Adapting Pretrained Language Models for Solving Tabular Prediction Problems in the Electronic Health Record
CoRR(2023)
摘要
We propose an approach for adapting the DeBERTa model for electronic health record (EHR) tasks using domain adaptation. We pretrain a small DeBERTa model on a dataset consisting of MIMIC-III discharge summaries, clinical notes, radiology reports, and PubMed abstracts. We compare this model's performance with a DeBERTa model pre-trained on clinical texts from our institutional EHR (MeDeBERTa) and an XGBoost model. We evaluate performance on three benchmark tasks for emergency department outcomes using the MIMIC-IV-ED dataset. We preprocess the data to convert it into text format and generate four versions of the original datasets to compare data processing and data inclusion. The results show that our proposed approach outperforms the alternative models on two of three tasks (p<0.001) and matches performance on the third task, with the use of descriptive columns improving performance over the original column names.
更多查看译文
关键词
tabular prediction
AI 理解论文
溯源树
样例
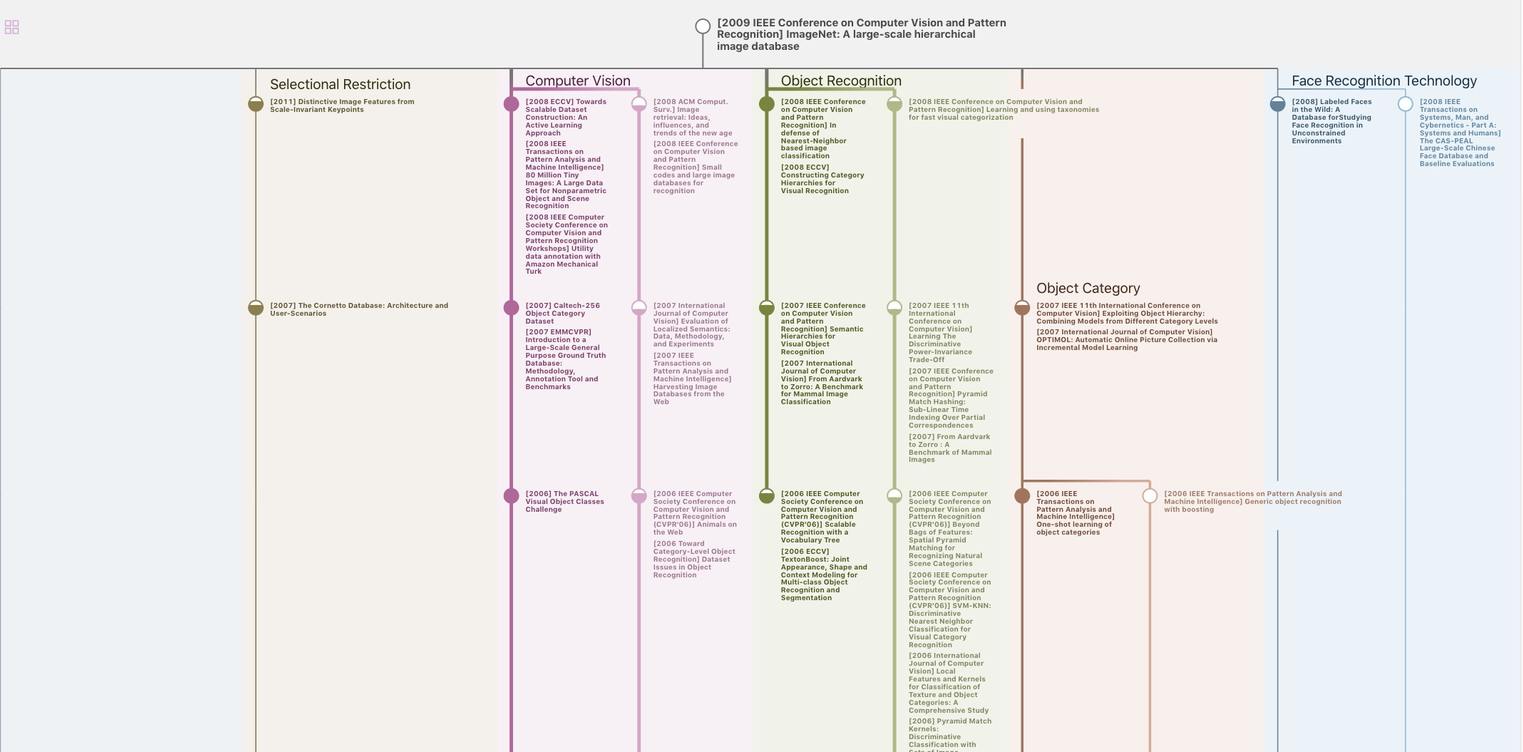
生成溯源树,研究论文发展脉络
Chat Paper
正在生成论文摘要