Beyond Empirical Risk Minimization: Local Structure Preserving Regularization for Improving Adversarial Robustness
CoRR(2023)
摘要
It is broadly known that deep neural networks are susceptible to being fooled by adversarial examples with perturbations imperceptible by humans. Various defenses have been proposed to improve adversarial robustness, among which adversarial training methods are most effective. However, most of these methods treat the training samples independently and demand a tremendous amount of samples to train a robust network, while ignoring the latent structural information among these samples. In this work, we propose a novel Local Structure Preserving (LSP) regularization, which aims to preserve the local structure of the input space in the learned embedding space. In this manner, the attacking effect of adversarial samples lying in the vicinity of clean samples can be alleviated. We show strong empirical evidence that with or without adversarial training, our method consistently improves the performance of adversarial robustness on several image classification datasets compared to the baselines and some state-of-the-art approaches, thus providing promising direction for future research.
更多查看译文
关键词
adversarial robustness,regularization,empirical risk minimization,local structure preserving
AI 理解论文
溯源树
样例
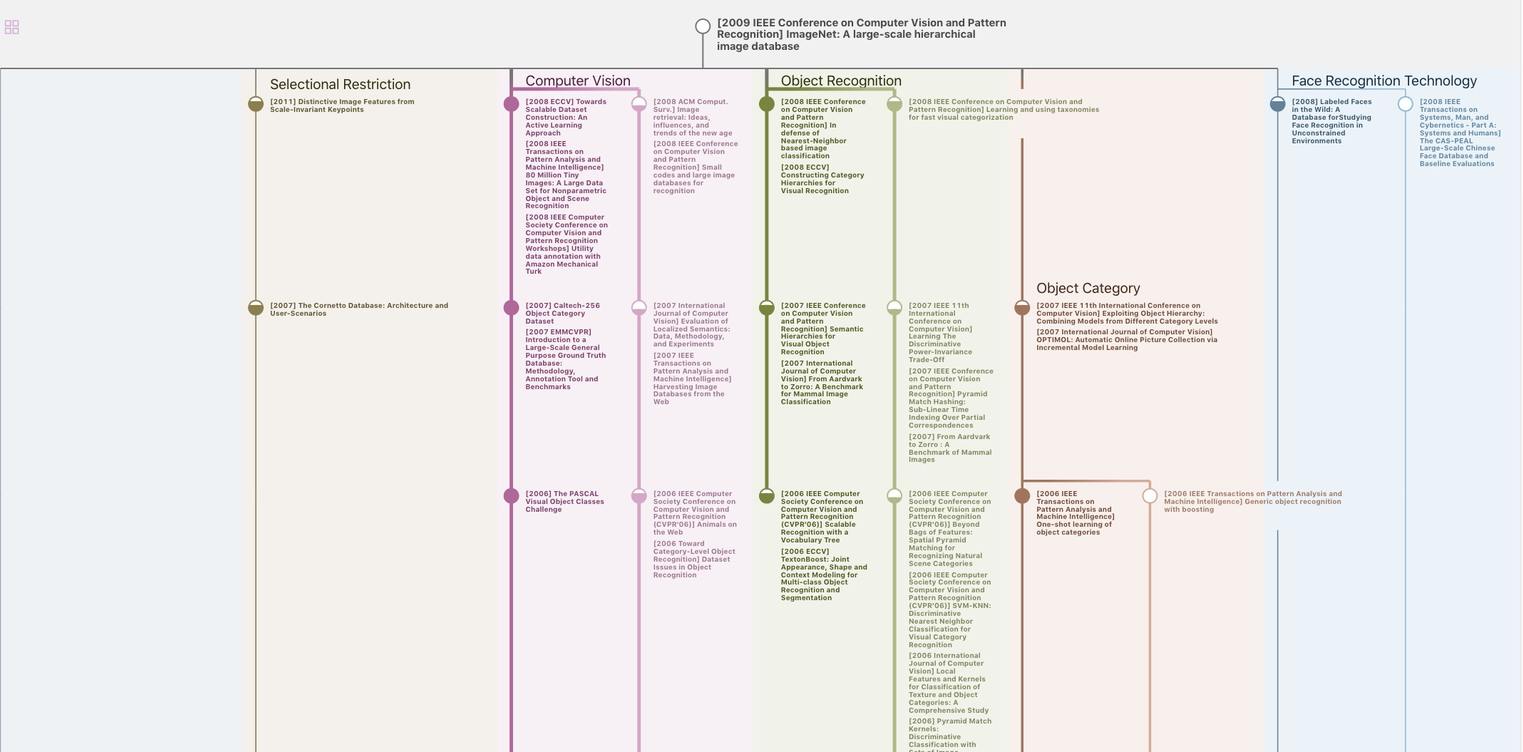
生成溯源树,研究论文发展脉络
Chat Paper
正在生成论文摘要