Causal schema induction for knowledge discovery
CoRR(2023)
摘要
Making sense of familiar yet new situations typically involves making generalizations about causal schemas, stories that help humans reason about event sequences. Reasoning about events includes identifying cause and effect relations shared across event instances, a process we refer to as causal schema induction. Statistical schema induction systems may leverage structural knowledge encoded in discourse or the causal graphs associated with event meaning, however resources to study such causal structure are few in number and limited in size. In this work, we investigate how to apply schema induction models to the task of knowledge discovery for enhanced search of English-language news texts. To tackle the problem of data scarcity, we present Torquestra, a manually curated dataset of text-graph-schema units integrating temporal, event, and causal structures. We benchmark our dataset on three knowledge discovery tasks, building and evaluating models for each. Results show that systems that harness causal structure are effective at identifying texts sharing similar causal meaning components rather than relying on lexical cues alone. We make our dataset and models available for research purposes.
更多查看译文
关键词
causal schema induction,discovery,knowledge
AI 理解论文
溯源树
样例
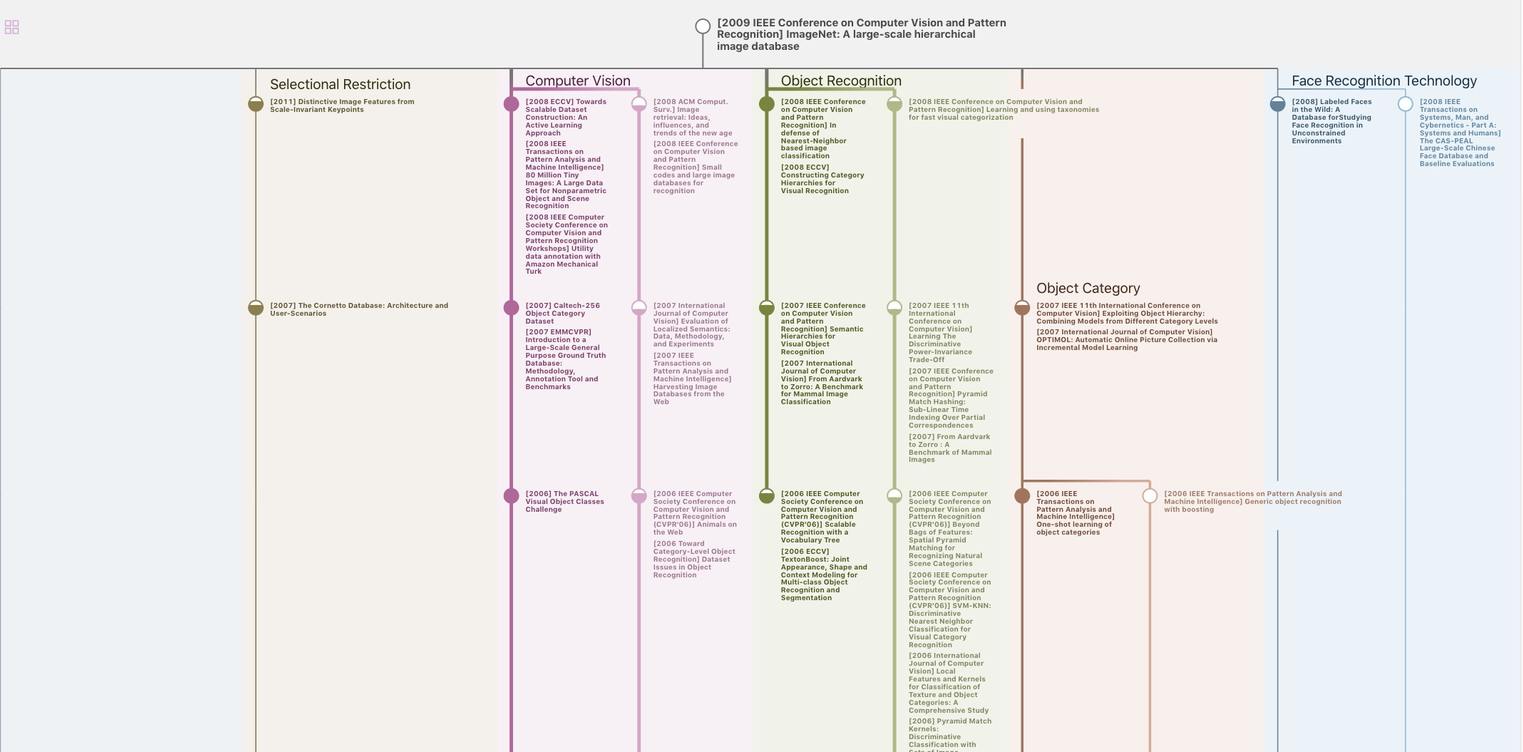
生成溯源树,研究论文发展脉络
Chat Paper
正在生成论文摘要