A New Approach to Traffic Accident Anticipation With Geometric Features for Better Generalizability
IEEE Access(2023)
摘要
Traffic accident anticipation is essential for successful autonomous and assistive driving systems. Existing accident anticipation algorithms that mostly rely on visual features of the accident related objects involved provides both high AP (Average Precision) and TTA (Time to Accident). Despite a spatiotemporal relationship with the visual features of the accident related objects involved, these methods are often biased and therefore not well generalizable. In this paper, firstly we discuss dataset biases and then show that those high AP and TTA results came mainly from visual biases. Secondly, to overcome some of the visual biases, we propose a novel deep learning framework that uses both visual and geometric information of the accident-related objects captured in dash cam videos. Thirdly, we show effectiveness of the proposed method in terms of generalization capability compared to existing approaches with several open datasets from actual accident videos.
更多查看译文
关键词
Computer vision,dataset bias,deep learning,traffic accident anticipation
AI 理解论文
溯源树
样例
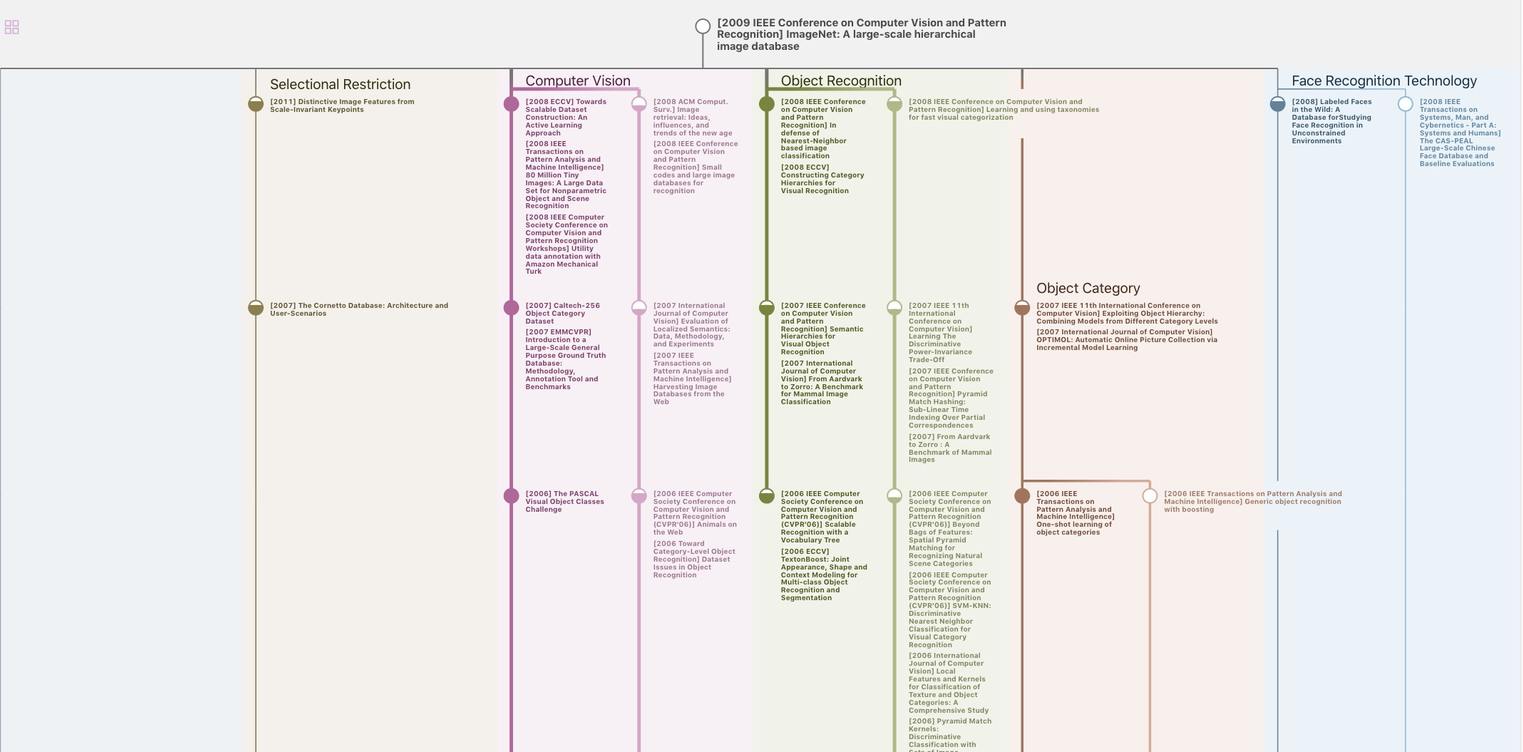
生成溯源树,研究论文发展脉络
Chat Paper
正在生成论文摘要