One-Bit Covariance Reconstruction with Non-zero Thresholds: Algorithm and Performance Analysis
arXiv (Cornell University)(2023)
摘要
Covariance matrix reconstruction is a topic of great significance in the field of one-bit signal processing and has numerous practical applications. Despite its importance, the conventional arcsine law with zero threshold is incapable of recovering the diagonal elements of the covariance matrix. To address this limitation, recent studies have proposed the use of non-zero clipping thresholds. However, the relationship between the estimation error and the sampling threshold is not yet known. In this paper, we undertake an analysis of the mean squared error by computing the Fisher information matrix for a given threshold. Our results reveal that the optimal threshold can vary considerably, depending on the variances and correlation coefficients. As a result, it is inappropriate to use a constant threshold to encompass parameters that vary widely. To mitigate this issue, we present a recovery scheme that incorporates time-varying thresholds. Our approach differs from existing methods in that it utilizes the exact values of the threshold, rather than its statistical properties, to enhance the estimation performance. Our simulations, including the direction-of-arrival estimation problem, demonstrate the efficacy of the developed scheme, especially in complex scenarios where the covariance elements are widely separated.
更多查看译文
关键词
thresholds,one-bit,non-zero
AI 理解论文
溯源树
样例
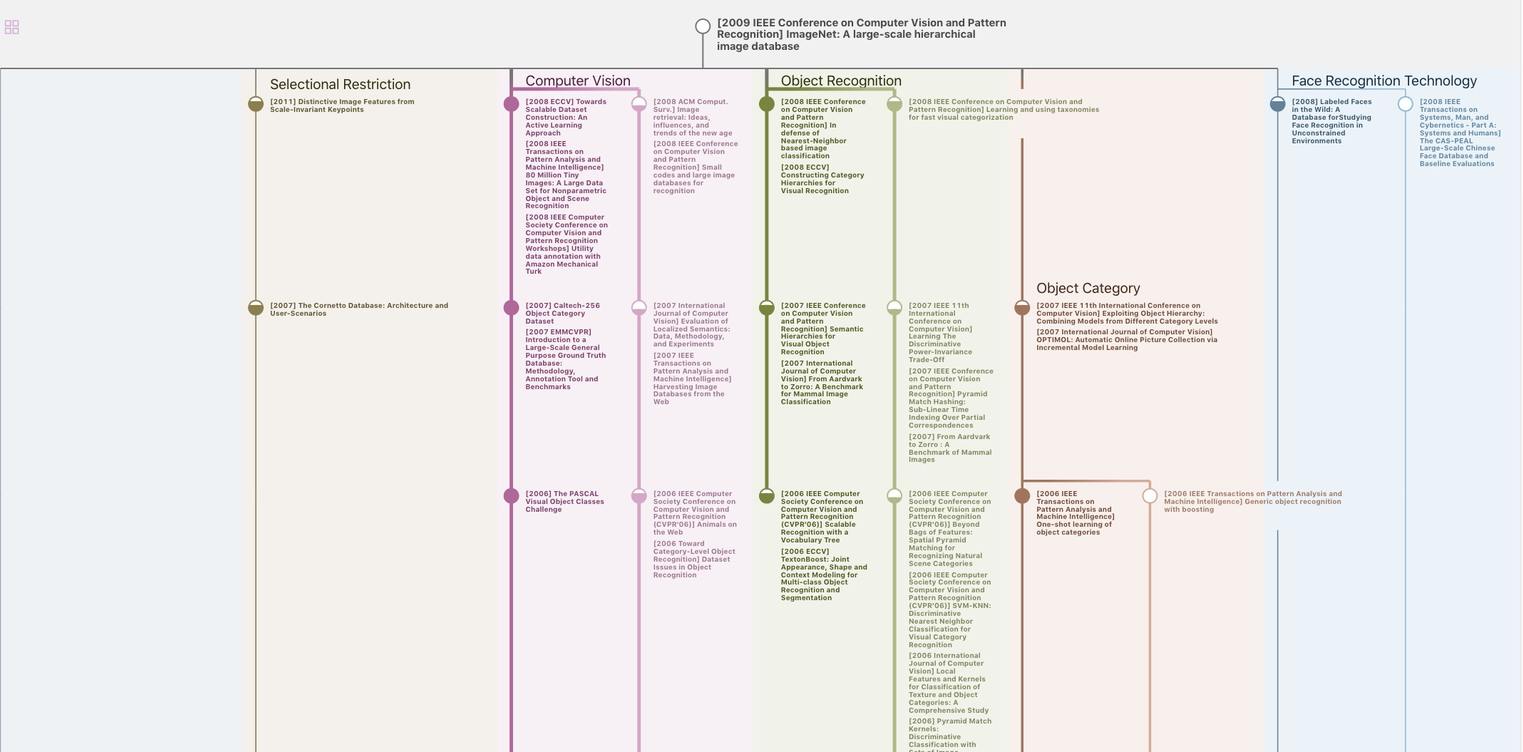
生成溯源树,研究论文发展脉络
Chat Paper
正在生成论文摘要