Correcting for Selection Bias and Missing Response in Regression using Privileged Information
UAI(2023)
摘要
When estimating a regression model, we might have data where some labels are missing, or our data might be biased by a selection mechanism. When the response or selection mechanism is ignorable (i.e., independent of the response variable given the features) one can use off-the-shelf regression methods; in the nonignorable case one typically has to adjust for bias. We observe that privileged data (i.e. data that is only available during training) might render a nonignorable selection mechanism ignorable, and we refer to this scenario as Privilegedly Missing at Random (PMAR). We propose a novel imputation-based regression method, named repeated regression, that is suitable for PMAR. We also consider an importance weighted regression method, and a doubly robust combination of the two. The proposed methods are easy to implement with most popular out-of-the-box regression algorithms. We empirically assess the performance of the proposed methods with extensive simulated experiments and on a synthetically augmented real-world dataset. We conclude that repeated regression can appropriately correct for bias, and can have considerable advantage over weighted regression, especially when extrapolating to regions of the feature space where response is never observed.
更多查看译文
关键词
selection bias,regression,missing response
AI 理解论文
溯源树
样例
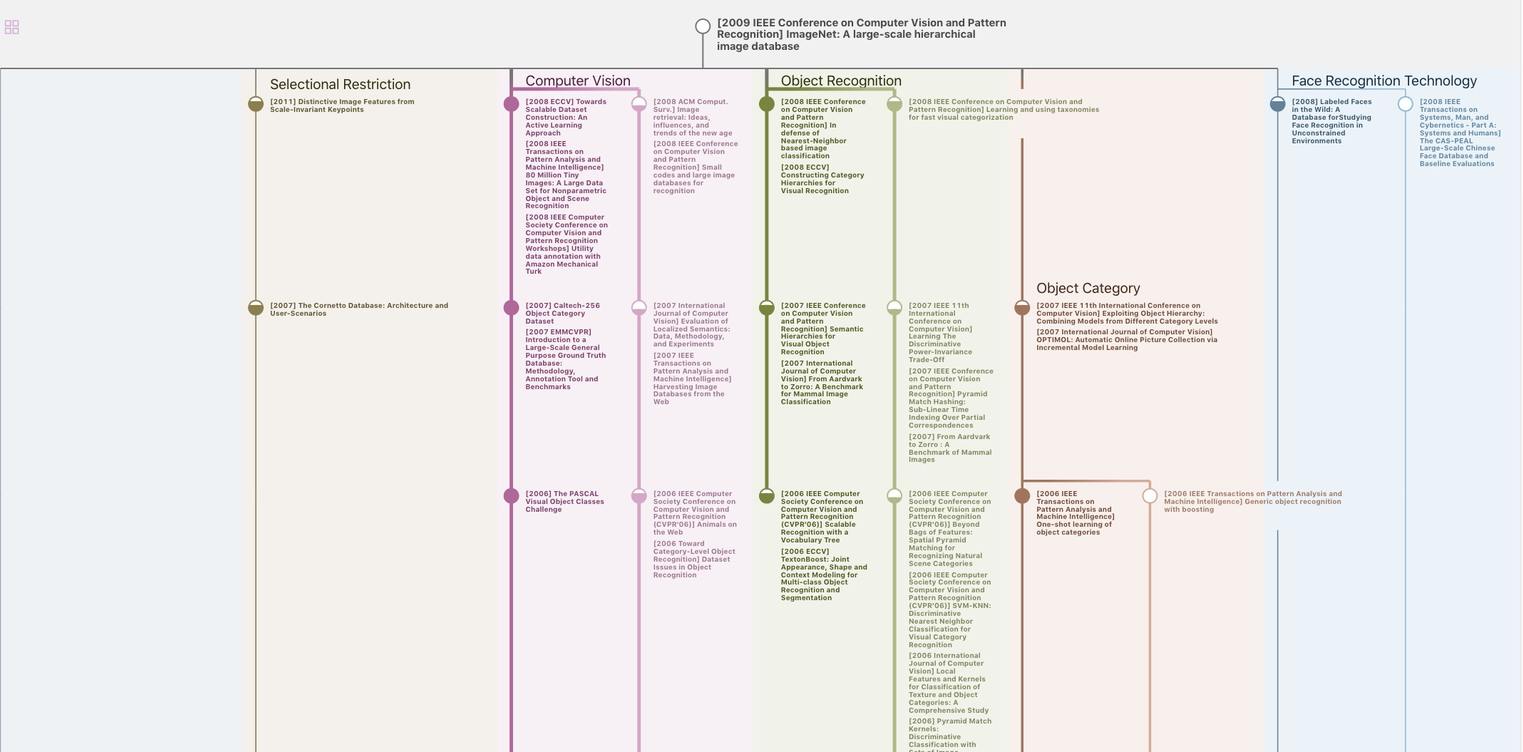
生成溯源树,研究论文发展脉络
Chat Paper
正在生成论文摘要