Self-adaptive fusion of local-temporal features for tool condition monitoring: A human experience free model
Mechanical Systems and Signal Processing(2023)
摘要
Cutting tool condition monitoring (TCM) techniques optimize production cost and machining quality, which is recognized as an important enabling technology for smart manufacturing. Un-like the existing monitoring algorithms, which depend on manual operation and domain knowledge in implementation, this paper proposes an autonomous TCM model without human experience based on the automatic selection and fusion of local-temporal features. In the pro-posed self-adaptive fusion model, the local features are automatically selected and fused by the adaptive fusion of multi-domain features according to their performance. Temporal features can be extracted autonomously by a heterogeneous feature fusion model with the help of a con-volutional block attention module. To address the empirical dependence in feature fusion, a method for pre-evaluation of fused features is proposed by calculating the fusion performance indexes, which provides a reliable basis for the selection of dimension reduction methods. Compared with existing fusion methods, the proposed model not only enables the classification of the extracted features but also facilitates the regression and monitoring of specific values of tool wear. The performance of the fused features by self-adaptive fusion is improved by 9.48% on average compared to the best-performing conventional fusion method. Milling experiments show that the proposed model can be robustly adapted to different cutting conditions and achieve optimal performance with minimal computational resources, which provides a theoretical reference for the development of standardized and autonomous TCM.
更多查看译文
关键词
Feature fusion,Automatic feature extraction,Tool wear monitoring,Local feature,Attention mechanism
AI 理解论文
溯源树
样例
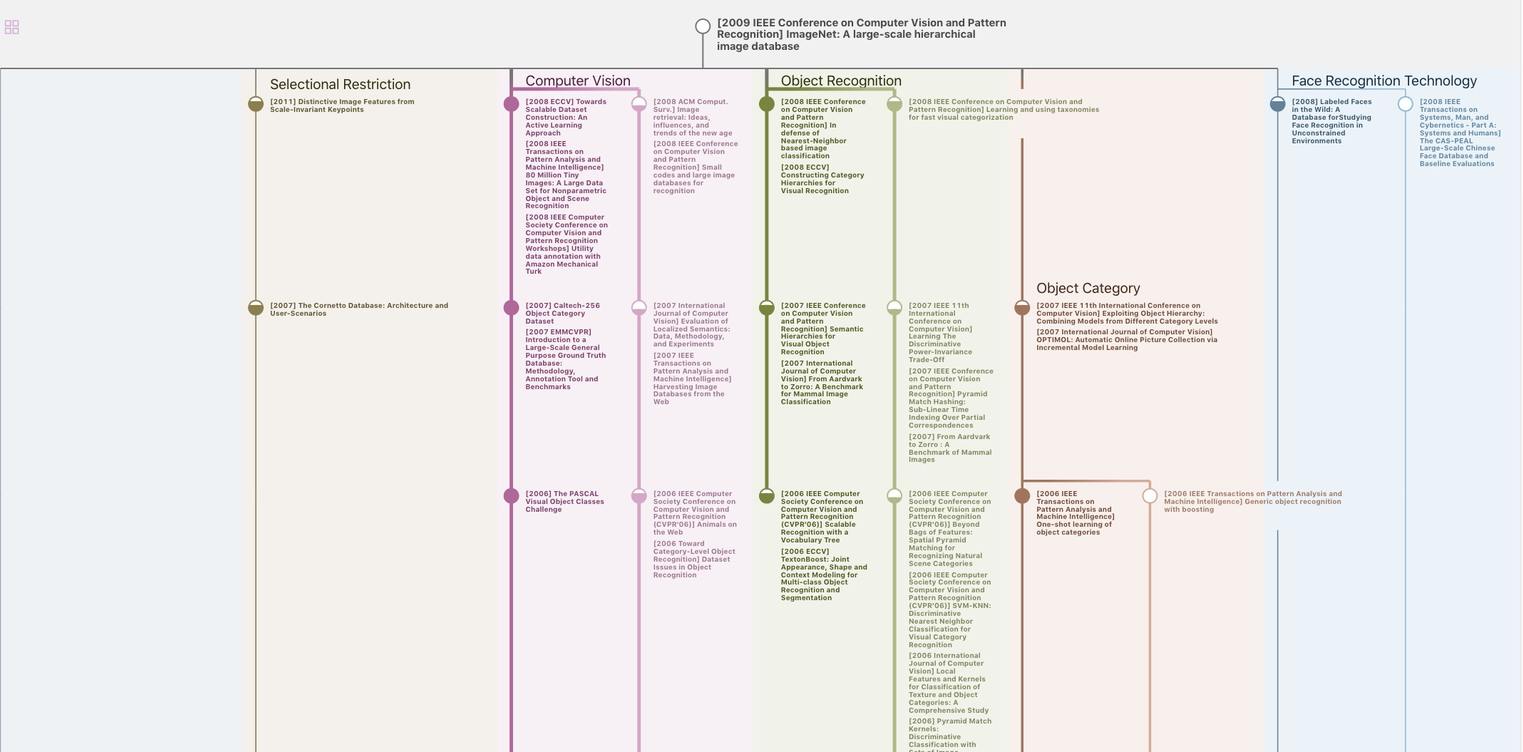
生成溯源树,研究论文发展脉络
Chat Paper
正在生成论文摘要