Dynamic Convolution-based Encoder-Decoder Framework for Image Captioning in Hindi
ACM Transactions on Asian and Low-Resource Language Information Processing(2023)
摘要
In sequence-to-sequence modeling tasks, such as image captioning, machine translation, and visual question answering, encoder-decoder architectures are state of the art. An encoder, convolutional neural network (CNN) encodes input images into fixed dimensional vector representation in the image captioning task, whereas a decoder, a recurrent neural network, performs language modeling and generates the target descriptions. Recent CNNs use the same operation over every pixel; however, all the image pixels are not equally important. To address this, the proposed method uses a dynamic convolution-based encoder for image encoding or feature extraction, Long-Short-Term-Memory as a decoder for language modeling, and X-Linear attention to make the system robust. Encoders, attentions, and decoders are important aspects of the image captioning task; therefore, we experiment with various encoders, decoders, and attention mechanisms. Most of the works for image captioning have been carried out for the English language in the existing literature. We propose a novel approach for caption generation from images in Hindi. Hindi, widely spoken in South Asia and India, is the fourth most-spoken language globally; it is India’s official language. The proposed method utilizes dynamic convolution operation on the encoder side to obtain a better image encoding quality. The Hindi image captioning dataset is manually created by translating the popular MSCOCO dataset from English to Hindi. In terms of BLEU scores, the performance of the proposed method is compared with other baselines, and the results obtained show that the proposed method outperforms different baselines. Manual human assessment in terms of adequacy and fluency of the captions generated further determines the efficacy of the proposed method in generating good-quality captions.
更多查看译文
关键词
Hindi,dynamic convolution,attention,deep learning
AI 理解论文
溯源树
样例
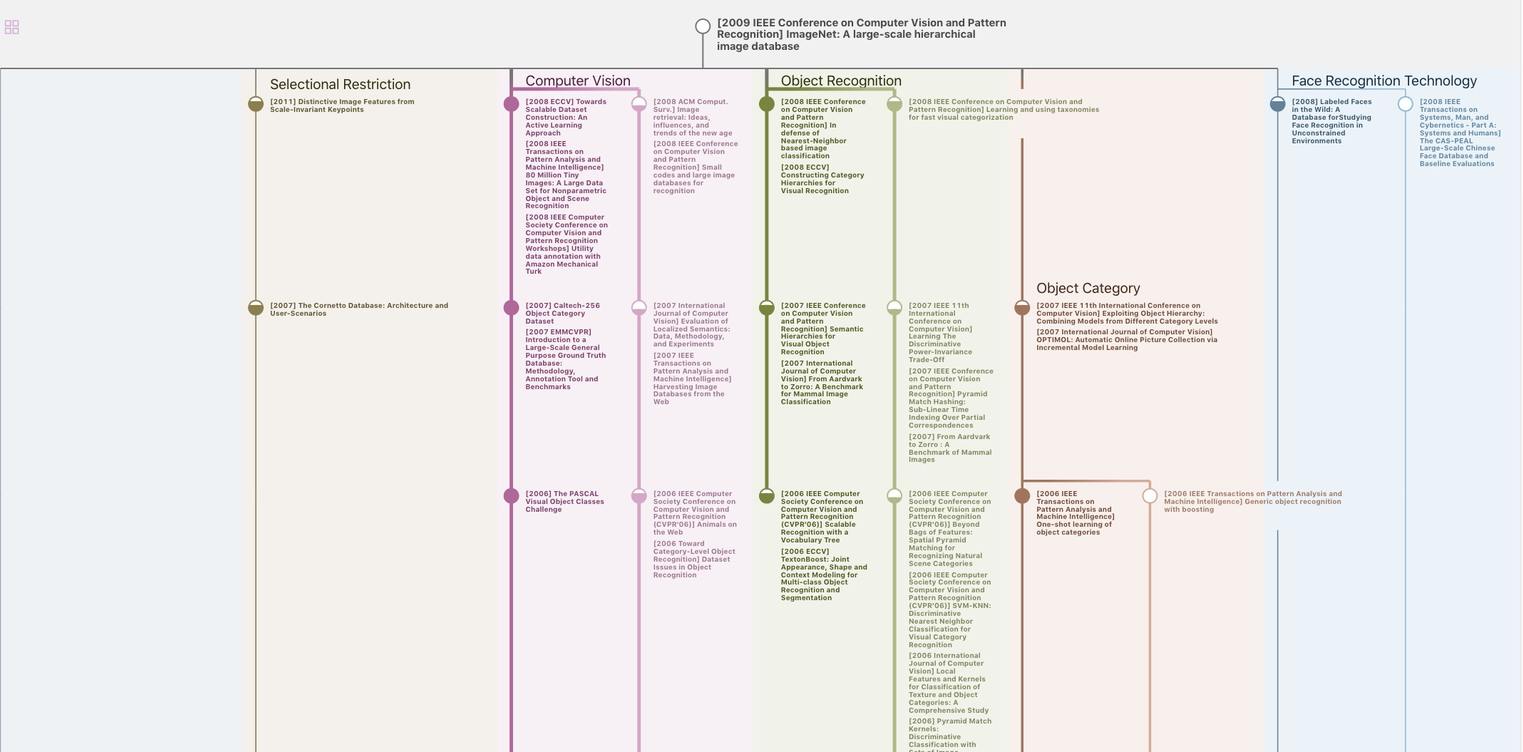
生成溯源树,研究论文发展脉络
Chat Paper
正在生成论文摘要