Real-time Context-Aware Multimodal Network for Activity and Activity-Stage Recognition from Team Communication in Dynamic Clinical Settings
Proceedings of the ACM on Interactive, Mobile, Wearable and Ubiquitous Technologies(2023)
摘要
In clinical settings, most automatic recognition systems use visual or sensory data to recognize activities. These systems cannot recognize activities that rely on verbal assessment, lack visual cues, or do not use medical devices. We examined speech-based activity and activity-stage recognition in a clinical domain, making the following contributions. (1) We collected a high-quality dataset representing common activities and activity stages during actual trauma resuscitation events-the initial evaluation and treatment of critically injured patients. (2) We introduced a novel multimodal network based on audio signal and a set of keywords that does not require a high-performing automatic speech recognition (ASR) engine. (3) We designed novel contextual modules to capture dynamic dependencies in team conversations about activities and stages during a complex workflow. (4) We introduced a data augmentation method, which simulates team communication by combining selected utterances and their audio clips, and showed that this method contributed to performance improvement in our data-limited scenario. In offline experiments, our proposed context-aware multimodal model achieved F1-scores of 73.2±0.8% and 78.1±1.1% for activity and activity-stage recognition, respectively. In online experiments, the performance declined about 10% for both recognition types when using utterance-level segmentation of the ASR output. The performance declined about 15% when we omitted the utterance-level segmentation. Our experiments showed the feasibility of speech-based activity and activity-stage recognition during dynamic clinical events.
更多查看译文
关键词
activity recognition,activity-stage recognition,context-aware recognition,keyword spotting,real-time application
AI 理解论文
溯源树
样例
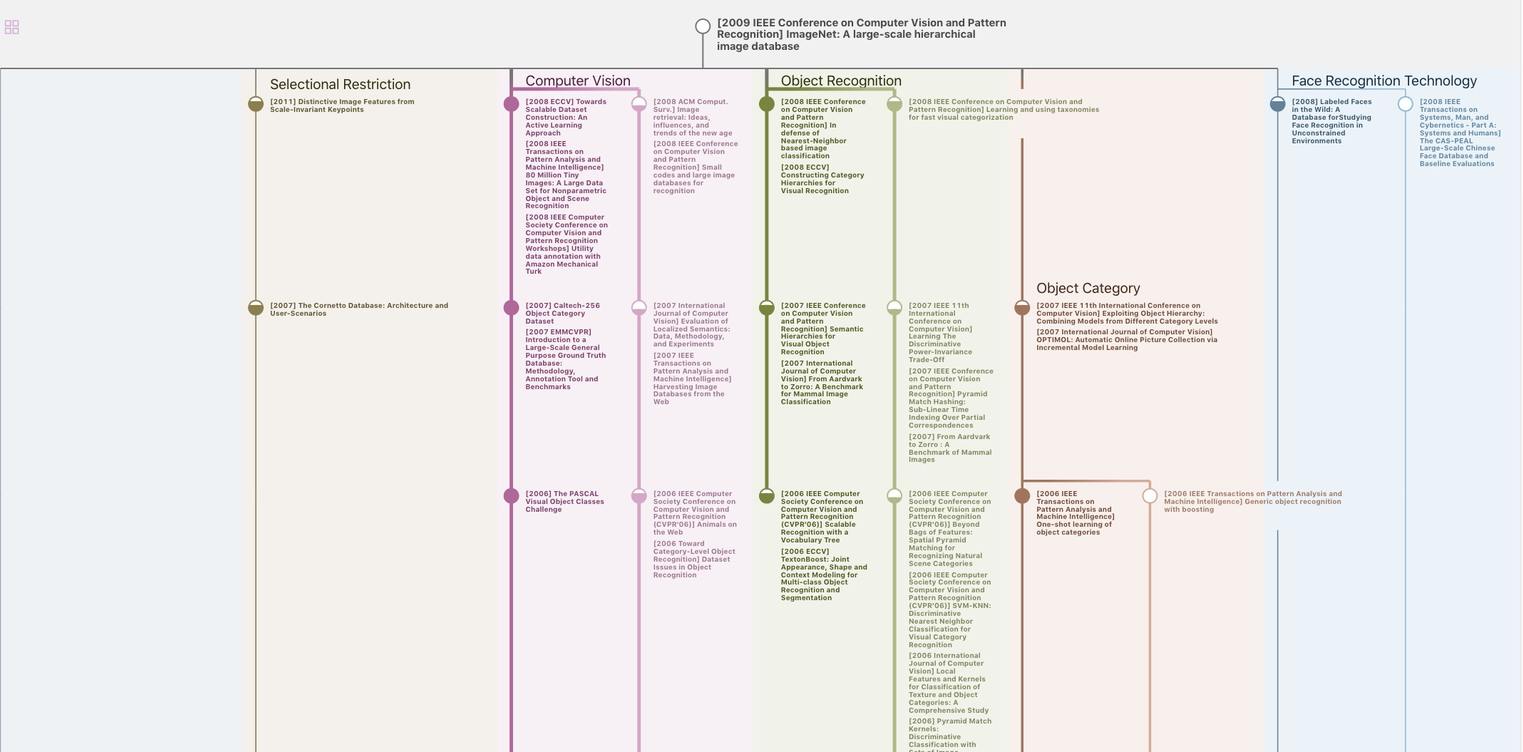
生成溯源树,研究论文发展脉络
Chat Paper
正在生成论文摘要