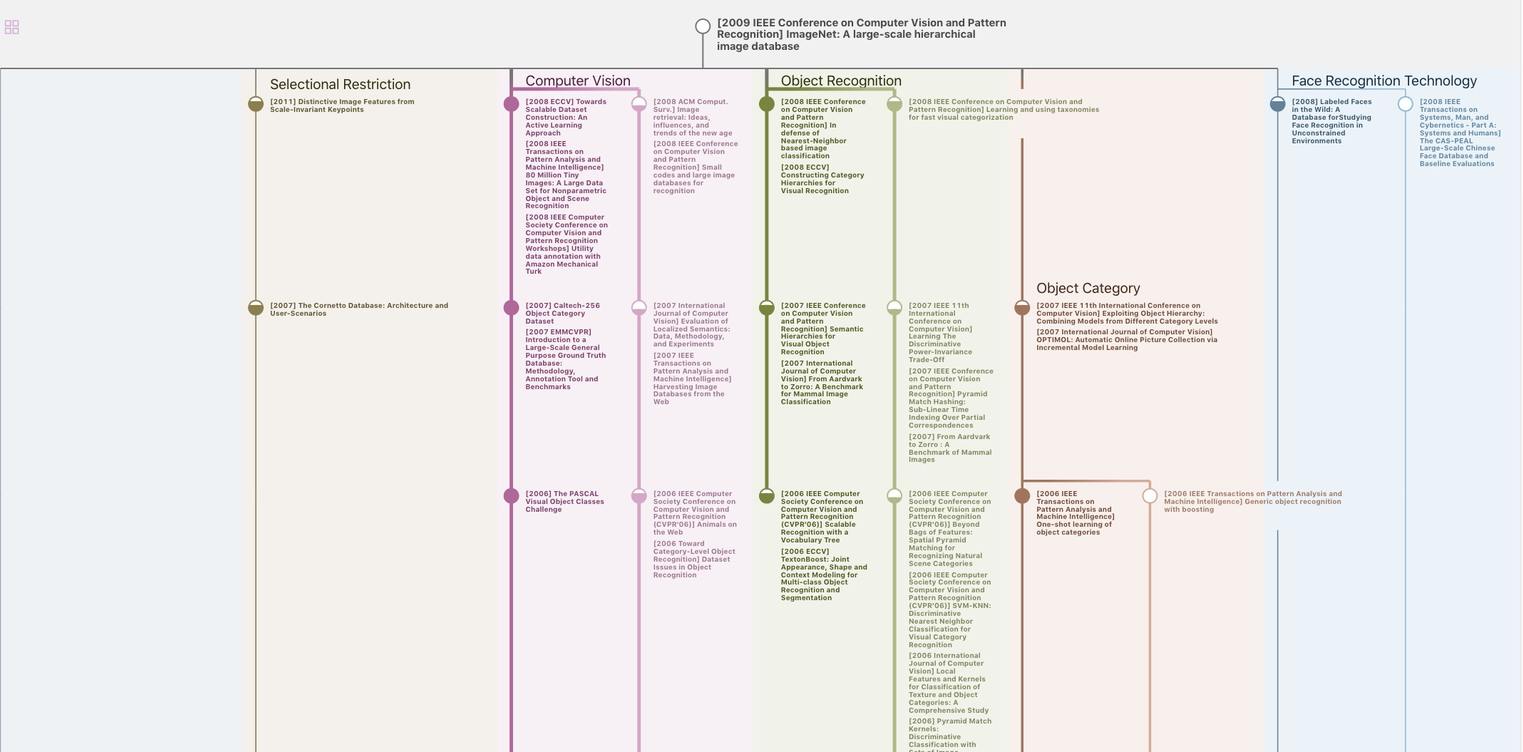
生成溯源树,研究论文发展脉络
Chat Paper
正在生成论文摘要