TPBTE: A model based on convolutional Transformer for predicting the binding of TCR to epitope.
Molecular immunology(2023)
摘要
T cell receptors (TCRs) selectively bind to antigens to fight pathogens with specific immunity. Current tools focus on the nature of amino acids within sequences and take less into account the nature of amino acids far apart and the relationship between sequences, leading to significant differences in the results from different datasets. We propose TPBTE, a model based on convolutional Transformer for Predicting the Binding of TCR to Epitope. It takes epitope sequences and the complementary decision region 3 (CDR3) sequences of TCRβ chain as inputs. And it uses a convolutional attention mechanism to learn amino acid representations between different positions of the sequences based on learning local features of the sequences. At the same time, it uses cross attention to learn the interaction information between TCR sequences and epitope sequences. A comprehensive evaluation of the TCR-epitope data shows that the average area under the curve of TPBTE outperforms the baseline model, and demonstrate an intentional performance. In addition, TPBTE can give the probability of binding TCR to epitopes, which can be used as the first step of epitope screening, narrowing the scope of epitope search and reducing the time of epitope search.
更多查看译文
关键词
Antigenic epitopes,Attention mechanism,T cell Epitope prediction,T cell receptor,Transformer
AI 理解论文
溯源树
样例
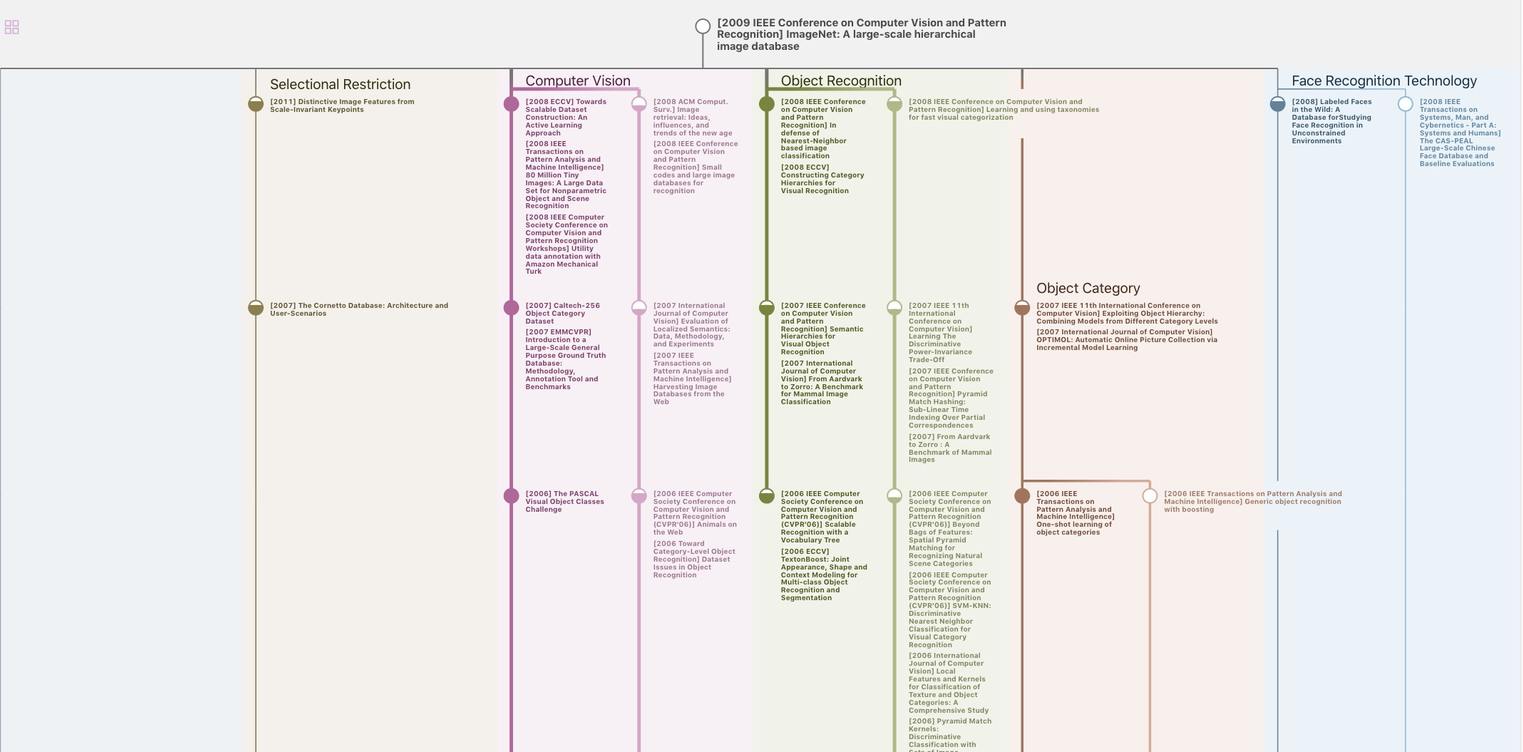
生成溯源树,研究论文发展脉络
Chat Paper
正在生成论文摘要