Multi-stage stacked temporal convolution neural networks (MS-S-TCNs) for biosignal segmentation and anomaly localization
Pattern Recognition(2023)
摘要
•We propose a novel deep learning architecture based on MS-TCN for 1D biosignal sequence-to-sequence prediction tasks which benefits from TCN modules with varying dilation factors and effective convolution based fusion.•To improve the predictive capability of the model we propose two novel loss functions: aiming smooth predictions, we propose a loss based on first-order derivative and aiming to solve class-imbalance, we propose a class-conditioned loss.•We demonstrate the universality of our proposed model over five tasks, and our proposed architecture significantly outperforms the state-of-the-art models, and also demonstrates significant performance gains compared to a vanilla MS-TCN formulation, while producing more refined predictions at the later stages.•Our architecture is interpretable. We use model interpretation to demonstrate the effectiveness of the components we used to formulate this architecture, in addition why it demonstrates better performance compared to recurrent neural networks.
更多查看译文
关键词
Deep learning,Electrocardiogram,Heart sounds,Lung sounds,Segmentation,Model interpretation
AI 理解论文
溯源树
样例
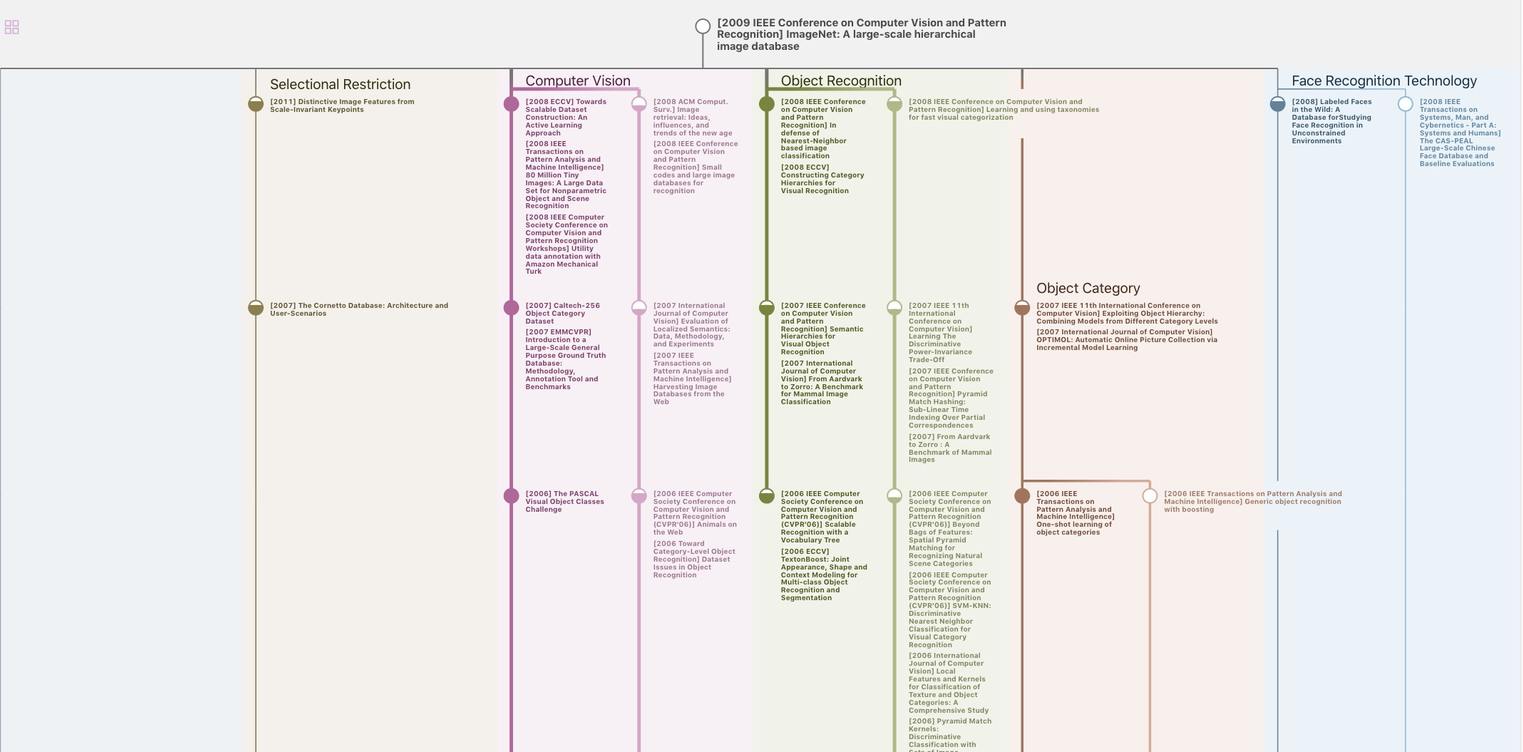
生成溯源树,研究论文发展脉络
Chat Paper
正在生成论文摘要