Synthetic data generation: State of the art in health care domain
Computer Science Review(2023)
摘要
Recent progress in artificial intelligence and machine learning has led to the growth of research in every aspect of life including the health care domain. However, privacy risks and legislations hinder the availability of patient data to researchers. Synthetic data (SD) has been regarded as a privacy-safe alternative to real data and has lately been employed in many research and academic endeavors. This growing body of research needs to be consolidated for the researchers and practitioners to gain a quick and fruitful comprehension of the state of the art in synthetic data generation in health care. The purpose of this study is to collate and synthesize the current state of synthetic data generation following a narrative review of 70 peer-reviewed studies discussing privacy-preserving synthetic medical data generation techniques. The literature shows the effectiveness of synthetic datasets for different applications in research, academics, and testing according to existing statistical and task-based utility metrics. However, the focus on longitudinal synthetic data seems deficient. Moreover, a unified metric for generic quality assessment of synthetic data is lacking. The results of this review will serve as a quick reference guide for the researchers and practitioners in the healthcare domain to select a suitable synthetic data strategy for their application based on its strengths and weaknesses and pave the path for further research and development in healthcare.(c) 2023 Elsevier Inc. All rights reserved.
更多查看译文
关键词
Synthetic data,Health informatics,Data privacy,Privacy preserving data publishing,Medical informatics,Generative adversarial networks,Electronic health records
AI 理解论文
溯源树
样例
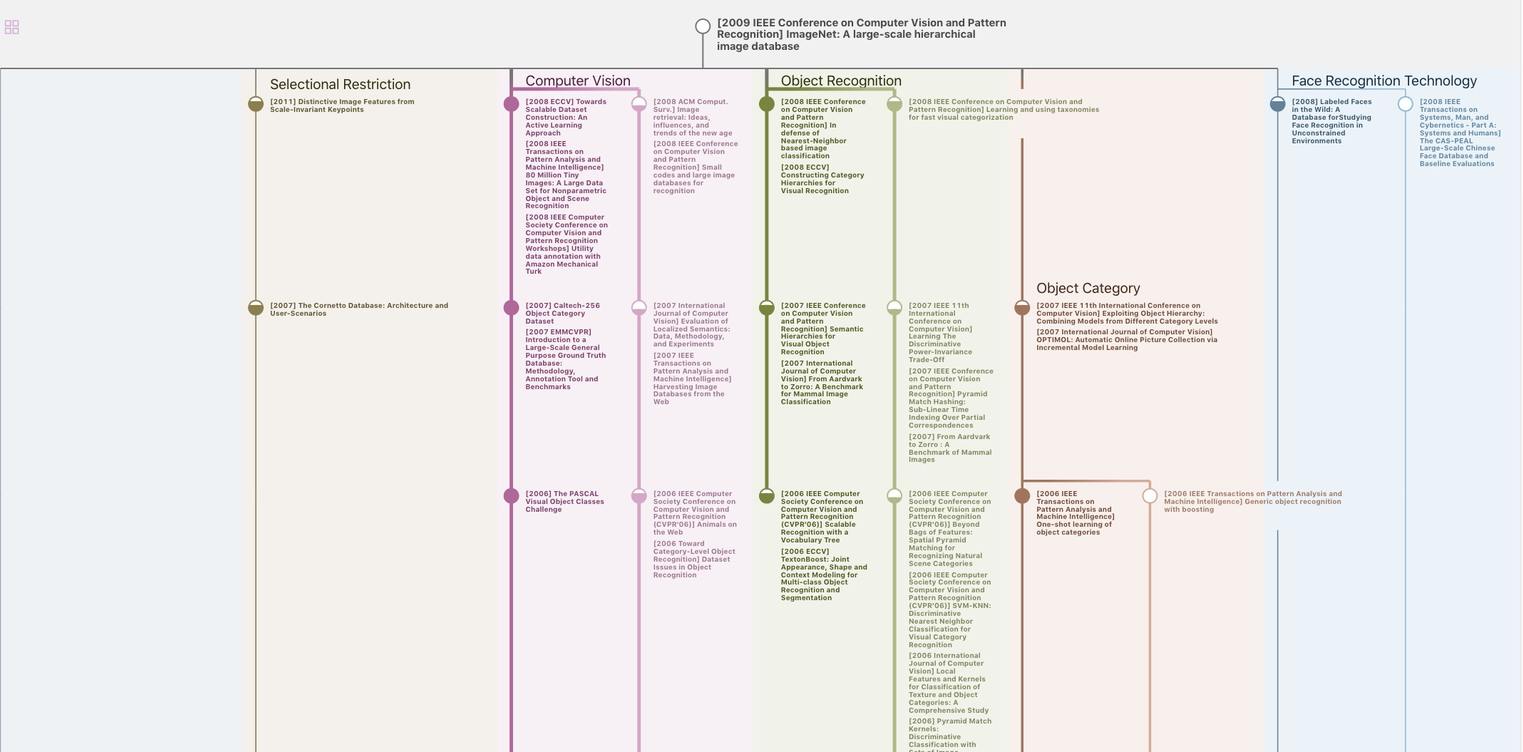
生成溯源树,研究论文发展脉络
Chat Paper
正在生成论文摘要