The transformative potential of machine learning for experiments in fluid mechanics
CoRR(2023)
摘要
The field of machine learning (ML) has rapidly advanced the state of the art in many fields of science and engineering, including experimental fluid dynamics, which is one of the original big-data disciplines. This Perspective article highlights several aspects of experimental fluid mechanics that stand to benefit from progress in ML, including augmenting the fidelity and quality of measurement techniques, improving experimental design and surrogate digital-twin models and enabling real-time estimation and control. In each case, we discuss recent success stories and ongoing challenges, along with caveats and limitations, and outline the potential for new avenues of ML-augmented and ML-enabled experimental fluid mechanics.
更多查看译文
关键词
machine learning,transformative potential,mechanics,fluid
AI 理解论文
溯源树
样例
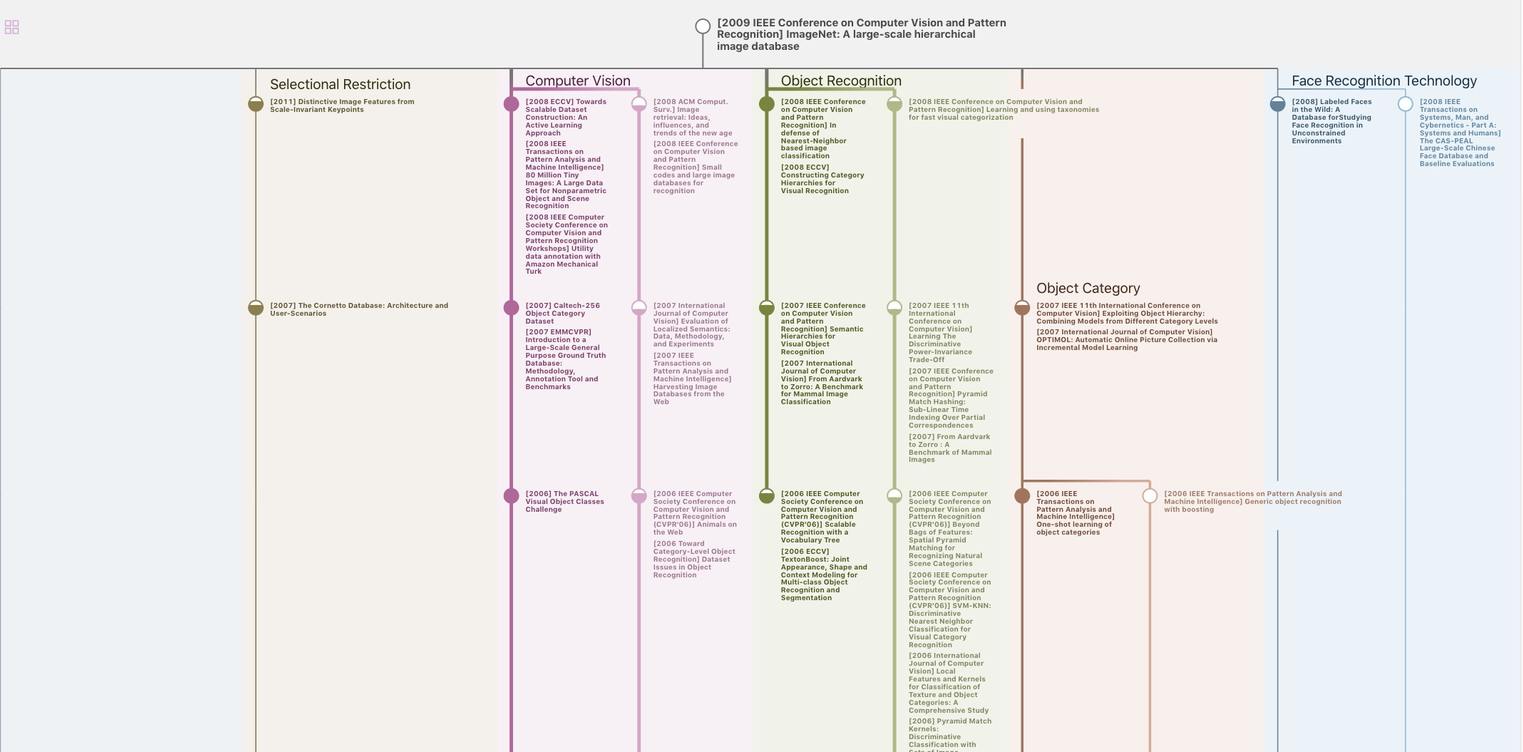
生成溯源树,研究论文发展脉络
Chat Paper
正在生成论文摘要