Knowing the Distance: Understanding the Gap Between Synthetic and Real Data For Face Parsing
CoRR(2023)
摘要
The use of synthetic data for training computer vision algorithms has become increasingly popular due to its cost-effectiveness, scalability, and ability to provide accurate multi-modality labels. Although recent studies have demonstrated impressive results when training networks solely on synthetic data, there remains a performance gap between synthetic and real data that is commonly attributed to lack of photorealism. The aim of this study is to investigate the gap in greater detail for the face parsing task. We differentiate between three types of gaps: distribution gap, label gap, and photorealism gap. Our findings show that the distribution gap is the largest contributor to the performance gap, accounting for over 50% of the gap. By addressing this gap and accounting for the labels gap, we demonstrate that a model trained on synthetic data achieves comparable results to one trained on a similar amount of real data. This suggests that synthetic data is a viable alternative to real data, especially when real data is limited or difficult to obtain. Our study highlights the importance of content diversity in synthetic datasets and challenges the notion that the photorealism gap is the most critical factor affecting the performance of computer vision models trained on synthetic data.
更多查看译文
关键词
face,synthetic,data,understanding
AI 理解论文
溯源树
样例
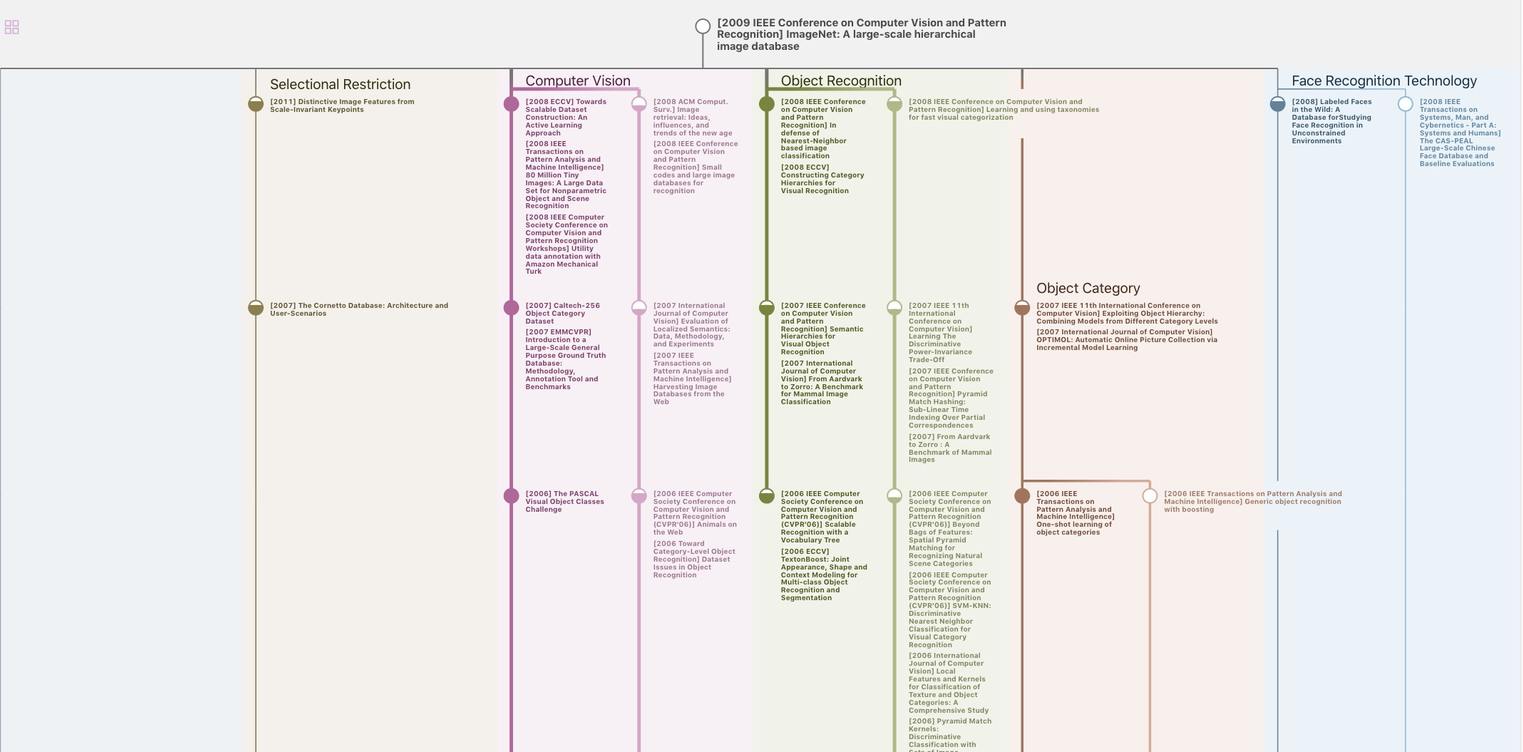
生成溯源树,研究论文发展脉络
Chat Paper
正在生成论文摘要