Pre-training Transformers for Knowledge Graph Completion
CoRR(2023)
摘要
Learning transferable representation of knowledge graphs (KGs) is challenging due to the heterogeneous, multi-relational nature of graph structures. Inspired by Transformer-based pretrained language models' success on learning transferable representation for texts, we introduce a novel inductive KG representation model (iHT) for KG completion by large-scale pre-training. iHT consists of a entity encoder (e.g., BERT) and a neighbor-aware relational scoring function both parameterized by Transformers. We first pre-train iHT on a large KG dataset, Wikidata5M. Our approach achieves new state-of-the-art results on matched evaluations, with a relative improvement of more than 25% in mean reciprocal rank over previous SOTA models. When further fine-tuned on smaller KGs with either entity and relational shifts, pre-trained iHT representations are shown to be transferable, significantly improving the performance on FB15K-237 and WN18RR.
更多查看译文
关键词
knowledge graph completion,knowledge graph,pre-training
AI 理解论文
溯源树
样例
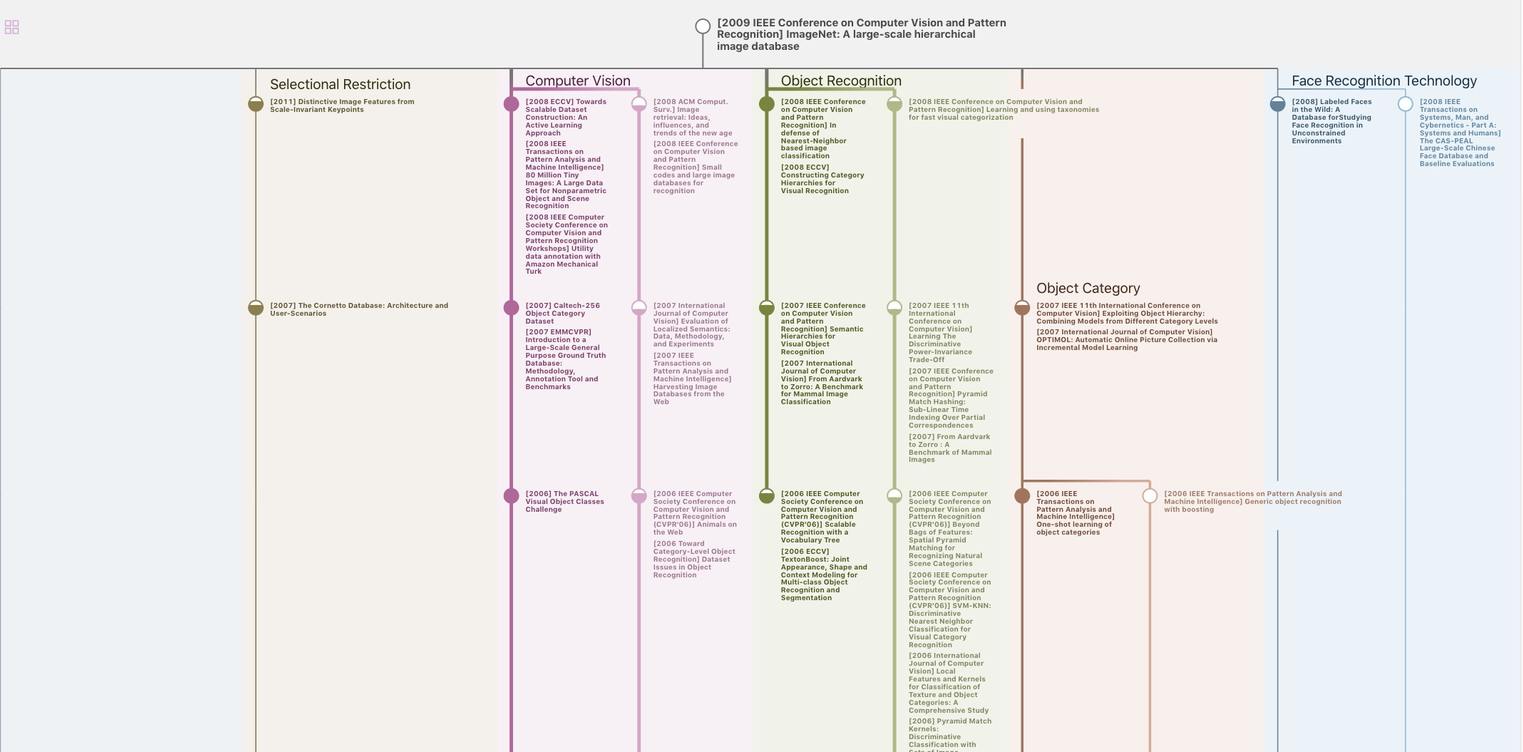
生成溯源树,研究论文发展脉络
Chat Paper
正在生成论文摘要