Structured Video-Language Modeling with Temporal Grouping and Spatial Grounding
ICLR 2024(2023)
摘要
Existing video-language pre-training methods primarily focus on
instance-level alignment between video clips and captions via global
contrastive learning but neglect rich fine-grained local information in both
videos and text, which is of importance to downstream tasks requiring temporal
localization and semantic reasoning. A powerful model is expected to be capable
of capturing region-object correspondences and recognizing scene changes in a
video clip, reflecting spatial and temporal granularity, respectively. To
strengthen model's understanding into such fine-grained details, we propose a
simple yet effective video-language modeling framework, S-ViLM, by exploiting
the intrinsic structures of these two modalities. It includes two novel
designs, inter-clip spatial grounding and intra-clip temporal grouping, to
promote learning region-object alignment and temporal-aware features,
simultaneously. Comprehensive evaluations demonstrate that S-ViLM performs
favorably against existing approaches in learning more expressive
representations. Specifically, S-ViLM surpasses the state-of-the-art methods
substantially on four representative downstream tasks, covering text-video
retrieval, video question answering, video action recognition, and temporal
action localization.
更多查看译文
关键词
multi-modal learning,video and language
AI 理解论文
溯源树
样例
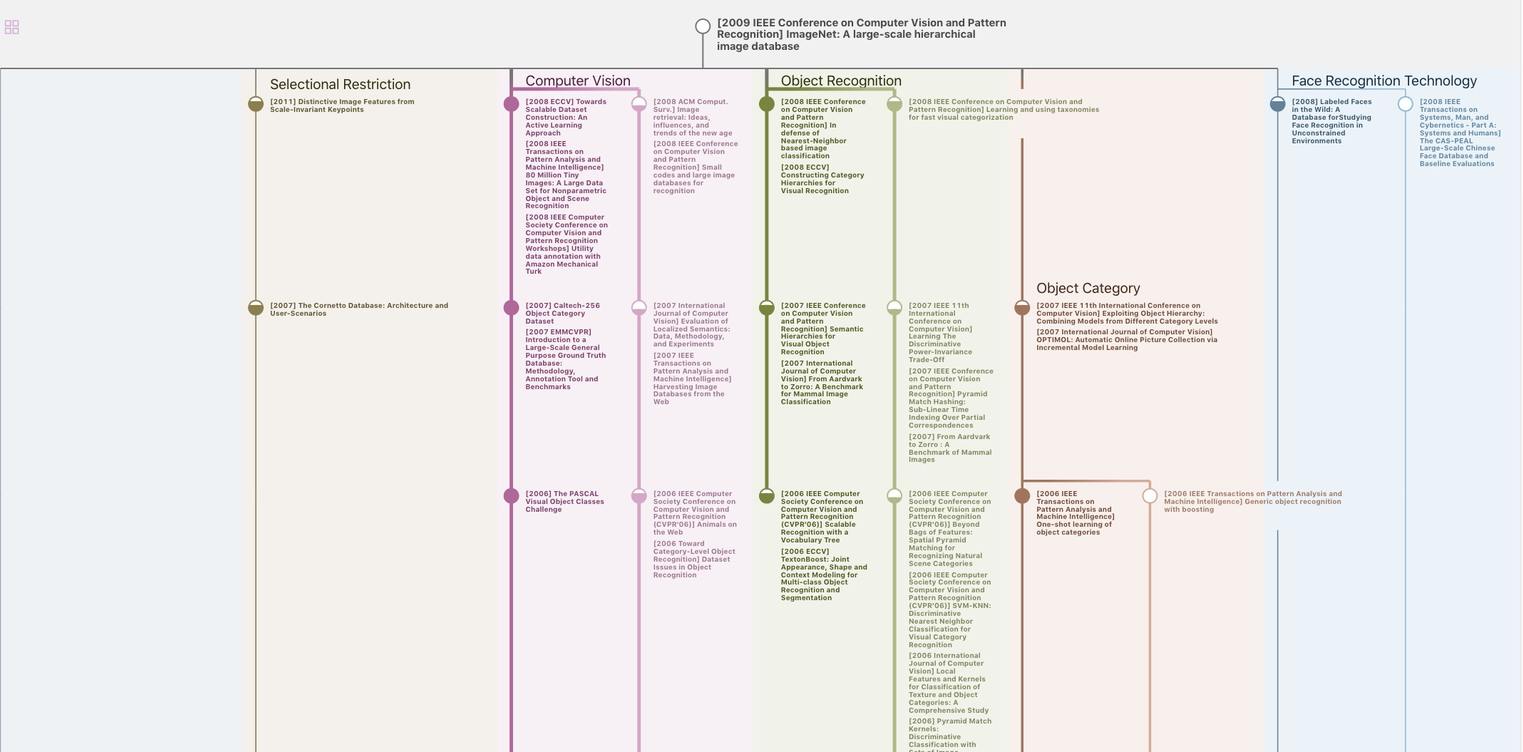
生成溯源树,研究论文发展脉络
Chat Paper
正在生成论文摘要